SpringAI实战链接
概述
想要使用SpringAI搭建知识库,就要使用SpringAI中的TikaDocumentReader,它属于ETL(提取、转换、加载)框架中的提取(Extract)阶段。
作用
TikaDocumentReader是Spring AI提供的一个文档读取器,它基于Apache Tika技术实现,能够读取并解析多种格式的文档,包括但不限于PDF、DOC/DOCX、PPT/PPTX和HTML等。这使得TikaDocumentReader成为一个非常灵活和强大的工具,适用于构建知识库或处理各种文档数据。
使用场景
TikaDocumentReader的使用场景非常广泛,包括但不限于:
构建知识库: 在构建知识库时,需要从各种格式的文档中提取文本内容。TikaDocumentReader能够轻松地读取这些文档,并将其转换为统一的格式,以便后续的处理和存储。
文档处理: 在处理大量文档时,如文档分类、摘要生成等任务中,TikaDocumentReader可以作为一个预处理步骤,将文档内容提取出来,为后续的处理提供便利。
**数据清洗:**在数据清洗过程中,有时需要从非结构化的文档中提取关键信息。TikaDocumentReader能够读取这些文档,并将其转换为结构化的数据格式,以便进行后续的数据清洗和分析。
准备工作一
在制作本地知识库的时候,还需要安装矢量数据库并下载插件vector,下载矢量化模型
矢量数据库(PostgreSQL)下载地址:EDB: Open-Source, Enterprise Postgres Database Management
插件vector下载地址:vector: Open-source vector similarity search for Postgres / PostgreSQL Extension Network
矢量化模型下载地址:text2vec-base-chinese · 模型库
遇到的问题
问题一
安装完成PostgreSQL之后,想用自带的管理器(pgAdmin4),但是报错,解决了半天没成功,直接改用navicat进行连接,但是连接的时报错(datlastsysoid does not exist),是因为Postgres 15 从pg_database表中删除了 datlastsysoid 字段引发此错误。(我安装的是PostgreSQL17)
解决方式
方法一:升级navicat
方法二:安装Postgres 15以下
方法三:修改navicat的dll文件
详述方法三:找到navicat安装的位置
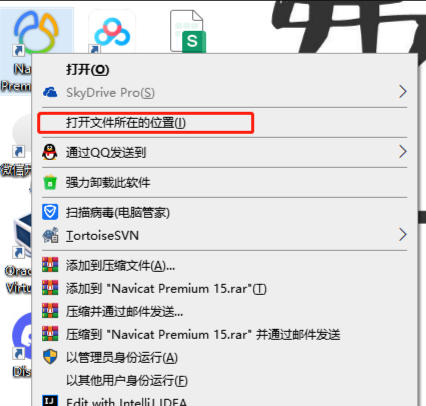
找到libcc.dll文件(最好进行备份)
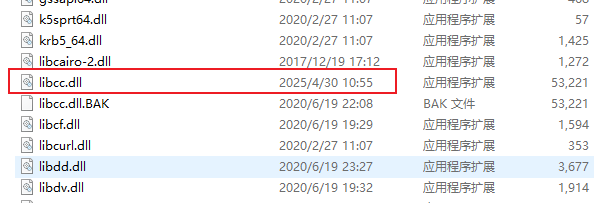
使用在线十六进制编辑器打开文件,在线地址:HexEd.it --- 基于浏览器的十六进制编辑器
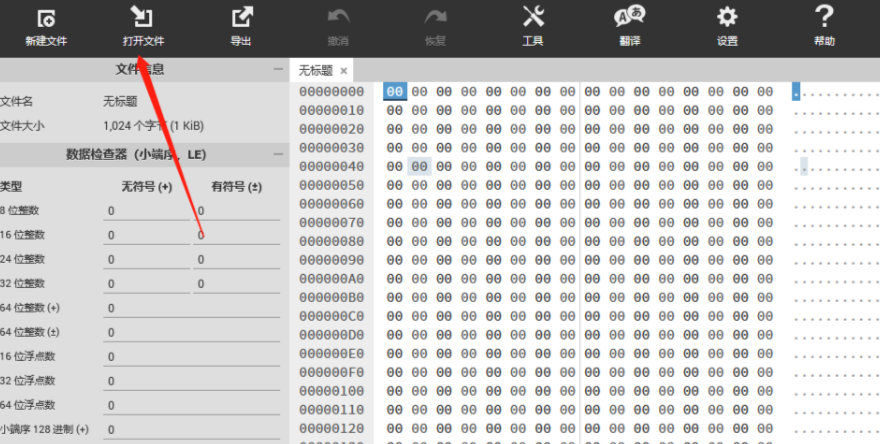
在文件中搜索"SELECT DISTINCT datlastsysoid",并将其替换为"SELECT DISTINCT dattablespace"
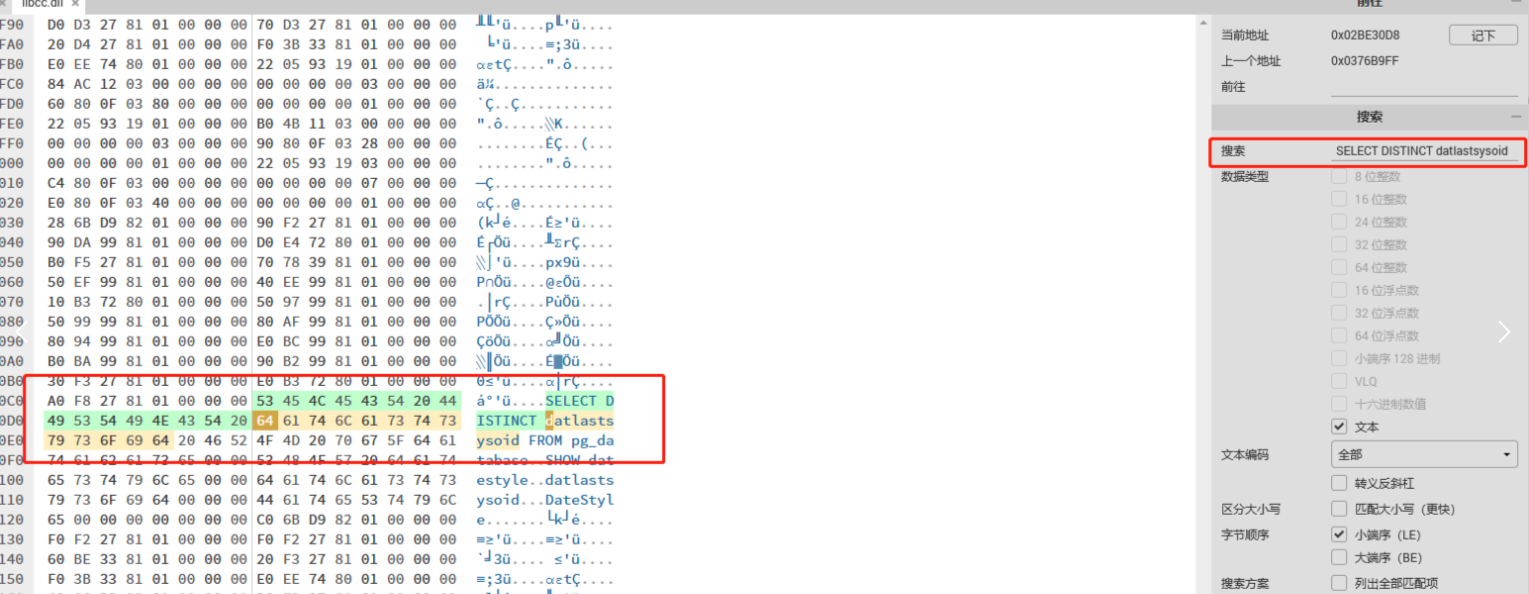
最后另存并替换原来的文件,重启navicat就可以使用了
