引言
生成对抗网络(Generative Adversarial Networks, GANs)由Ian Goodfellow等人在2014年提出,通过生成器和判别器两个神经网络的对抗训练,成功实现了高质量数据的生成。GANs在图像生成、数据增强、风格迁移等领域取得了显著成果,成为深度学习的重要分支。本文将深入探讨GANs的基本原理、核心算法及其在实际中的应用,并提供代码示例以帮助读者更好地理解和掌握这一技术。
第一章 GANs的基本概念
1.1 什么是生成对抗网络
生成对抗网络由两个相互对抗的神经网络组成:生成器(Generator)和判别器(Discriminator)。生成器负责生成与真实数据相似的假数据,判别器负责区分真实数据和生成数据。生成器和判别器通过对抗训练,最终生成器能够生成逼真的数据,判别器难以区分其真伪。
1.2 GANs的基本结构
- 生成器(Generator):接受随机噪声作为输入,生成与真实数据分布相似的样本。
- 判别器(Discriminator):接受真实数据和生成数据作为输入,输出区分它们的概率。
GANs的目标是通过对抗训练,使得生成器生成的数据与真实数据无法区分,从而实现高质量的数据生成。
1.3 GANs的训练过程
GANs的训练过程可以概括为以下步骤:
- 初始化:随机初始化生成器和判别器的参数。
- 判别器训练:固定生成器的参数,更新判别器的参数,使其能够更好地区分真实数据和生成数据。
- 生成器训练:固定判别器的参数,更新生成器的参数,使其生成的数据能够欺骗判别器。
- 迭代:重复步骤2和3,直到生成器生成的数据与真实数据难以区分。
第二章 GANs的核心算法
2.1 标准GANs
标准GANs的损失函数由生成器和判别器的对抗损失组成。判别器的目标是最大化正确分类的概率,生成器的目标是最小化生成数据被判别器识别为假的概率。
python
import tensorflow as tf
from tensorflow.keras import layers
# 生成器模型
def build_generator():
model = tf.keras.Sequential()
model.add(layers.Dense(256, activation='relu', input_dim=100))
model.add(layers.BatchNormalization())
model.add(layers.LeakyReLU(alpha=0.2))
model.add(layers.Dense(512, activation='relu'))
model.add(layers.BatchNormalization())
model.add(layers.LeakyReLU(alpha=0.2))
model.add(layers.Dense(1024, activation='relu'))
model.add(layers.BatchNormalization())
model.add(layers.LeakyReLU(alpha=0.2))
model.add(layers.Dense(28 * 28 * 1, activation='tanh'))
model.add(layers.Reshape((28, 28, 1)))
return model
# 判别器模型
def build_discriminator():
model = tf.keras.Sequential()
model.add(layers.Flatten(input_shape=(28, 28, 1)))
model.add(layers.Dense(512, activation='relu'))
model.add(layers.LeakyReLU(alpha=0.2))
model.add(layers.Dense(256, activation='relu'))
model.add(layers.LeakyReLU(alpha=0.2))
model.add(layers.Dense(1, activation='sigmoid'))
return model
# 编译模型
generator = build_generator()
discriminator = build_discriminator()
