1 非线性激活
1.1 几种常见的非线性激活:
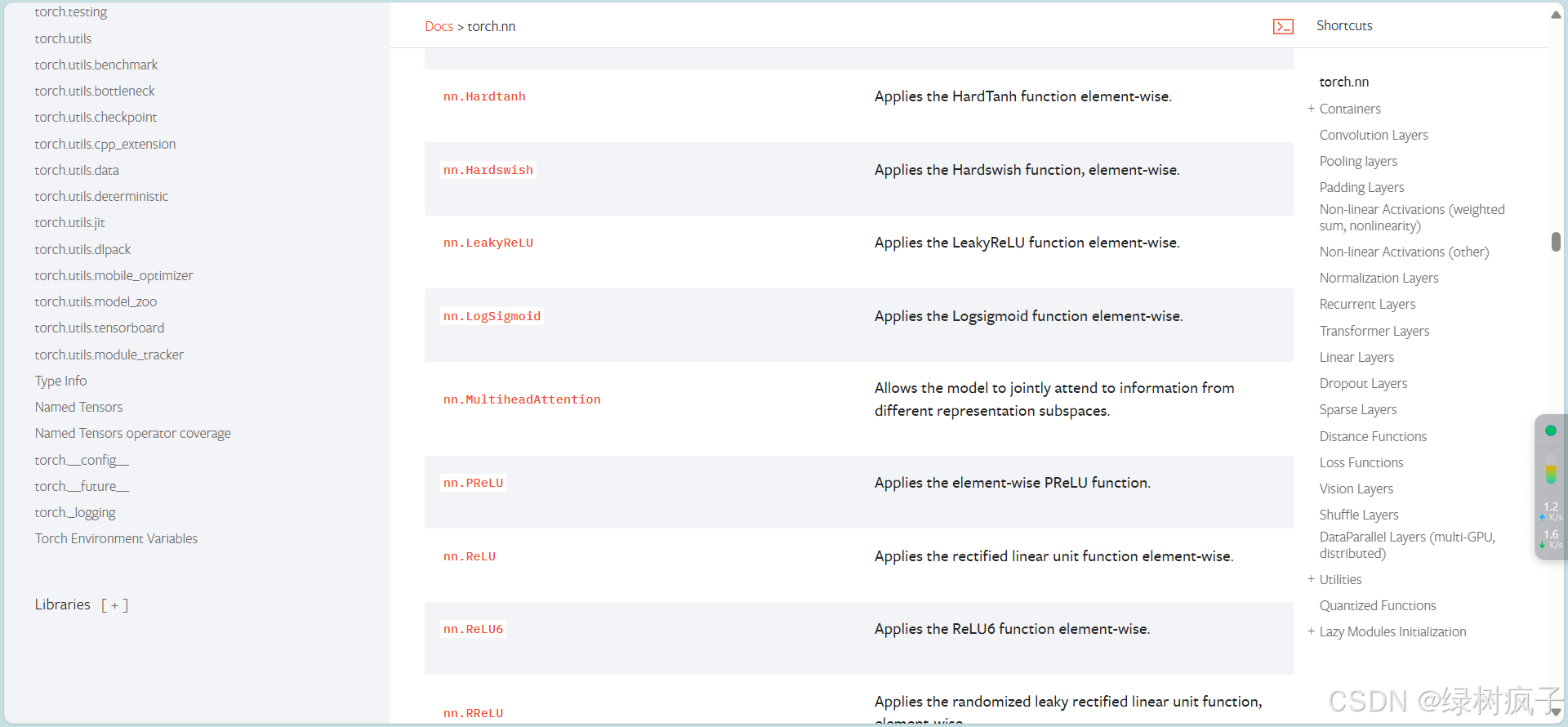
ReLU (Rectified Linear Unit)线性整流函数
Sigmoid
1.2代码实战:
1.2.1 ReLU
python
import torch
from torch import nn
from torch.nn import ReLU
input=torch.tensor([[1,-0.5],
[-1,3]])
input=torch.reshape(input,(-1,1,2,2))
print(input.shape)
class Tudui(nn.Module):
def __init__(self):
super(Tudui,self).__init__()
self.relu1 = ReLU()
def forward(self, input):
output = self.relu1(input)
return output
tudui=Tudui()
output=tudui(input)
print(output)
- inplace 参数:是否在原来位置上更新

1.2.2 Sigmoid
python
import torch
import torchvision
from torch import nn
from torch.nn import ReLU, Sigmoid
from torch.utils.data import DataLoader
from torch.utils.tensorboard import SummaryWriter
input=torch.tensor([[1,-0.5],
[-1,3]])
input=torch.reshape(input,(-1,1,2,2))
print(input.shape)
dataset = torchvision.datasets.CIFAR10("./data", train=False,