问题二
安装vector插件的时候遇到的问题,网上也有很多方法,自己百度吧,(真的是一步一个坑)我参考的是:Windows 安装 PostgreSQL 并安装 vector 扩展_win10上安装postgresql的 vector扩展-CSDN博客
问题三
下载矢量化模型
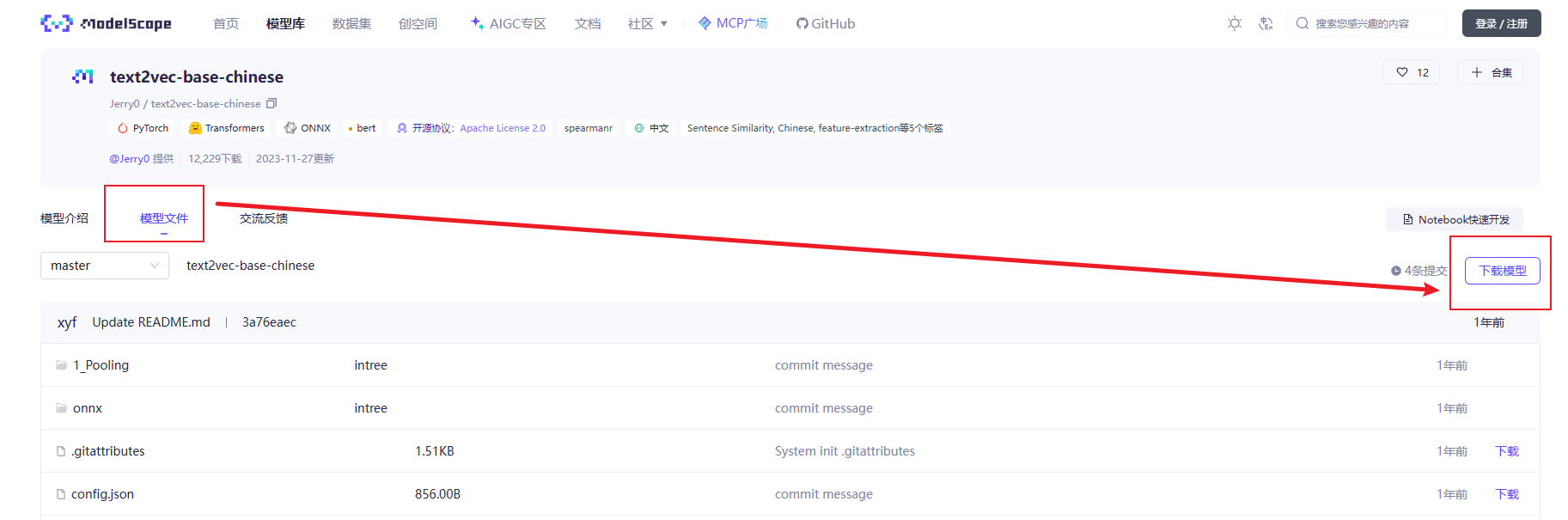
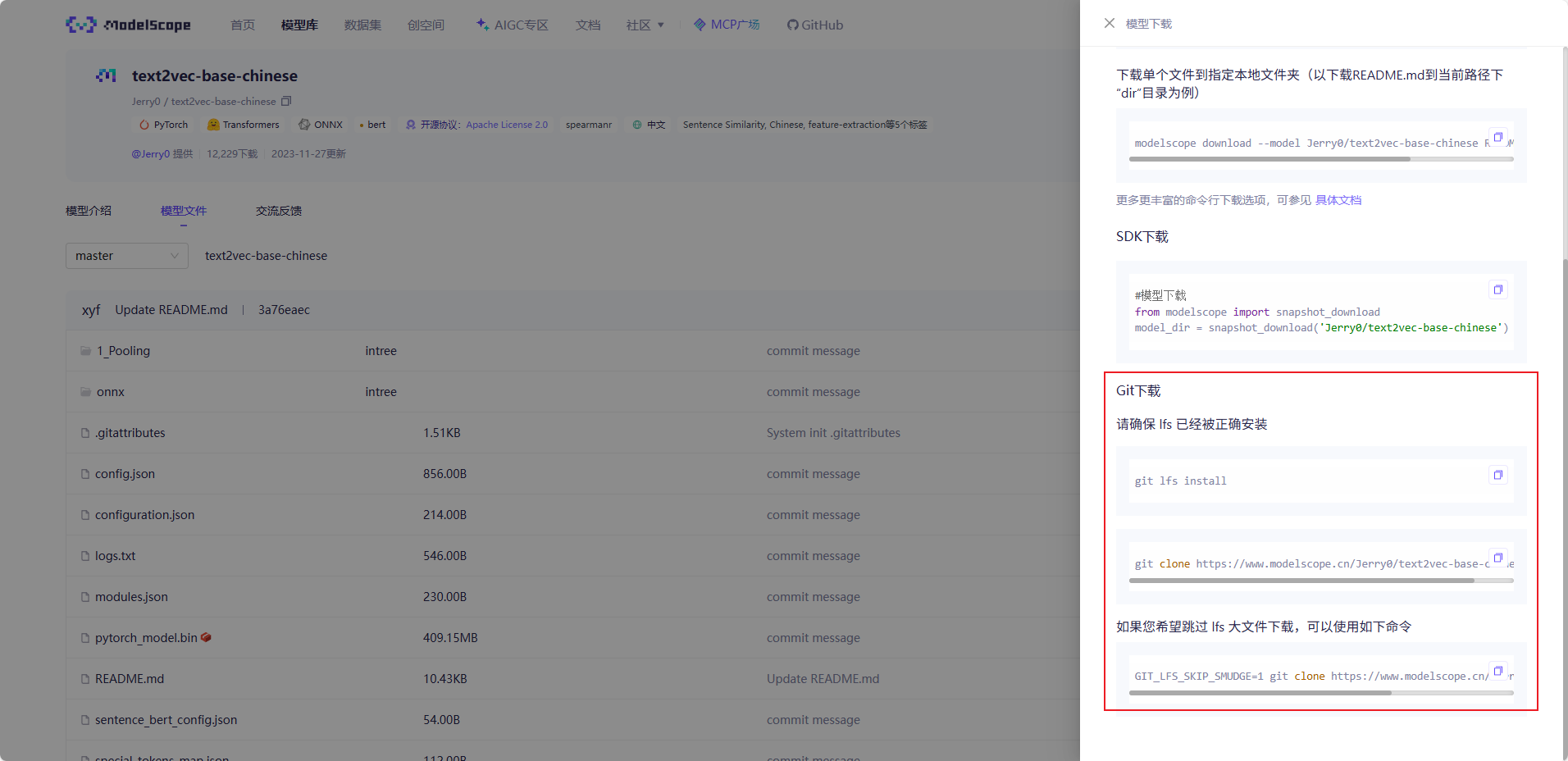
准备工作二
PostgreSQL和插件vector都安装好之后,创建矢量表
sql
CREATE EXTENSION IF NOT EXISTS vector;
CREATE EXTENSION IF NOT EXISTS hstore;
CREATE EXTENSION IF NOT EXISTS "uuid-ossp";
CREATE TABLE IF NOT EXISTS vector_store (
id uuid DEFAULT uuid_generate_v4() PRIMARY KEY,
content text,
metadata json,
embedding vector(768)
);
CREATE INDEX ON vector_store USING HNSW (embedding vector_cosine_ops);
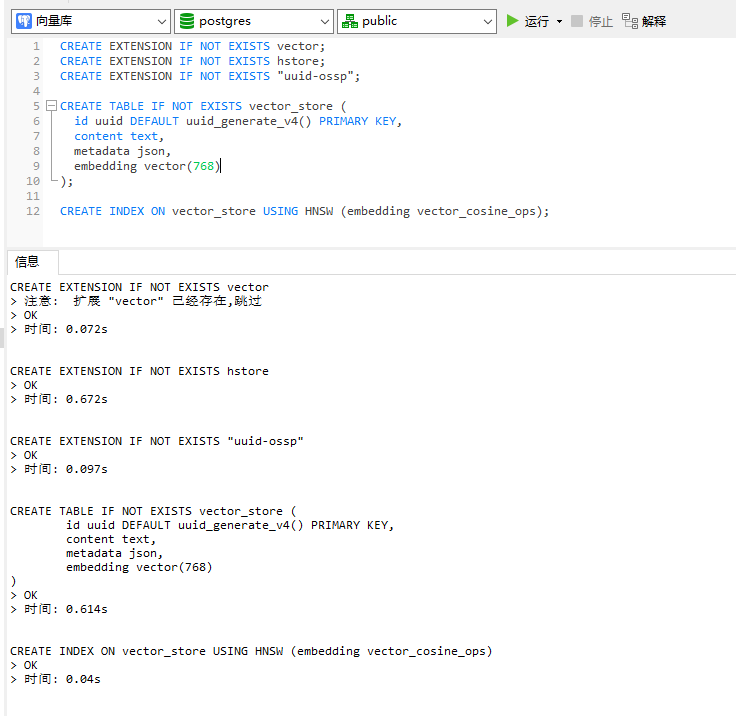
搭建工程
通过第一篇帖子,已经可以创建一个SpringAI的demo了,现在需要将矢量化模型放在resources下
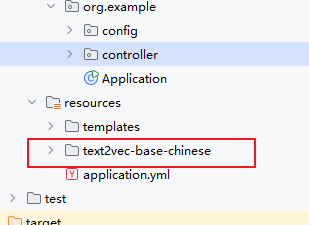
修改pom文件
java
<?xml version="1.0" encoding="UTF-8"?>
<project xmlns="http://maven.apache.org/POM/4.0.0"
xmlns:xsi="http://www.w3.org/2001/XMLSchema-instance"
xsi:schemaLocation="http://maven.apache.org/POM/4.0.0 http://maven.apache.org/xsd/maven-4.0.0.xsd">
<modelVersion>4.0.0</modelVersion>
<groupId>org.example</groupId>
<artifactId>SpringAI_Demo</artifactId>
<version>1.0-SNAPSHOT</version>
<parent>
<groupId>org.springframework.boot</groupId>
<artifactId>spring-boot-starter-parent</artifactId>
<version>3.2.5</version>
<relativePath/> <!-- lookup parent from repository -->
</parent>
<properties>
<java.version>17</java.version>
<spring-ai.version>1.0.0-M6</spring-ai.version>