discriminator.compile(optimizer='adam', loss='binary_crossentropy', metrics=['accuracy'])
# GAN模型
discriminator.trainable = False
gan_input = layers.Input(shape=(100,))
generated_image = generator(gan_input)
gan_output = discriminator(generated_image)
gan = tf.keras.models.Model(gan_input, gan_output)
gan.compile(optimizer='adam', loss='binary_crossentropy')
# 加载MNIST数据集
(x_train, _), (_, _) = tf.keras.datasets.mnist.load_data()
x_train = (x_train.astype('float32') - 127.5) / 127.5
x_train = np.expand_dims(x_train, axis=3)
# 训练GANs
batch_size = 128
epochs = 10000
half_batch = int(batch_size / 2)
for epoch in range(epochs):
# 训练判别器
idx = np.random.randint(0, x_train.shape[0], half_batch)
real_images = x_train[idx]
noise = np.random.normal(0, 1, (half_batch, 100))
generated_images = generator.predict(noise)
d_loss_real = discriminator.train_on_batch(real_images, np.ones((half_batch, 1)))
d_loss_fake = discriminator.train_on_batch(generated_images, np.zeros((half_batch, 1)))
d_loss = 0.5 * np.add(d_loss_real, d_loss_fake)
# 训练生成器
noise = np.random.normal(0, 1, (batch_size, 100))
valid_y = np.array([1] * batch_size)
g_loss = gan.train_on_batch(noise, valid_y)
if epoch % 1000 == 0:
print(f"{epoch} [D loss: {d_loss[0]} | D accuracy: {100 * d_loss[1]}] [G loss: {g_loss}]")
2.2 深度卷积生成对抗网络(DCGAN)
DCGAN通过在生成器和判别器中引入卷积层,显著提高了图像生成的质量。以下是一个基于DCGAN的示例。
python
def build_generator():
model = tf.keras.Sequential()
model.add(layers.Dense(7 * 7 * 256, use_bias=False, input_shape=(100,)))
model.add(layers.BatchNormalization())
model.add(layers.LeakyReLU())
model.add(layers.Reshape((7, 7, 256)))
model.add(layers.Conv2DTranspose(128, (5, 5), strides=(1, 1), padding='same', use_bias=False))
model.add(layers.BatchNormalization())
model.add(layers.LeakyReLU())
model.add(layers.Conv2DTranspose(64, (5, 5), strides=(2, 2), padding='same', use_bias=False))
model.add(layers.BatchNormalization())
model.add(layers.LeakyReLU())
