参考:
https://huggingface.co/Qwen/Qwen2-VL-2B-Instruct
模型:
c
export HF_ENDPOINT=https://hf-mirror.com
huggingface-cli download --resume-download --local-dir-use-symlinks False Qwen/Qwen2-VL-2B-Instruct --local-dir qwen2-vl
安装:
transformers-4.45.0.dev0
accelerate-0.34.2 safetensors-0.4.5
c
pip install git+https://github.com/huggingface/transformers
pip install 'accelerate>=0.26.0'
代码:
单张图片
c
from PIL import Image
import requests
import torch
from torchvision import io
from typing import Dict
from transformers import Qwen2VLForConditionalGeneration, AutoTokenizer, AutoProcessor
# Load the model in half-precision on the available device(s)
model = Qwen2VLForConditionalGeneration.from_pretrained(
"/ai/qwen2-vl", torch_dtype="auto", device_map="auto"
)
processor = AutoProcessor.from_pretrained("/ai/qwen2-vl")
# Image
url = "https://qianwen-res.oss-cn-beijing.aliyuncs.com/Qwen-VL/assets/demo.jpeg"
image = Image.open(requests.get(url, stream=True).raw)
conversation = [
{
"role": "user",
"content": [
{
"type": "image",
},
{"type": "text", "text": "Describe this image."},
],
}
]
# Preprocess the inputs
text_prompt = processor.apply_chat_template(conversation, add_generation_prompt=True)
# Excepted output: '<|im_start|>system\nYou are a helpful assistant.<|im_end|>\n<|im_start|>user\n<|vision_start|><|image_pad|><|vision_end|>Describe this image.<|im_end|>\n<|im_start|>assistant\n'
inputs = processor(
text=[text_prompt], images=[image], padding=True, return_tensors="pt"
)
inputs = inputs.to("cuda")
# Inference: Generation of the output
output_ids = model.generate(**inputs, max_new_tokens=128)
generated_ids = [
output_ids[len(input_ids) :]
for input_ids, output_ids in zip(inputs.input_ids, output_ids)
]
output_text = processor.batch_decode(
generated_ids, skip_special_tokens=True, clean_up_tokenization_spaces=True
)
print(output_text)
这是图片:
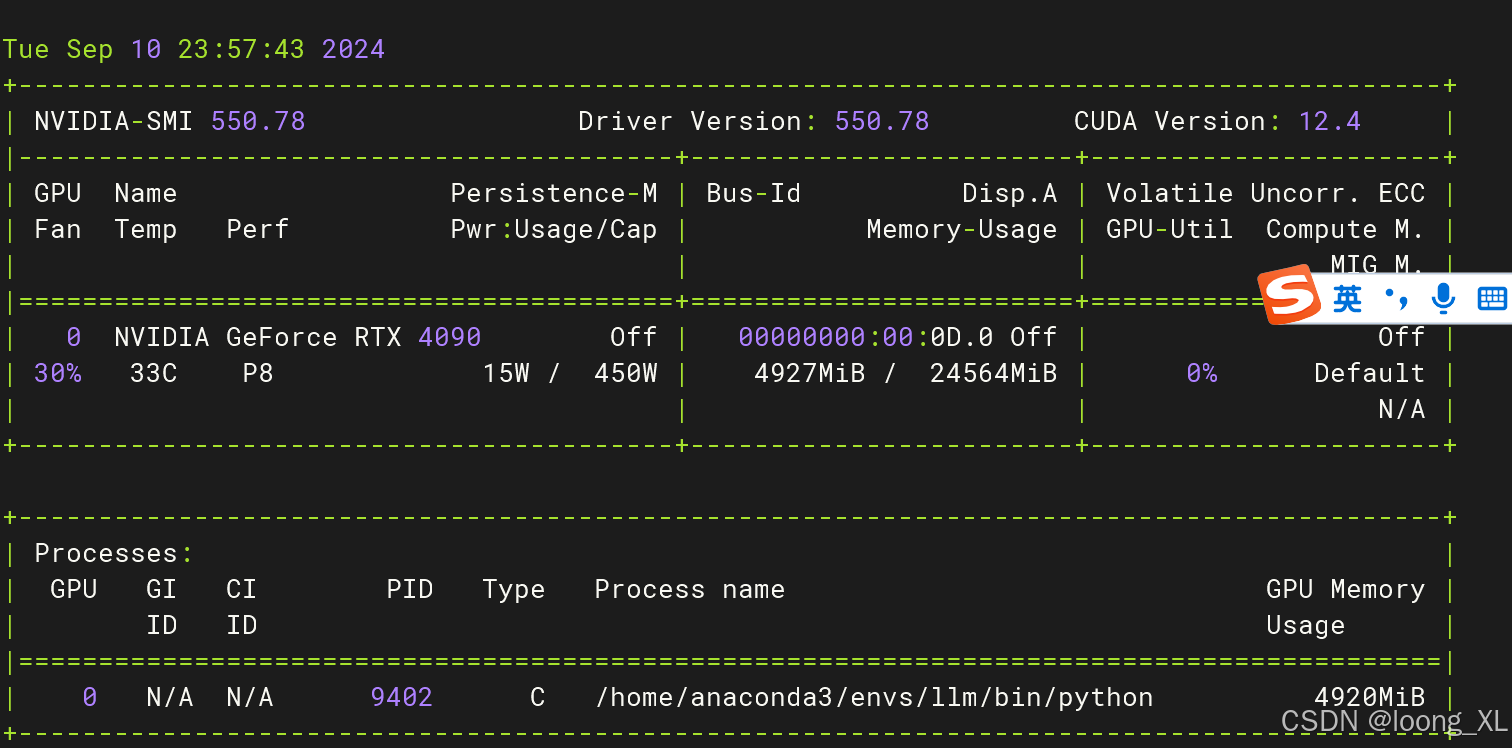