<maven.compiler.source>17</maven.compiler.source>
<maven.compiler.target>17</maven.compiler.target>
<project.build.sourceEncoding>UTF-8</project.build.sourceEncoding>
</properties>
<dependencyManagement>
<dependencies>
<dependency>
<groupId>org.springframework.ai</groupId>
<artifactId>spring-ai-bom</artifactId>
<version>${spring-ai.version}</version>
<type>pom</type>
<scope>import</scope>
</dependency>
</dependencies>
</dependencyManagement>
<dependencies>
<!-- 常规jar-->
<dependency>
<groupId>org.springframework.boot</groupId>
<artifactId>spring-boot-starter-web</artifactId>
</dependency>
<dependency>
<groupId>org.springframework.boot</groupId>
<artifactId>spring-boot-starter-test</artifactId>
<scope>test</scope>
</dependency>
<dependency>
<groupId>org.springframework.boot</groupId>
<artifactId>spring-boot-starter-actuator</artifactId>
</dependency>
<!-- springAI-->
<dependency>
<groupId>org.springframework.ai</groupId>
<artifactId>spring-ai-openai-spring-boot-starter</artifactId>
</dependency>
<!-- 向量存储引擎-->
<dependency>
<groupId>org.springframework.ai</groupId>
<artifactId>spring-ai-pgvector-store-spring-boot-starter</artifactId>
</dependency>
<dependency>
<groupId>org.springframework.ai</groupId>
<artifactId>spring-ai-transformers-spring-boot-starter</artifactId>
</dependency>
<!-- 向量库-->
<dependency>
<groupId>org.postgresql</groupId>
<artifactId>postgresql</artifactId>
</dependency>
<!-- 文档解析器-->
<dependency>
