关于代码中导入的模块, 个人更建议把导入的各个模块放在代码最前面, 有利于后期封装函数
当然, 对于新手来说, 我的建议是模块在使用的时候导入, 这样学习的印象更深刻,
等到知识和代码都熟练了, 再改也不迟
python
# 1.导入外部数据集breast-cancer-wisconsin
import pandas as pd
names=["Sample code number","Clump Thickness","Uniformity of Cell Size","Uniformity of Cell Shape",
"Marginal Adhesion","Single Epithelial Cell Size","Bare Nuclei","Bland Chromatin","Normal Nucleoli", "Mitoses","Class"]
cancer_data=pd.read_csv(r"C:\Users\鹰\Desktop\ML_Set\breast_cancer_wisconsin\breast-cancer-wisconsin.data", names=names)
# 2.数据基本处理- 缺失值处理, 确定特征值和目标值, 数据集分割
# 缺失值处理, 关于缺失值, 特殊字符和异常值的检测和处理, 教学视频内没有讲, 怎么搞?
import numpy as np
print(cancer_data.isna().sum())
cancer_data=cancer_data.replace(to_replace="?", value=np.nan)
cancer_data=cancer_data.dropna()
# 确定特征值, 目标值
x_all=cancer_data.iloc[:, 1:-1]
y_all=cancer_data.iloc[:,-1]
# 分割数据集
from sklearn.model_selection import train_test_split
x_train,x_test,y_train,y_test=train_test_split(x_all,y_all, test_size=0.2)
# 3.特征工程-特征预处理
# 特征预处理-标准化
from sklearn.preprocessing import StandardScaler
scaler=StandardScaler()
scaler.fit_transform(x_train)
scaler.fit_transform(x_test)
# 4.模型训练-逻辑回归
from sklearn.linear_model import LogisticRegression
estimator=LogisticRegression()
estimator.fit(x_train,y_train)
# 5.模型评估-预测值, 准确率, 分类模型的评估指标[精确率, 召回率, f1-score, AUC]
# 预测值
y_predict=estimator.predict(x_test)
print("predict_values :", y_predict)
# 准确率
score=estimator.score(x_test, y_test)
print("accuracy :", score)
# 精确率, 召回率, f1-score
from sklearn.metrics import classification_report
class_report=classification_report(y_test, y_predict,labels=(2,4),target_names=("Benign tumor(良性肿瘤)", "Malignant tumor(恶性肿瘤)"))
print(class_report)
# AUC指标, 适合评估不平衡二分类问题
y_test=np.where(y_test>3,1,0)
from sklearn.metrics import roc_auc_score
AUC=roc_auc_score(y_test,y_predict)
print("AUC :", AUC)
为了防止失效, 我就多放几个地址, 理解万岁,
第一个直接去官网下载数据集, 第二个是我通过百度网盘分享的链接
地址1:
兄弟们, 注意啊,刻骨铭心的教训 当进入UCI网站时, 收索乳腺癌肿瘤预测, 会查找到三个数据集, 咱们应该选择数据集后面标注original, 样本数量为699的数据集
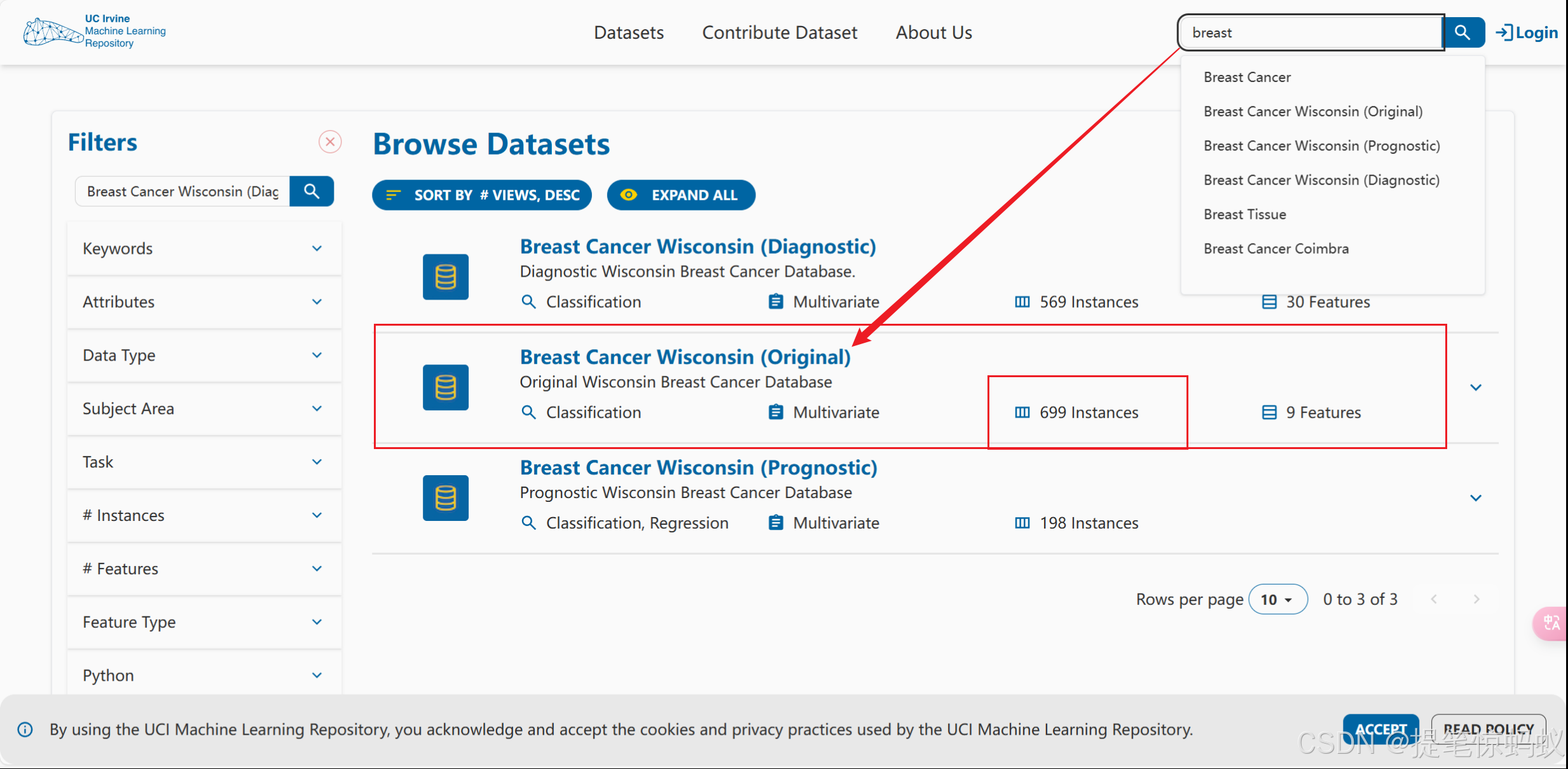
地址2:
链接:https://pan.baidu.com/s/1sTJdDaj_pXUvurlCWzWFDQ
提取码:dzlk