中文问
c
# Image
url = "https://qianwen-res.oss-cn-beijing.aliyuncs.com/Qwen-VL/assets/demo.jpeg"
image = Image.open(requests.get(url, stream=True).raw)
conversation = [
{
"role": "user",
"content": [
{
"type": "image",
},
{"type": "text", "text": "描述下这张图片."},
],
}
]
# Preprocess the inputs
text_prompt = processor.apply_chat_template(conversation, add_generation_prompt=True)
# Excepted output: '<|im_start|>system\nYou are a helpful assistant.<|im_end|>\n<|im_start|>user\n<|vision_start|><|image_pad|><|vision_end|>Describe this image.<|im_end|>\n<|im_start|>assistant\n'
inputs = processor(
text=[text_prompt], images=[image], padding=True, return_tensors="pt"
)
inputs = inputs.to("cuda")
# Inference: Generation of the output
output_ids = model.generate(**inputs, max_new_tokens=128)
generated_ids = [
output_ids[len(input_ids) :]
for input_ids, output_ids in zip(inputs.input_ids, output_ids)
]
output_text = processor.batch_decode(
generated_ids, skip_special_tokens=True, clean_up_tokenization_spaces=True
)
print(output_text)
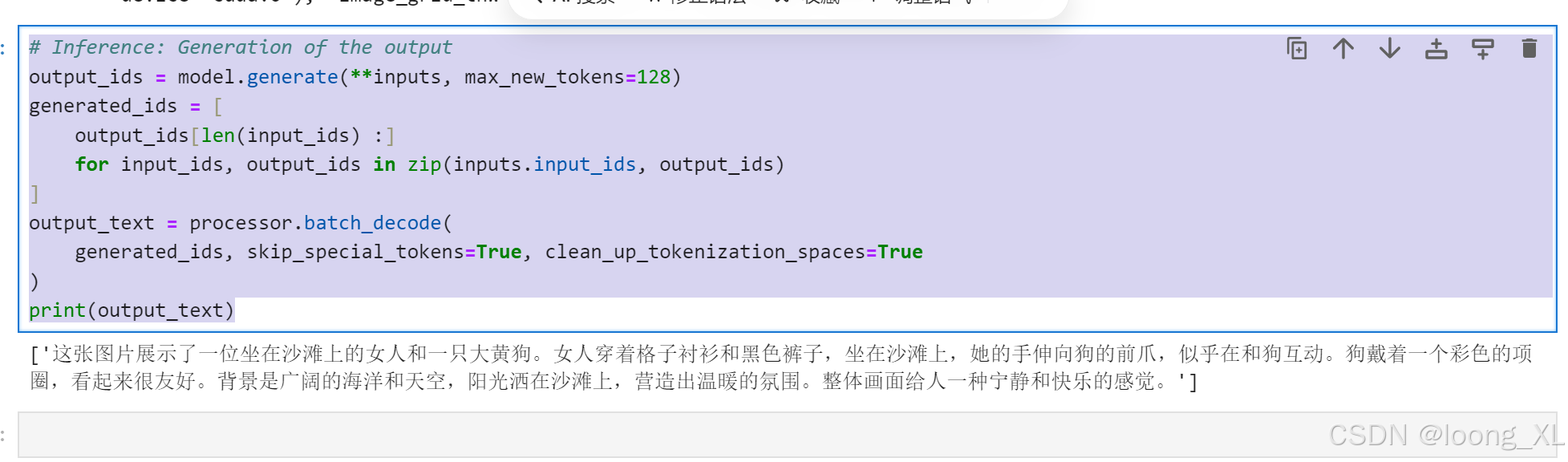
多张图片
c
def load_images(image_info):
images = []
for info in image_info:
if "image" in info:
if info["image"].startswith("http"):
image = Image.open(requests.get(info["image"], stream=True).raw)
else:
image = Image.open(info["image"])
images.append(image)
return images
# Messages containing multiple images and a text query
messages = [
{
"role": "user",
"content": [
{"type": "image", "image": "/ai/fight.png"},
{"type": "image", "image": "/ai/long.png"},
{"type": "text", "text": "描述下这两张图片"},
],
}
]
# Load images
image_info = messages[0]["content"][:2] # Extract image info from the message
images = load_images(image_info)
# Preprocess the inputs
text_prompt = processor.apply_chat_template(messages, add_generation_prompt=True)
inputs = processor(
text=[text_prompt], images=images, padding=True, return_tensors="pt"