model.add(layers.Conv2DTranspose(1, (5, 5), strides=(2, 2), padding='same', use_bias=False, activation='tanh'))
return model
def build_discriminator():
model = tf.keras.Sequential()
model.add(layers.Conv2D(64, (5, 5), strides=(2, 2), padding='same', input_shape=[28, 28, 1]))
model.add(layers.LeakyReLU())
model.add(layers.Dropout(0.3))
model.add(layers.Conv2D(128, (5, 5), strides=(2, 2), padding='same'))
model.add(layers.LeakyReLU())
model.add(layers.Dropout(0.3))
model.add(layers.Flatten())
model.add(layers.Dense(1))
return model
generator = build_generator()
discriminator = build_discriminator()
# 编译判别器
discriminator.compile(optimizer='adam', loss='binary_crossentropy', metrics=['accuracy'])
# 编译GAN模型
discriminator.trainable = False
gan_input = layers.Input(shape=(100,))
generated_image = generator(gan_input)
gan_output = discriminator(generated_image)
gan = tf.keras.models.Model(gan_input, gan_output)
gan.compile(optimizer='adam', loss='binary_crossentropy')
# 加载MNIST数据集
(x_train, _), (_, _) = tf.keras.datasets.mnist.load_data()
x_train = (x_train.astype('float32') - 127.5) / 127.5
x_train = np.expand_dims(x_train, axis=3)
# 训练DCGAN
batch_size = 128
epochs = 10000
half_batch = int(batch_size / 2)
for epoch in range(epochs):
# 训练判别器
idx = np.random.randint(0, x_train.shape[0], half_batch)
real_images = x_train[idx]
noise = np.random.normal(0, 1, (half_batch, 100))
generated_images = generator.predict(noise)
d_loss_real = discriminator.train_on_batch(real
_images, np.ones((half_batch, 1)))
d_loss_fake = discriminator.train_on_batch(generated_images, np.zeros((half_batch, 1)))
d_loss = 0.5 * np.add(d_loss_real, d_loss_fake)
# 训练生成器
noise = np.random.normal(0, 1, (batch_size, 100))
valid_y = np.array([1] * batch_size)
g_loss = gan.train_on_batch(noise, valid_y)
if epoch % 1000 == 0:
print(f"{epoch} [D loss: {d_loss[0]} | D accuracy: {100 * d_loss[1]}] [G loss: {g_loss}]")
