文章目录
- 1.接入AI
- 2.小助手的实现流程
- 3.Flask应用示例
-
- Python文件.py
- index.html
- 运行Flask应用
- [地址栏输入 http://localhost:5000/](#地址栏输入 http://localhost:5000/)
1.接入AI
获取API密钥
在智谱AI的官方网站上注册,右上角点击API密钥,新建并复制一个 API Key,不要在公开的代码中暴露你的API密钥
Python代码
在Jupyter Notebook中,发送HTTP请求到智谱AI的API,需要提前pip install zhipuai
运行代码,看到 AI 的回复
python
from zhipuai import ZhipuAI
client = ZhipuAI(api_key="") # 填写自己的APIKey
response = client.chat.completions.create(
model="glm-4-0520", # 填写需要调用的模型编码
messages=[
{"role": "system", "content": "你是一个乐于解答各种问题的助手,你的任务是为用户提供专业、准确、有见地的建议。"},
{"role": "user", "content": "我对太阳系的行星非常感兴趣,特别是土星。请提供关于土星的基本信息,包括其大小、组成、环系统和任何独特的天文现象。"},
],
stream=True,
)
for chunk in response:
print(chunk.choices[0].delta)
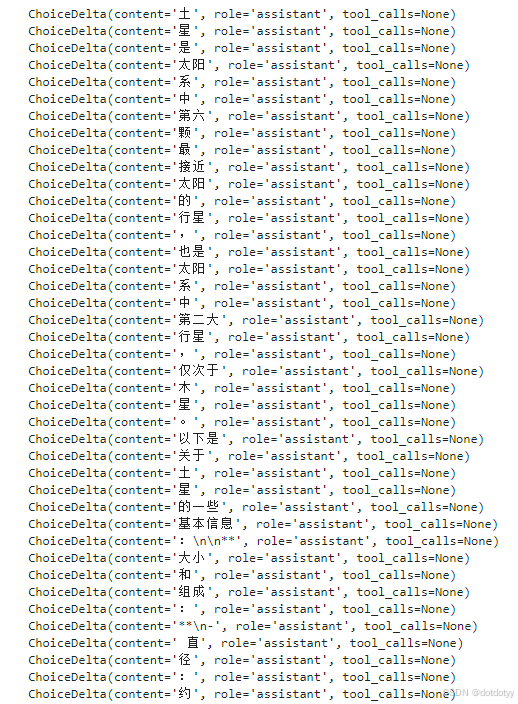
2.小助手的实现流程
(1)提供求职者的简历内容,输入给 AI 面试官,让其分析并生成面试问题
(2)将生成的问题逐一输入给 AI 求职者,让其给出答案
(3)对 AI 生成的结果进行组合整理
python
from zhipuai import ZhipuAI
# 初始化ZhipuAI客户端
client = ZhipuAI(api_key="") # ""填写自己的APIKey
def generate_interview_questions(resume):
# 构建系统消息,描述面试官的角色和任务
system_message = {
"role": "system",
"content": "你是一位经验丰富的 AI 面试官,下面我会给你一份求职者的简历,请分析简历并提出相关的面试问题。要求输出格式如下,每个问题一行,此外不要有任何多余的内容:{序号}. {面试问题}"
}
# 构建用户消息,包含简历内容
user_message = {"role": "user", "content": resume}
# 调用API生成面试问题
response = client.chat.completions.create(
model="glm-4-0520", # 填写需要调用的模型编码
messages=[system_message, user_message],
stream=True,
)
# 处理API响应,生成面试问题列表
questions = []
current_question = ""
for chunk in response:
delta = chunk.choices[0].delta
if delta.content:
current_question += delta.content
if current_question.endswith('.'):
questions.append(current_question.strip())
current_question = ""
if len(questions) == 10: # 生成10个问题后停止
break
return questions
# 示例简历
user_resume = "Java程序员,工作经验3年,熟悉MySQL、Redis,有过电商项目经历"
# 生成面试问题
interview_questions = generate_interview_questions(user_resume)
# 打印面试问题
for i, question in enumerate(interview_questions, start=1):
print(f"{i}. {question}")
# 假设我们有一个函数来调用AI求职者系统,并使用生成的面试问题来获取回答
def invoke(prompt, user_prompt):
response = client.chat.completions.create(
model="glm-4-0520",
messages=[{"role": "system", "content": prompt}, {"role": "user", "content": user_prompt}],
max_tokens=150, # 增加最大token数以获取更完整的回答
stop=None,
temperature=0.7
)
return response.choices[0].message.content.strip()
# 遍历面试问题列表,每个问题都要调用一次 AI 求职者
question_answer_map = {}
for question in interview_questions:
user_prompt = f"---个人简历---\n{user_resume}\n---面试问题---\n{question}"
ai_applicant_reply = invoke(AI_APPLICANT_SYSTEM_PROMPT, user_prompt)
question_answer_map[question] = ai_applicant_reply.strip()