<groupId>org.springframework.ai</groupId>
<artifactId>spring-ai-tika-document-reader</artifactId>
</dependency>
<!-- lombok-->
<dependency>
<groupId>org.projectlombok</groupId>
<artifactId>lombok</artifactId>
<optional>true</optional>
</dependency>
</dependencies>
<build>
<resources>
<resource>
<directory>src/main/java</directory>
<!--所在的目录-->
<includes>
<!--包括目录下的.properties,.xml 文件都会被扫描到-->
<include>**/*.properties</include>
<include>**/*.xml</include>
</includes>
<filtering>false</filtering>
</resource>
<resource>
<directory>src/main/resources</directory>
<includes>
<include>**/*.*</include>
</includes>
</resource>
</resources>
<plugins>
<plugin>
<groupId>org.springframework.boot</groupId>
<artifactId>spring-boot-maven-plugin</artifactId>
<version>3.2.5</version>
</plugin>
</plugins>
</build>
<repositories>
<repository>
<id>spring-milestones</id>
<name>Spring Milestones</name>
<url>https://repo.spring.io/milestone</url>
<snapshots>
<enabled>false</enabled>
</snapshots>
</repository>
</repositories>
</project>
与第一篇相比,多了如下依赖
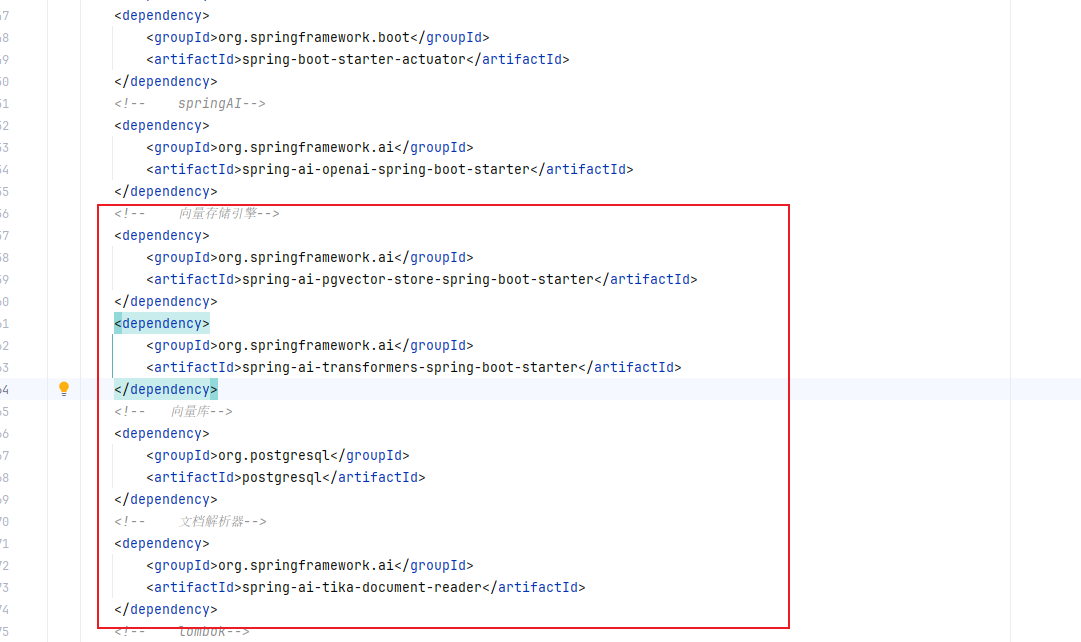
每个依赖的作用也已经在文件中加了注释
修改application.yml
java
server:
port: 3210
spring:
#向量库
datasource:
url: jdbc:postgresql://localhost:5432/postgres
username: postgres
password: #数据库密码
driver-class-name: org.postgresql.Driver
ai:
#调用ai大模型(可使用本地化部署模型,也可以使用线上的)
openai:
base-url: https://api.siliconflow.cn
api-key: #你自己申请的key
chat:
options:
model: deepseek-ai/DeepSeek-R1-Distill-Qwen-7B