2.3 条件生成对抗网络(Conditional GAN)
条件生成对抗网络(Conditional GAN, cGAN)通过在生成器和判别器中引入条件变量,使生成的数据能够满足特定条件。
python
def build_generator():
model = tf.keras.Sequential()
model.add(layers.Dense(7 * 7 * 256, use_bias=False, input_shape=(110,)))
model.add(layers.BatchNormalization())
model.add(layers.LeakyReLU())
model.add(layers.Reshape((7, 7, 256)))
model.add(layers.Conv2DTranspose(128, (5, 5), strides=(1, 1), padding='same', use_bias=False))
model.add(layers.BatchNormalization())
model.add(layers.LeakyReLU())
model.add(layers.Conv2DTranspose(64, (5, 5), strides=(2, 2), padding='same', use_bias=False))
model.add(layers.BatchNormalization())
model.add(layers.LeakyReLU())
model.add(layers.Conv2DTranspose(1, (5, 5), strides=(2, 2), padding='same', use_bias=False, activation='tanh'))
return model
def build_discriminator():
model = tf.keras.Sequential()
model.add(layers.Conv2D(64, (5, 5), strides=(2, 2), padding='same', input_shape=[28, 28, 11]))
model.add(layers.LeakyReLU())
model.add(layers.Dropout(0.3))
model.add(layers.Conv2D(128, (5, 5), strides=(2, 2), padding='same'))
model.add(layers.LeakyReLU())
model.add(layers.Dropout(0.3))
model.add(layers.Flatten())
model.add(layers.Dense(1))
return model
generator = build_generator()
discriminator = build_discriminator()
# 编译判别器
discriminator.compile(optimizer='adam', loss='binary_crossentropy', metrics=['accuracy'])
# 编译cGAN模型
discriminator.trainable = False
noise_input = layers.Input(shape=(100,))
label_input = layers.Input(shape=(10,))
gan_input = layers.Concatenate()([noise_input, label_input])
generated_image = generator(gan_input)
label_image = layers.Concatenate()([generated_image, label_input])
gan_output = discriminator(label_image)
cgan = tf.keras.models.Model([noise_input, label_input], gan_output)
cgan.compile(optimizer='adam', loss='binary_crossentropy')
# 加载MNIST数据集
(x_train, y_train), (_, _) = tf.keras.datasets.mnist.load_data()