)
inputs = inputs.to("cuda")
# Inference: Generation of the output
output_ids = model.generate(**inputs, max_new_tokens=128)
generated_ids = [
output_ids[len(input_ids) :]
for input_ids, output_ids in zip(inputs.input_ids, output_ids)
]
output_text = processor.batch_decode(
generated_ids, skip_special_tokens=True, clean_up_tokenization_spaces=True
)
print(output_text)
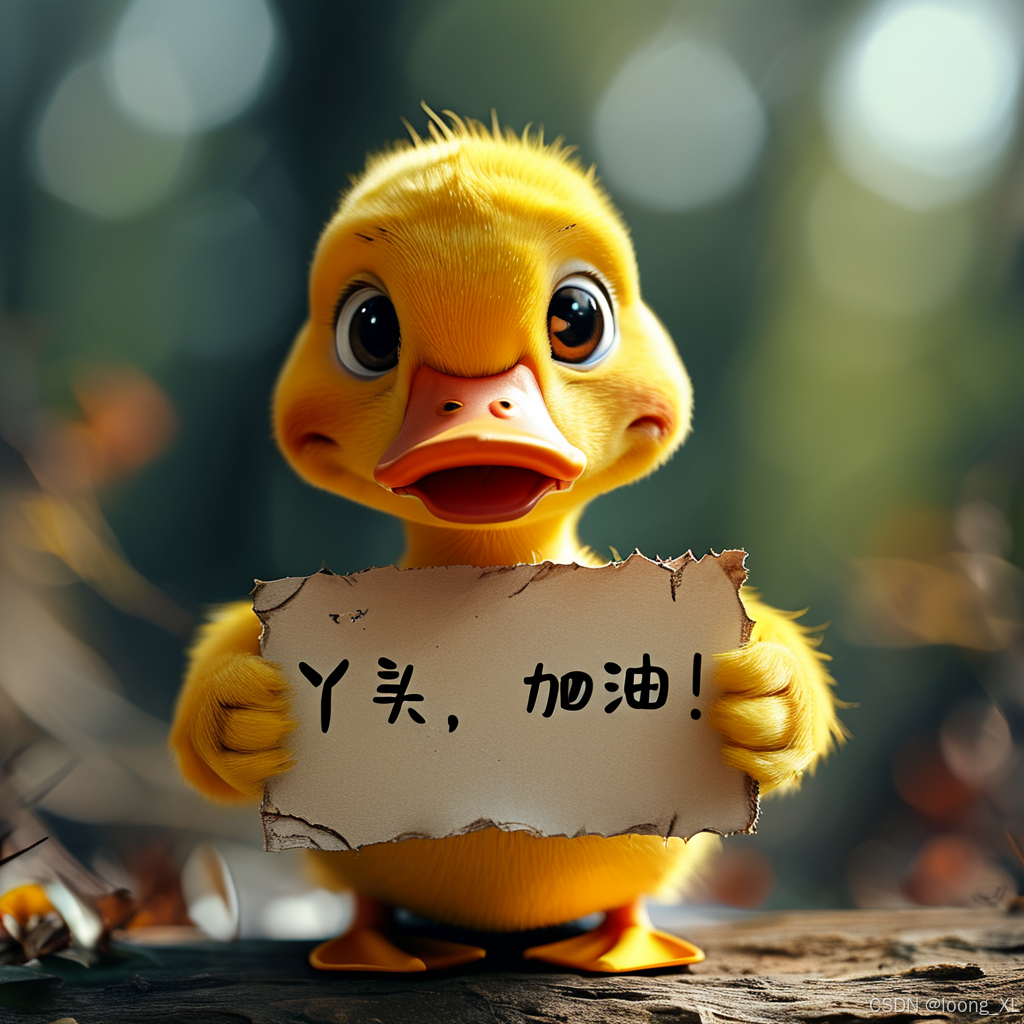
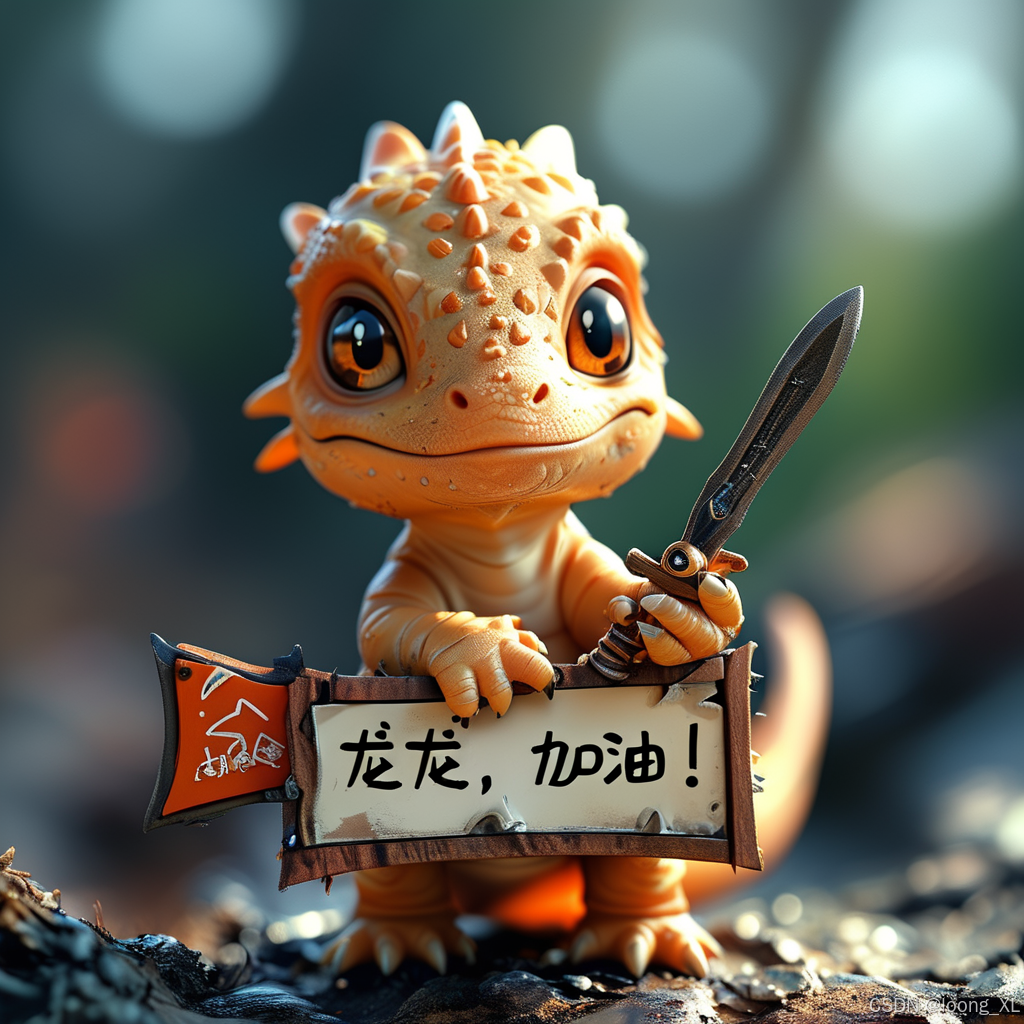
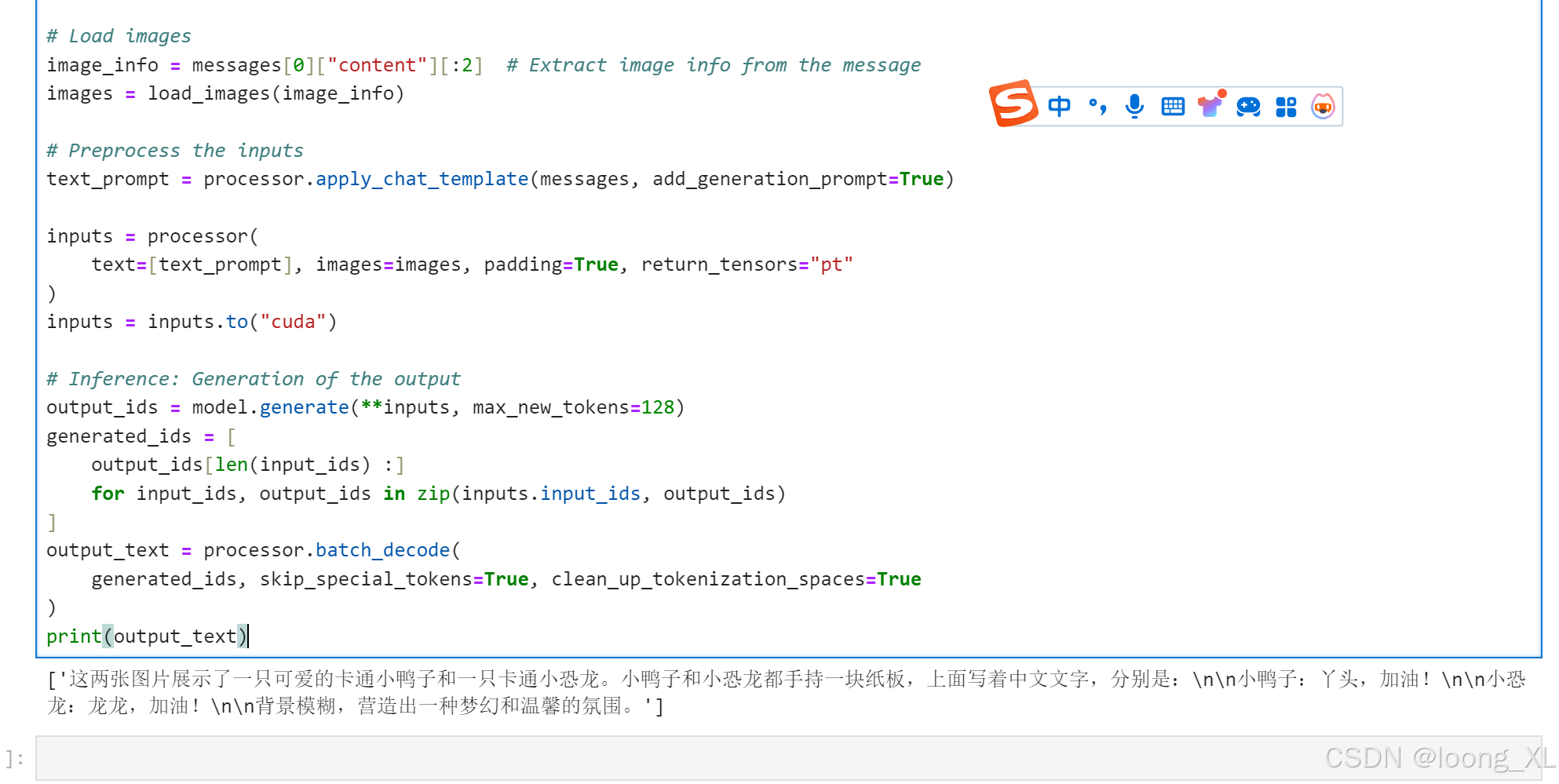
视频
安装
c
pip install qwen-vl-utils
c
from qwen_vl_utils import process_vision_info
# Messages containing a images list as a video and a text query
messages = [
{
"role": "user",
"content": [
{
"type": "video",
"video": [
"file:///path/to/frame1.jpg",
"file:///path/to/frame2.jpg",
"file:///path/to/frame3.jpg",
"file:///path/to/frame4.jpg",
],
"fps": 1.0,
},
{"type": "text", "text": "Describe this video."},
],
}
]