transform=torchvision.transforms.ToTensor(), download=True)
dataloader = DataLoader(dataset, batch_size=64)
class Tudui(nn.Module):
def __init__(self):
super(Tudui,self).__init__()
self.sigmoid1 = Sigmoid()
def forward(self, input):
output = self.sigmoid1(input)
return output
tudui=Tudui()
writer = SummaryWriter("logs_Non-linear")
step = 0
for data in dataloader:
imgs, targets = data
writer.add_images("input", imgs, step)
output = tudui(imgs)
writer.add_images("output",output, step)
step = step + 1
writer.close()
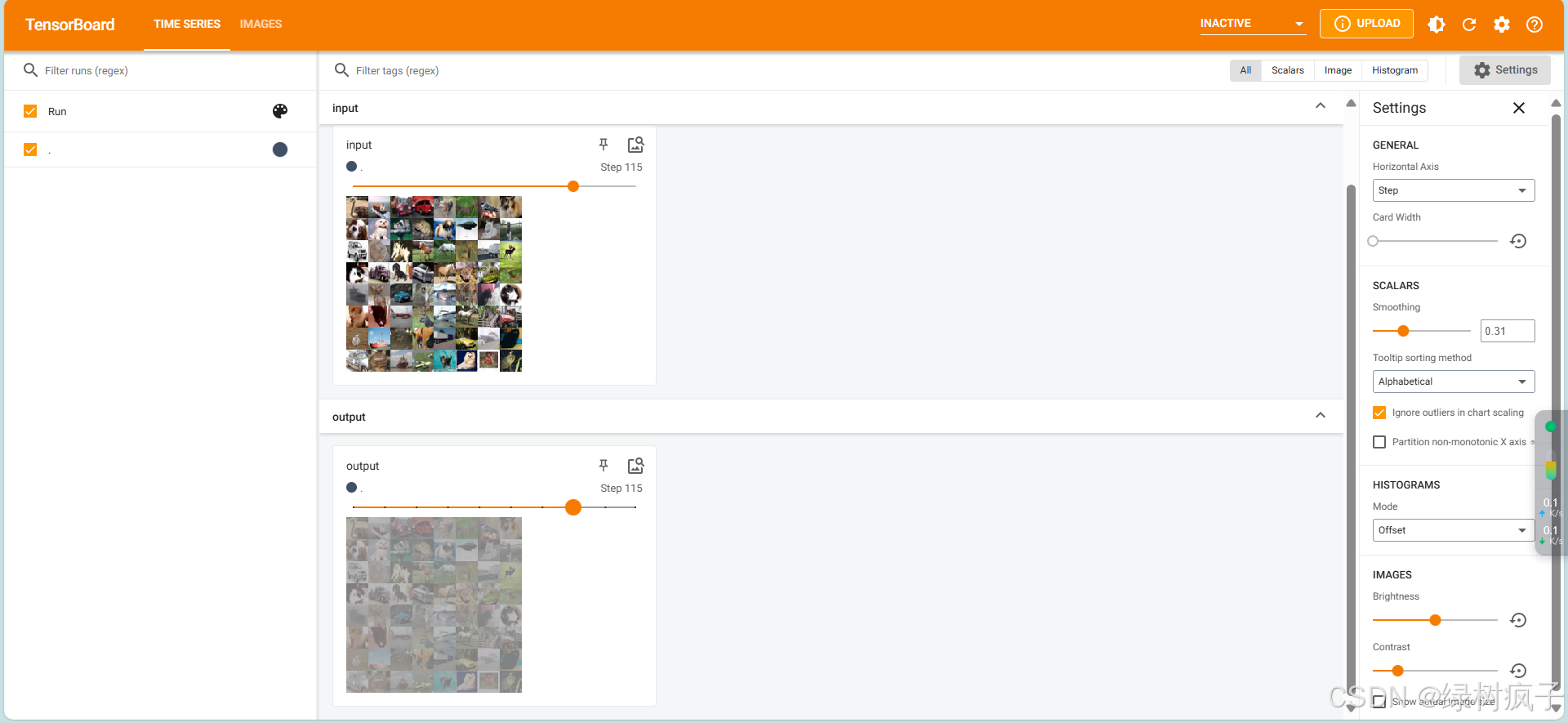
非线性变化的主要目的在于给网络引入非线性的特征。非线性特征越多,越能训练出符合各种曲线或特征的模型,从而提高模型的泛化能力。
2 线性层及其他层介绍:
2.1简要介绍nn模块里的各种层:
-
Normalization Layers正则化层
正则化可以加快神经网络的训练速度,用的比较少,不作介绍,自己看文档
-
Recurrent Layers:
一般用于文字识别,自己看文档。
-
Transformer Layers:
-
Linear Layers:
-
Dropout Layers:
在训练过程中,随机将输入张量的部分元素清零。主要作用是防止过拟合。