x_train = (x_train.astype('float32') - 127.5) / 127.5
x_train = np.expand_dims(x_train, axis=3)
y_train = tf.keras.utils.to_categorical(y_train, 10)
# 训练cGAN
batch_size = 128
epochs = 10000
half_batch = int(batch_size / 2)
for epoch in range(epochs):
# 训练判别器
idx = np.random.randint(0, x_train.shape[0], half_batch)
real_images = x_train[idx]
real_labels = y_train[idx]
noise = np.random.normal(0, 1, (half_batch, 100))
generated_labels = np.random.randint(0, 10, half_batch)
generated_labels = tf.keras.utils.to_categorical(generated_labels, 10)
generated_images = generator.predict([noise, generated_labels])
real_images_with_labels = np.concatenate([real_images, real_labels], axis=3)
generated_images_with_labels = np.concatenate([generated_images, generated_labels], axis=3)
d_loss_real = discriminator.train_on_batch(real_images_with_labels, np.ones((half_batch, 1)))
d_loss_fake = discriminator.train_on_batch(generated_images_with_labels, np.zeros((half_batch, 1)))
d_loss = 0.5 * np.add(d_loss_real, d_loss_fake)
# 训练生成器
noise = np.random.normal(0, 1, (batch_size, 100))
valid_y = np.array([1] * batch_size)
labels = np.random.randint(0, 10, batch_size)
labels = tf.keras.utils.to_categorical(labels, 10)
g_loss = cgan.train_on_batch([noise, labels], valid_y)
if epoch % 1000 == 0:
print(f"{epoch} [D loss: {d_loss[0]} | D accuracy: {100 * d_loss[1]}] [G loss: {g_loss}]")
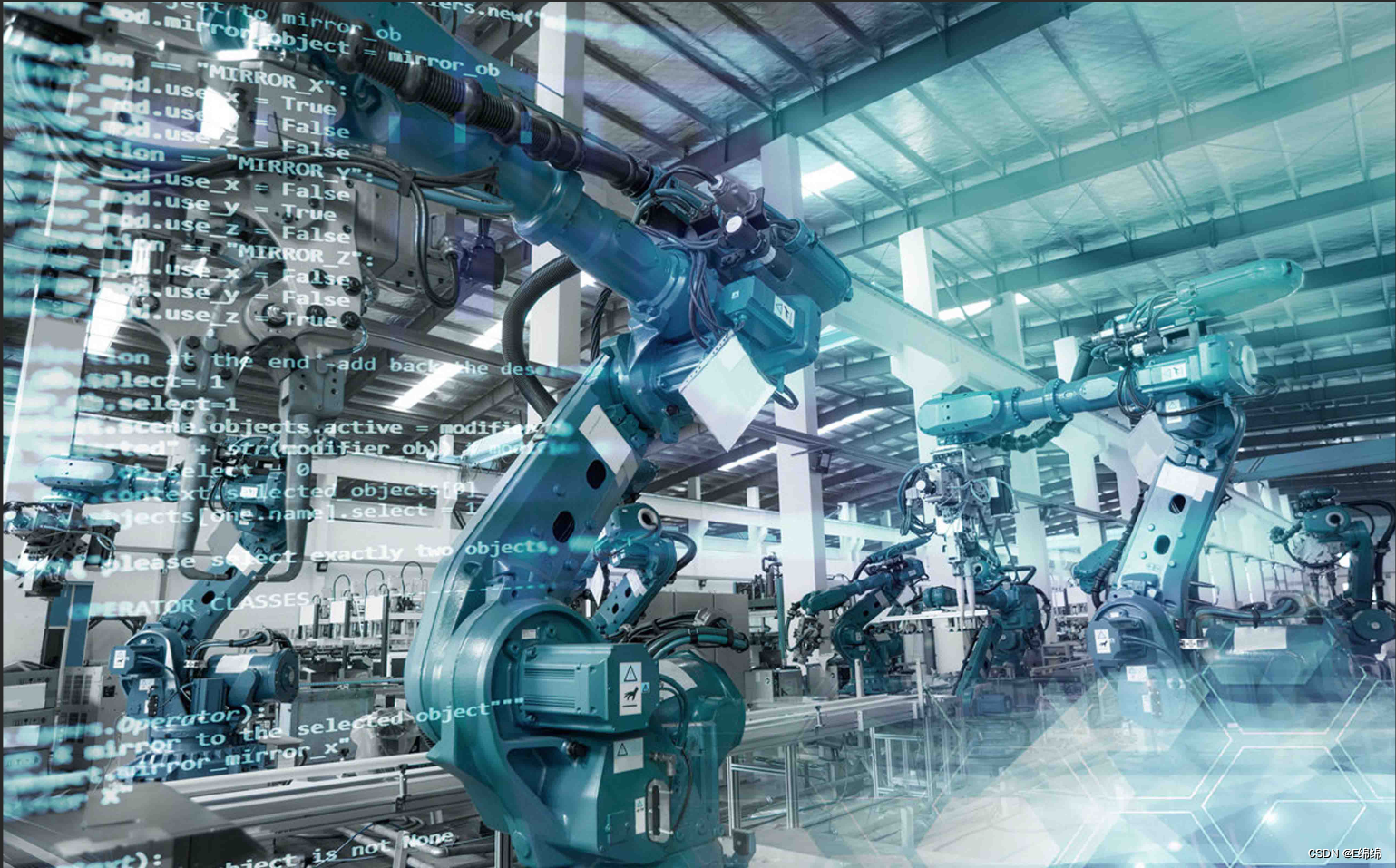
第三章 GANs的应用实例
3.1 图像生成
GANs在图像生成任务中表现出色,可以生成高质量的图像。以下是一个使用DCGAN生成手写数字图像的示例。
python
import matplotlib.pyplot as plt
# 生成手写数字图像
noise = np.random.normal(0, 1, (25, 100))
generated_images = generator.predict(noise)
# 绘制生成的图像
plt.figure(figsize=(10, 10))
for i in range(generated_images.shape[0]):
plt.subplot(5, 5, i + 1)
plt.imshow(generated_images[i, :, :, 0], cmap='gray')
plt.axis('off')
plt.tight_layout()
plt.show()
3.2 数据增强
GANs可以用于数据增强,通过生成新的样本扩展训练数据集,从而提高模型的泛化能力。以下是一个使用cGAN生成带标签的手写数字图像的示例。