#调用矢量化模型
embedding:
transformer:
onnx:
modelUri: classpath:/text2vec-base-chinese/onnx/model.onnx
tokenizer:
uri: classpath:/text2vec-base-chinese/onnx/tokenizer.json
#矢量化配置
vectorstore:
pgvector:
index-type: HNSW
distance-type: COSINE_DISTANCE
dimensions: 768
EmbeddingController(矢量化接口)
java
import org.springframework.ai.document.Document;
import org.springframework.ai.reader.tika.TikaDocumentReader;
import org.springframework.ai.transformer.splitter.TokenTextSplitter;
import org.springframework.ai.vectorstore.SearchRequest;
import org.springframework.ai.vectorstore.VectorStore;
import org.springframework.beans.factory.annotation.Autowired;
import org.springframework.core.io.InputStreamResource;
import org.springframework.web.bind.annotation.GetMapping;
import org.springframework.web.bind.annotation.PostMapping;
import org.springframework.web.bind.annotation.RequestParam;
import org.springframework.web.bind.annotation.RestController;
import org.springframework.web.multipart.MultipartFile;
import java.util.List;
import static java.util.stream.Collectors.toList;
/**
* @Author majinzhong
* @Date 2025/4/30 14:00
* @Version 1.0
*/
@RestController
public class EmbeddingController {
@Autowired
VectorStore vectorStore;
@PostMapping("/ai/vectorStore")
public List<String> vectorStore(@RequestParam(name = "file") MultipartFile file) throws Exception {
// 从IO流中读取文件