# 打印问题和答案
for question, answer in question_answer_map.items():
print(f"{question}\n{answer}\n")
# 输出问题答案映射
print(question_answer_map)
3.Flask应用示例
助手集成到一个Python Flask应用中
开发环境中已安装Flask、zhipuai
目录结构
Python文件.py
在你的项目目录下创建一个新的Python文件,app.py,代码:
python
from flask import Flask, render_template, request
from zhipuai import ZhipuAI
app = Flask(__name__)
client = ZhipuAI(api_key="") # 请填写自己的APIKey
def generate_interview_questions(resume):
system_message = {
"role": "system",
"content": "你是一位经验丰富的 AI 面试官,下面我会给你一份求职者的简历,请分析简历并提出相关的面试问题。要求输出格式如下,每个问题一行,此外不要有任何多余的内容:{序号}. {面试问题}"
}
user_message = {"role": "user", "content": resume}
response = client.chat.completions.create(
model="glm-4-0520",
messages=[system_message, user_message],
stream=True,
)
questions = []
current_question = ""
for chunk in response:
delta = chunk.choices[0].delta
if delta.content:
current_question += delta.content
if current_question.endswith('.'):
questions.append(current_question.strip())
current_question = ""
if len(questions) == 11:
break
return questions
def invoke(prompt, user_prompt):
response = client.chat.completions.create(
model="glm-4-0520",
messages=[{"role": "system", "content": prompt}, {"role": "user", "content": user_prompt}],
max_tokens=500,
stop=None,
temperature=0.7
)
return response.choices[0].message.content.strip()
@app.route('/', methods=['GET', 'POST'])
def index():
questions_answers = {}
if request.method == 'POST':
resume = request.form['resume']
interview_questions = generate_interview_questions(resume)
for question in interview_questions:
ai_response = invoke("你的系统提示", f"---个人简历---\n{resume}\n---面试问题---\n{question}")
questions_answers[question] = ai_response.strip()
return render_template('index.html', questions_answers=questions_answers)
if __name__ == '__main__':
app.run(debug=True)
index.html
xml
<!DOCTYPE html>
<html lang="zh">
<head>
<meta charset="UTF-8">
<meta name="viewport" content="width=device-width, initial-scale=1.0">
<title>面试助手</title>
</head>
<body>
<h1>AI 面试助手</h1>
<form method="POST">
<label for="resume">输入简历:</label><br>
<textarea id="resume" name="resume" rows="5" cols="40" required></textarea><br>
<input type="submit" value="生成面试问题">
</form>
<h2>生成的面试问题和答案</h2>
<ul>
{% for question, answer in questions_answers.items() %}
<li><strong>{{ question }}</strong>: {{ answer }}</li>
{% endfor %}
</ul>
</body>
</html>
运行Flask应用
在终端中,导航到项目目录并运行以下命令:
python app.py
此时,Flask应用现在应该在 http://localhost:5000/ 运行
地址栏输入 http://localhost:5000/
显示如下,简历输入"Java程序员,工作经验3年,熟悉MySQL、Redis,有过电商项目经历"
点击按钮,过一会儿会出现