python
# 生成带标签的手写数字图像
noise = np.random.normal(0, 1, (25, 100))
labels = np.array([0, 1, 2, 3, 4, 5, 6, 7, 8, 9] * 2 + [0, 1, 2, 3, 4])
labels = tf.keras.utils.to_categorical(labels, 10)
generated_images = generator.predict([noise, labels])
# 绘制生成的图像
plt.figure(figsize=(10, 10))
for i in range(generated_images.shape[0]):
plt.subplot(5, 5, i + 1)
plt.imshow(generated_images[i, :, :, 0], cmap='gray')
plt.axis('off')
plt.tight_layout()
plt.show()
3.3 风格迁移
GANs可以用于风格迁移,通过将一种图像的内容与另一种图像的风格结合,生成具有新风格的图像。以下是一个使用CycleGAN进行图像风格迁移的示例。
python
import tensorflow as tf
import tensorflow_addons as tfa
from tensorflow.keras import layers
def residual_block(x, filters, kernel_size=3):
fx = layers.Conv2D(filters, kernel_size, padding='same')(x)
fx = tfa.layers.InstanceNormalization()(fx)
fx = layers.ReLU()(fx)
fx = layers.Conv2D(filters, kernel_size, padding='same')(fx)
fx = tfa.layers.InstanceNormalization()(fx)
x = layers.Add()([x, fx])
return x
def build_generator():
inputs = layers.Input(shape=[256, 256, 3])
x = layers.Conv2D(64, 7, padding='same')(inputs)
x = tfa.layers.InstanceNormalization()(x)
x = layers.ReLU()(x)
x = layers.Conv2D(128, 3, strides=2, padding='same')(x)
x = tfa.layers.InstanceNormalization()(x)
x = layers.ReLU()(x)
x = layers.Conv2D(256, 3, strides=2, padding='same')(x)
x = tfa.layers.InstanceNormalization()(x)
x = layers.ReLU()(x)
for _ in range(9):
x = residual_block(x, 256)
x = layers.Conv2DTranspose(128, 3, strides=2, padding='same')(x)
x = tfa.layers.InstanceNormalization()(x)
x = layers.ReLU()(x)
x = layers.Conv2DTranspose(64, 3, strides=2, padding='same')(x)
x = tfa.layers.InstanceNormalization()(x)
x = layers.ReLU()(x)
x = layers.Conv2D(3, 7, padding='same')(x)
x = layers.Activation('tanh')(x)
return tf.keras.Model(inputs, x)
def build_discriminator():
inputs = layers.Input(shape=[256, 256, 3])
x = layers.Conv2D(64, 4, strides=2, padding='same')(inputs)