# Messages containing a video and a text query
messages = [
{
"role": "user",
"content": [
{
"type": "video",
"video": "/ai/血液从上肢流入上腔静脉.mp4",
"max_pixels": 360 * 420,
"fps": 1.0,
},
{"type": "text", "text": "描述下这个视频"},
],
}
]
# Preparation for inference
text = processor.apply_chat_template(
messages, tokenize=False, add_generation_prompt=True
)
image_inputs, video_inputs = process_vision_info(messages)
inputs = processor(
text=[text],
images=image_inputs,
videos=video_inputs,
padding=True,
return_tensors="pt",
)
inputs = inputs.to("cuda")
# Inference
generated_ids = model.generate(**inputs, max_new_tokens=128)
generated_ids_trimmed = [
out_ids[len(in_ids) :] for in_ids, out_ids in zip(inputs.input_ids, generated_ids)
]
output_text = processor.batch_decode(
generated_ids_trimmed, skip_special_tokens=True, clean_up_tokenization_spaces=False
)
print(output_text)
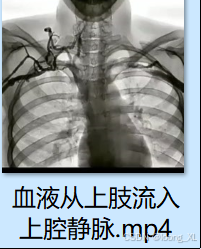
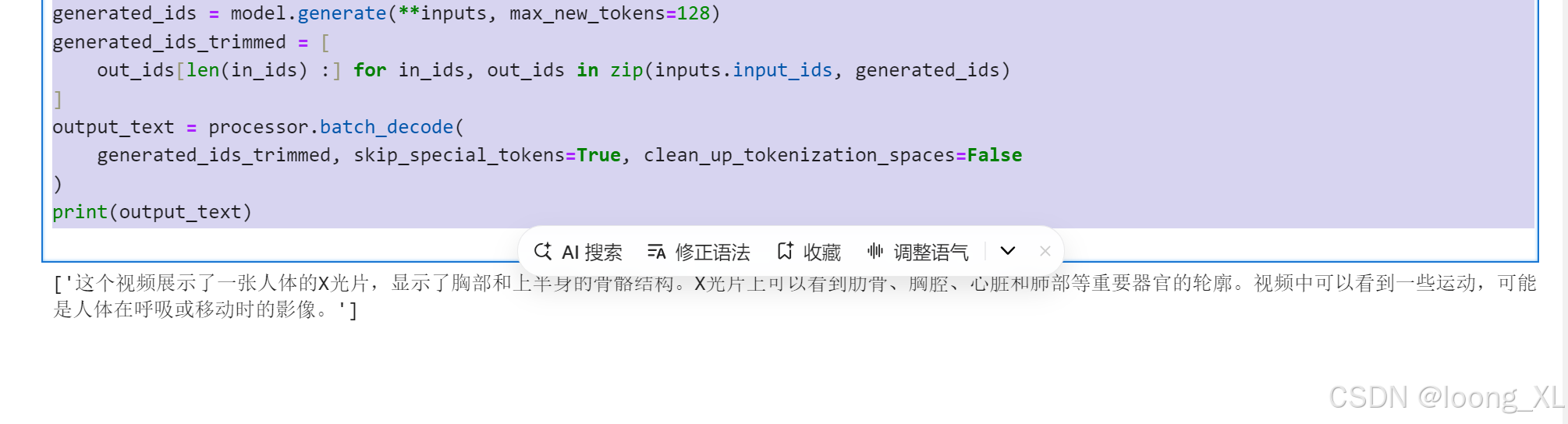