TikaDocumentReader tikaDocumentReader = new TikaDocumentReader(new InputStreamResource(file.getInputStream()));
// 将文本内容划分成更小的块
List<Document> splitDocuments = new TokenTextSplitter()
.apply(tikaDocumentReader.read());
// 存入向量数据库,这个过程会自动调用embeddingModel,将文本变成向量再存入。
vectorStore.add(splitDocuments);
return splitDocuments.stream().map(Document::getText).collect(toList());
}
@GetMapping("/ai/vectorSearch")
public List<String> vectorSearch(@RequestParam(name = "text") String text) {
List<Document> documents = vectorStore.similaritySearch(SearchRequest.builder().query(text).topK(1).build());
return documents.stream().map(Document::getText).collect(toList());
}
}
修改SimpleAiController(AI接口)
java
import org.springframework.ai.chat.client.ChatClient;
import org.springframework.ai.chat.client.advisor.MessageChatMemoryAdvisor;
import org.springframework.ai.chat.client.advisor.SimpleLoggerAdvisor;
import org.springframework.ai.chat.memory.InMemoryChatMemory;
import org.springframework.ai.document.Document;
import org.springframework.ai.vectorstore.SearchRequest;
import org.springframework.ai.vectorstore.VectorStore;
import org.springframework.beans.factory.annotation.Autowired;
import org.springframework.http.MediaType;
import org.springframework.http.codec.ServerSentEvent;
import org.springframework.web.bind.annotation.*;
import reactor.core.publisher.Flux;
import java.util.List;
import java.util.Map;
/**
* @Author majinzhong
* @Date 2025/4/28 10:37
* @Version 1.0