x = layers.LeakyReLU(alpha=0.2)(x)
x = layers.Conv2D(128, 4, strides=2, padding='same')(x)
x = tfa.layers.InstanceNormalization()(x)
x = layers.LeakyReLU(alpha=0.2)(x)
x = layers.Conv2D(256, 4, strides=2, padding='same')(x)
x = tfa.layers.InstanceNormalization()(x)
x = layers.LeakyReLU(alpha=0.2)(x)
x = layers.Conv2D(512, 4, strides=2, padding='same')(x)
x = tfa.layers.InstanceNormalization()(x)
x = layers.LeakyReLU(alpha=0.2)(x)
x = layers.Conv2D(1, 4, padding='same')(x)
return tf.keras.Model(inputs, x)
# 构建CycleGAN模型
generator_g = build_generator()
generator_f = build_generator()
discriminator_x = build_discriminator()
discriminator_y = build_discriminator()
# 编译模型
generator_g.compile(optimizer='adam', loss='mse')
generator_f.compile(optimizer='adam', loss='mse')
discriminator_x.compile(optimizer='adam', loss='mse')
discriminator_y.compile(optimizer='adam', loss='mse')
# 训练CycleGAN
# 训练数据准备和训练代码略
# 使用CycleGAN进行风格迁移
def generate_images(model, test_input):
prediction = model(test_input)
plt.figure(figsize=(12, 12))
display_list = [test_input[0], prediction[0]]
title = ['Input Image', 'Predicted Image']
for i in range(2):
plt.subplot(1, 2, i + 1)
plt.title(title[i])
plt.imshow(display_list[i] * 0.5 + 0.5)
plt.axis('off')
plt.show()
# 测试图像
test_image = tf.expand_dims(tf.image.resize(test_image, (256, 256)), axis=0) / 127.5 - 1
generate_images(generator_g, test_image)
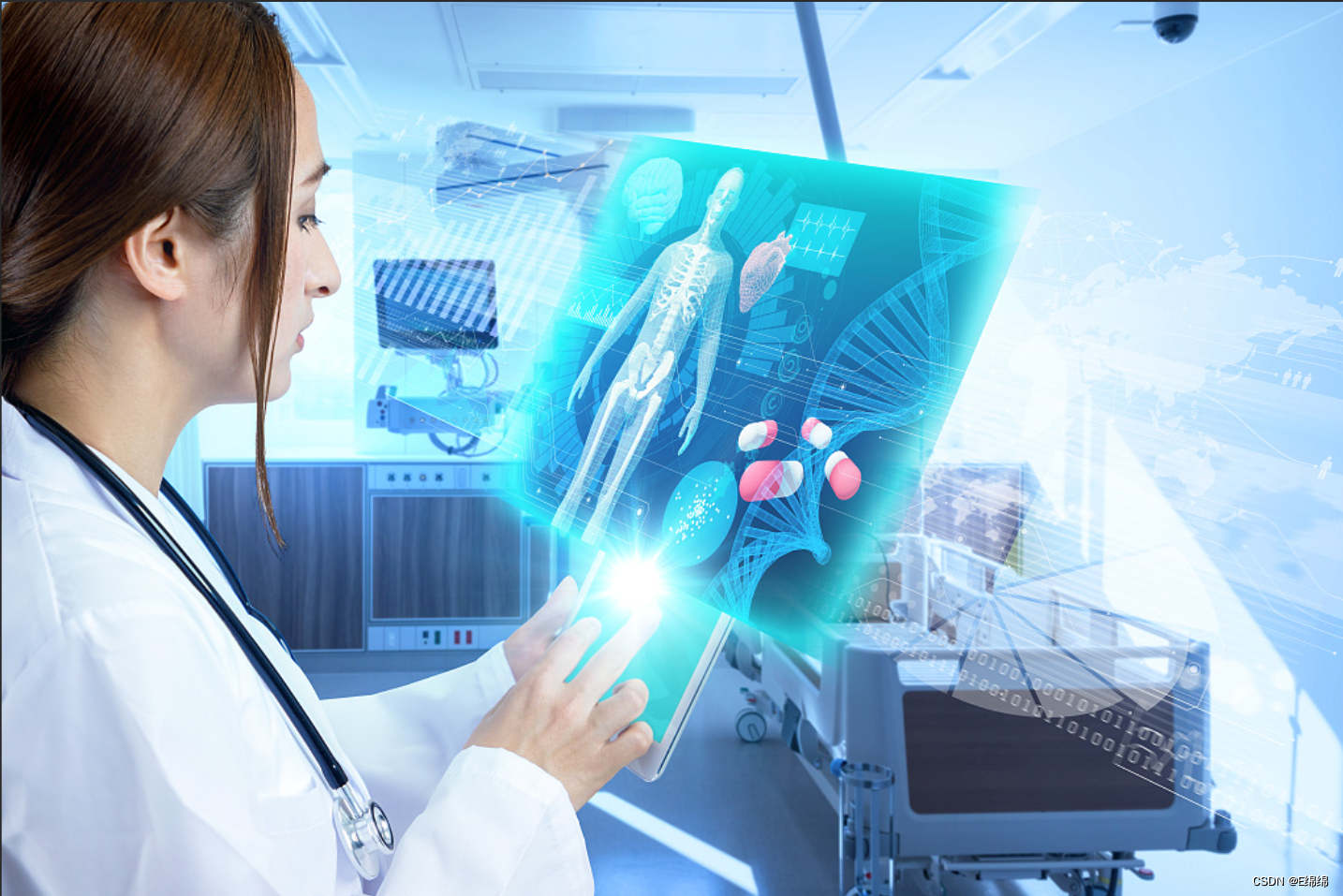
第四章 GANs的未来发展与挑战
4.1 训练稳定性
GANs的训练过程容易出现不稳定性,如模式崩溃(mode collapse)和梯度消失等问题。研究如何提高GANs训练的稳定性是一个重要的方向。
4.2 模型评价
如何有效评估GANs生成数据的质量和多样性是一个挑战。研究方向包括开发更好的评价指标,如Frechet Inception Distance(FID)和Inception Score(IS)等。
4.3 应用扩展
GANs的应用范围不断扩大,研究如何在更多领域和任务中应用GANs,如文本生成、音频生成和科学模拟等,是一个重要的方向。
结论
生成对抗网络作为一种强大的生成模型,通过生成器和判别器的对抗训练,实现了高质量的数据生成和多种应用。本文详细介绍了GANs的基本概念、核心算法及其在实际中的应用,并提供了具体的代码示例,帮助读者深入理解和掌握这一技术。希望本文能够为您进一步探索和应用生成对抗网络提供有价值的参考。
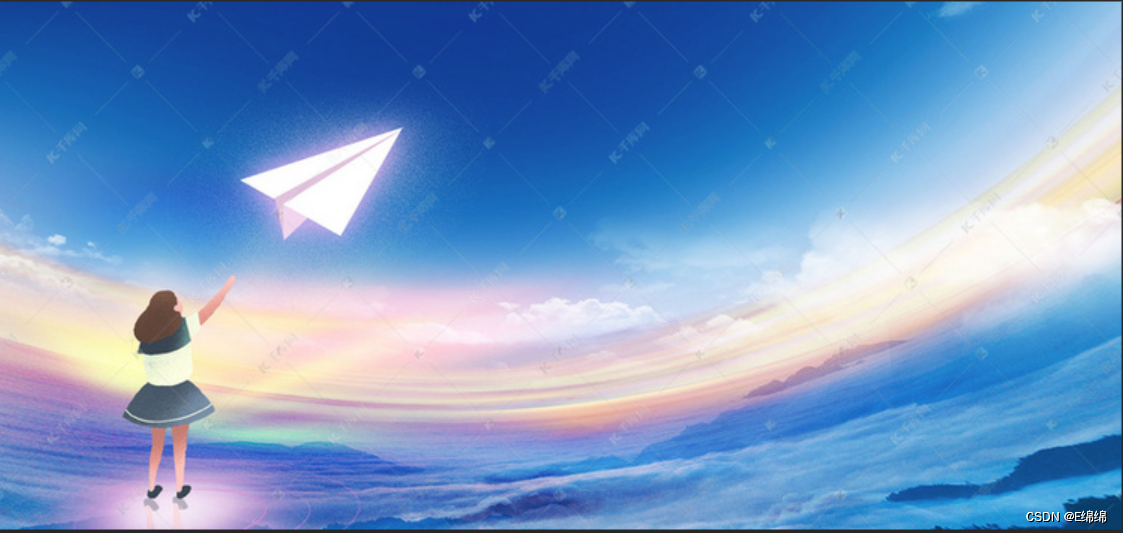