-
Saprse Layers:
用于自然语言处理。
-
Distance Functions:
计算两个值之间的距离
-
Loss Functions:
计算误差
2.2 Linear Layers讲解:
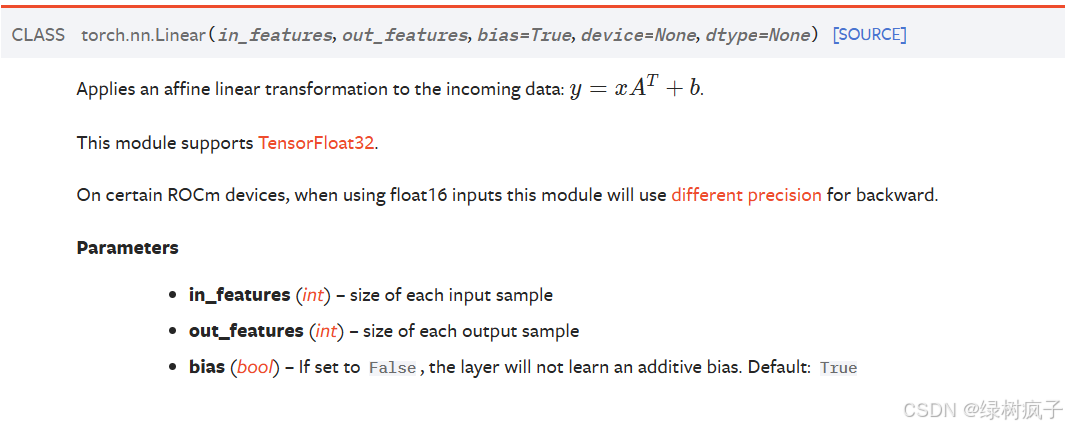
Linear Layers的weight和bias的初始化是正态分布,可参考官方文档。
2.3代码实战:
python
import torch
import torchvision
from torch import nn
from torch.nn import Linear
from torch.utils.data import DataLoader
dataset = torchvision.datasets.CIFAR10("./data", train=False,
transform=torchvision.transforms.ToTensor(), download=True)
dataloader = DataLoader(dataset, batch_size=64)
class Tudui(nn.Module):
def __init__(self):
super(Tudui,self).__init__()
self.linear1 = Linear(196608,10)
def forward(self, input):
output = self.linear1(input)
return output
tudui=Tudui()
for data in dataloader:
imgs, targets = data
print(imgs.shape)
output=torch.flatten(imgs)
print(output.shape)
output = tudui(output)
print(output.shape)

torch.flatten()可以展平数据