* SpringAI对话样例
*/
@CrossOrigin
@RestController
public class SimpleAiController {
@Autowired
VectorStore vectorStore;
// 负责处理OpenAI的bean,所需参数来自properties文件
private final ChatClient chatClient;
//对话记忆
private final InMemoryChatMemory inMemoryChatMemory;
public SimpleAiController(ChatClient chatClient,InMemoryChatMemory inMemoryChatMemory) {
this.chatClient = chatClient;
this.inMemoryChatMemory = inMemoryChatMemory;
}
/**
* 根据消息直接输出回答
* @param map
* @return
*/
@PostMapping("/ai/call")
public String call(@RequestBody Map<String,String> map) {
String message = map.get("message");
return chatClient.prompt().user(message).call().content().trim();
}
/**
* 根据消息采用流式输出
* @param message
* @return
*/
@PostMapping(value = "/ai/stream", produces = MediaType.TEXT_EVENT_STREAM_VALUE)
public Flux<ServerSentEvent<String>> streamChat(@RequestParam(value = "message", defaultValue = "Hello!") String message) {
return chatClient.prompt(message)
.stream().content().map(content -> ServerSentEvent.builder(content).event("message").build())
//问题回答结速标识,以便前端消息展示处理
.concatWithValues(ServerSentEvent.builder("").build())
.onErrorResume(e -> Flux.just(ServerSentEvent.builder("Error: " + e.getMessage()).event("error").build()));
}
/**
* 对话记忆(多轮对话)
* @param message
* @return
* @throws InterruptedException
*/
@GetMapping(value = "/ai/streamresp", produces = MediaType.TEXT_EVENT_STREAM_VALUE)
public Flux<ServerSentEvent<String>> streamResp(@RequestParam(value = "message", defaultValue = "Hello!") String message){
Flux<ServerSentEvent<String>> serverSentEventFlux = chatClient.prompt(message)
.advisors(new MessageChatMemoryAdvisor(inMemoryChatMemory, "123", 10), new SimpleLoggerAdvisor())
.stream().content().map(content -> ServerSentEvent.builder(content).event("message").build())
//问题回答结速标识,以便前端消息展示处理
.concatWithValues(ServerSentEvent.builder("").build())
.onErrorResume(e -> Flux.just(ServerSentEvent.builder("Error: " + e.getMessage()).event("error").build()));
return serverSentEventFlux;
}
/**
* 整合知识库和自己提问的问题一块向AI提问
* @param message
* @return
*/
@GetMapping("/ai/vectorStoreChat")
public Flux<String> ollamaApi(@RequestParam(value = "message") String message) {
//从知识库检索相关信息,再将检索得到的信息同用户的输入一起构建一个prompt,最后调用ollama api
List<Document> documents = vectorStore.similaritySearch(SearchRequest.builder().query(message).topK(1).build());
String targetMessage = String.format("已知信息:%s\n 用户提问:%s\n", documents.get(0).getText(), message);
return chatClient.prompt(targetMessage).stream().content();
}
}
与第一篇相比,多了如下代码
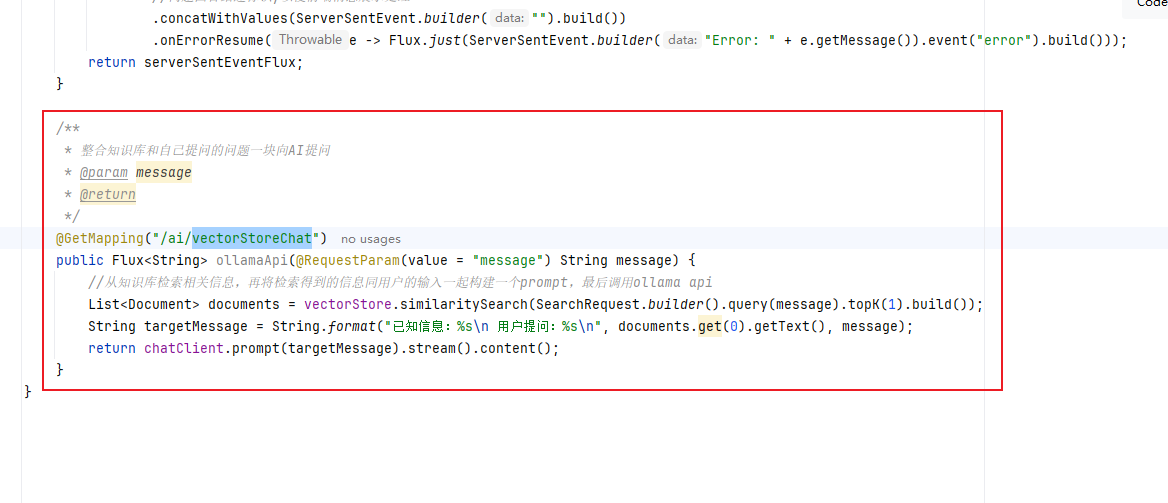
测试
首先测试上传文件到矢量库
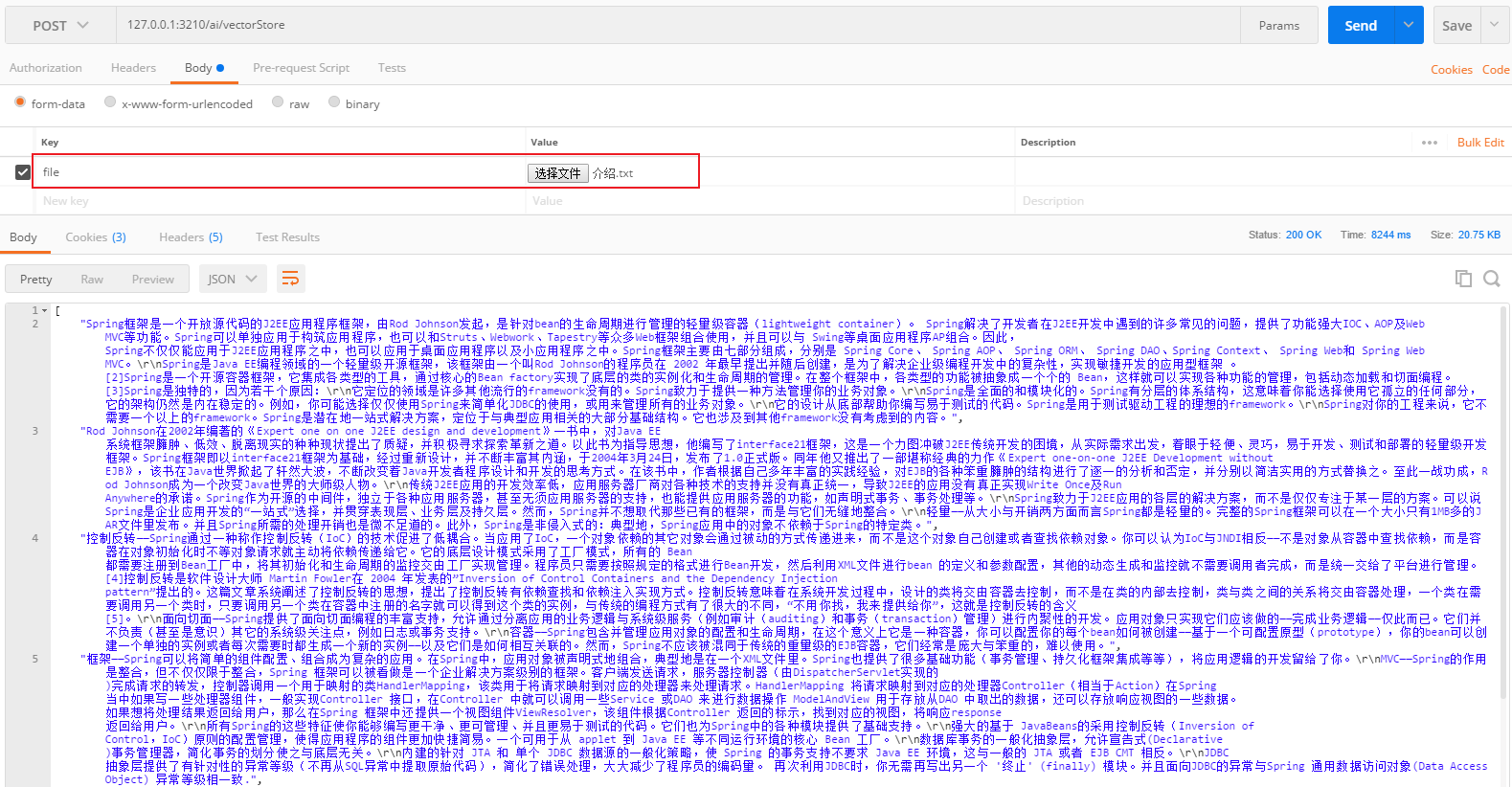
上传成功之后,查看数据库已经有数据了
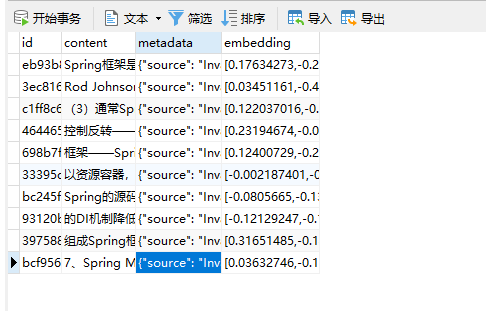
然后测试查询矢量库
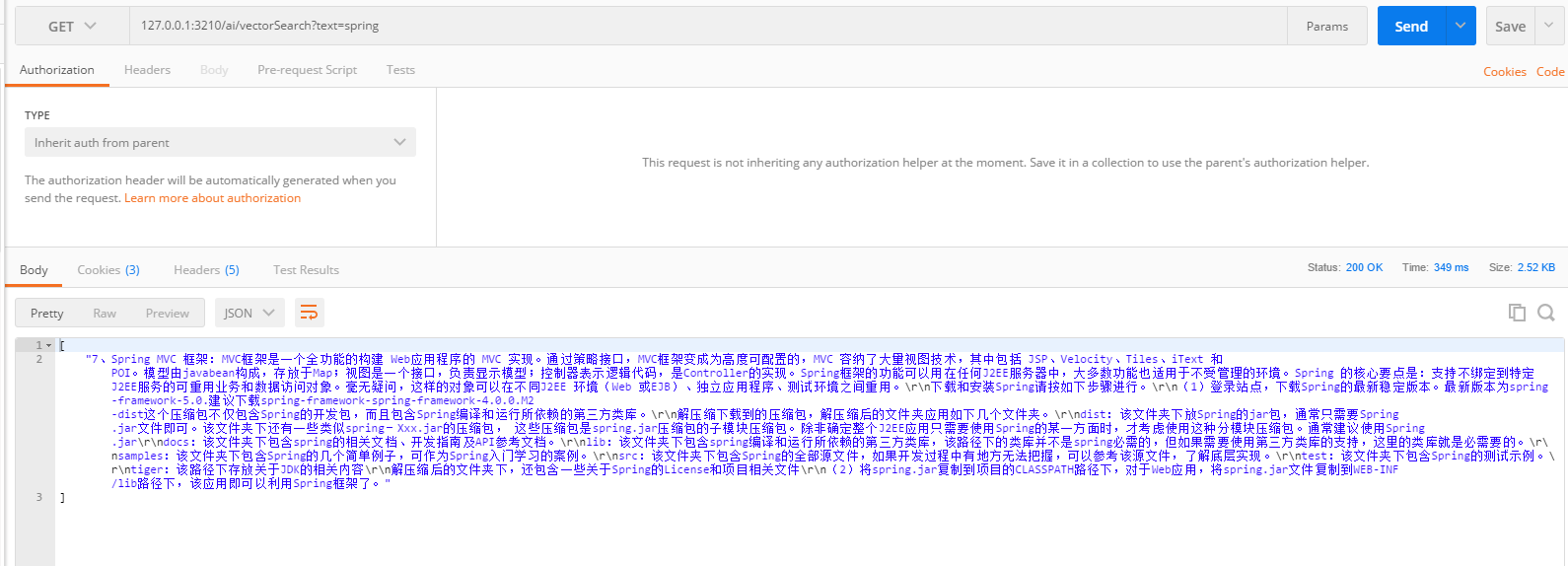
最后测试矢量库和AI统一的接口
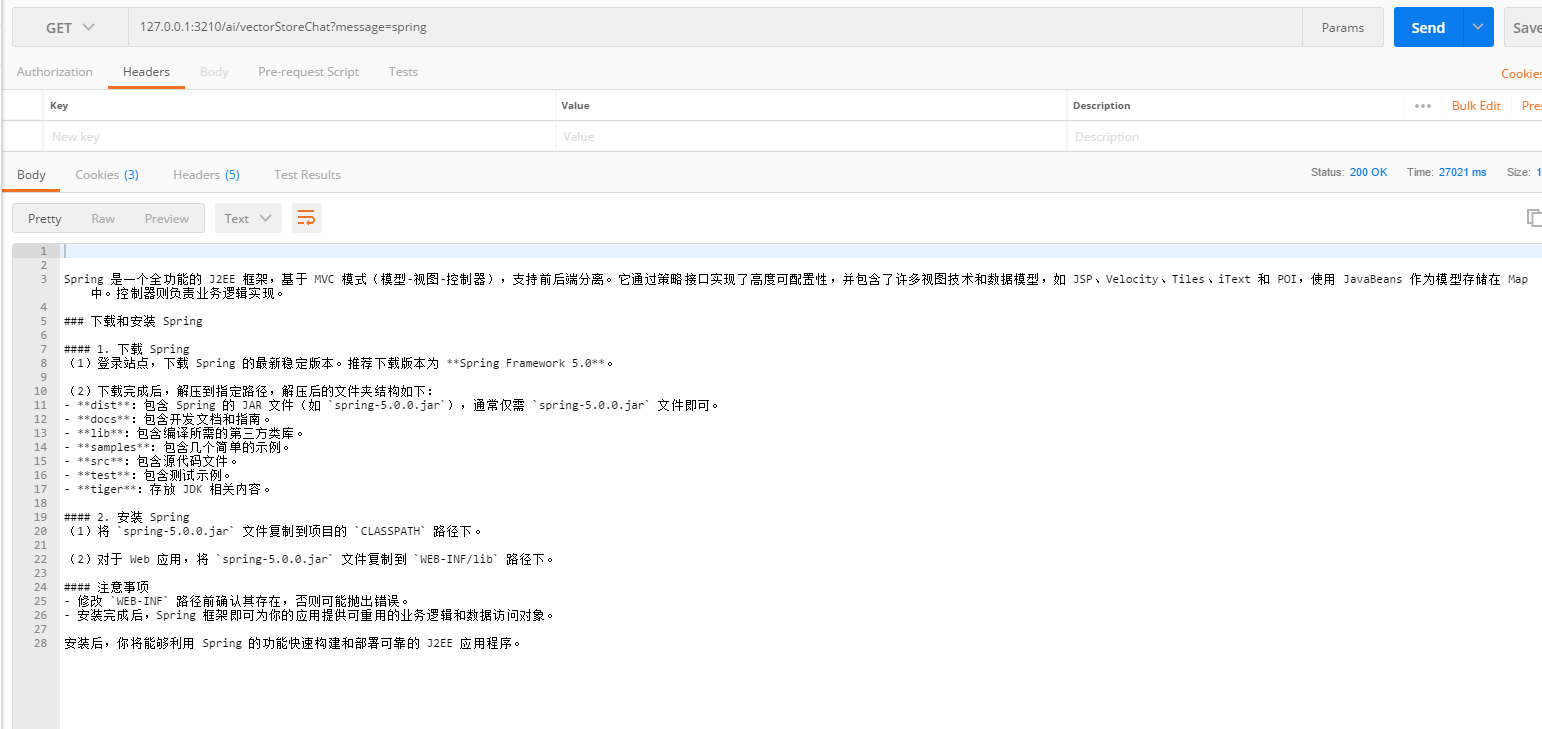
再来看一下如果只通过AI进行查询会返回什么(没有矢量库)
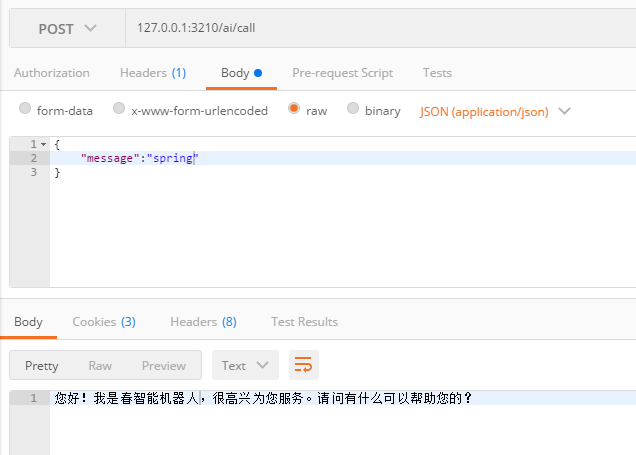
如此看来,本地化知识库成功,接下来就剩往里面一直添数据了。