作者:来自 Elastic Han Xiang Choong
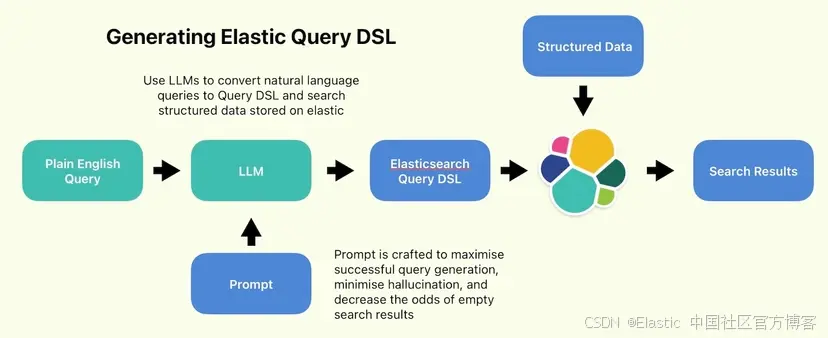
这篇简短的文章是关于将结构化数据上传到 Elastic 索引,然后将纯英语查询转换为查询 DSL 语句,以使用特定过滤器和范围搜索特定条件。完整代码位于此 Github repo 中。
首先,运行以下命令安装依赖项(Elasticsearch 和 OpenAI):
pip install elasticsearch==8.14.0 openai==1.35.13
你将需要一个 Elastic Cloud 部署和一个 Azure OpenAI 部署来跟进此笔记本。有关更多详细信息,请参阅此 readme 文件。
如果这些可用,请填写下面的 env.example 并将其重命名为 .env:
ELASTIC_ENDPOINT=<YOUR ELASTIC ENDPOINT>
ELASTIC_API_KEY=<YOUR ELASTIC API KEY>
ELASTIC_INDEX_NAME=<YOUR ELASTIC INDEX NAME>
AZURE_OPENAI_KEY_1=<AZURE OPEN AI API KEY>
AZURE_OPENAI_KEY_2=<AZURE OPEN AI API KEY>
AZURE_OPENAI_REGION=<AZURE OPEN AI API REGION>
AZURE_OPENAI_ENDPOINT=<AZURE OPEN AI API ENDPOINT>
最后,打开 main.ipynb 笔记本,并按顺序执行每个单元。请注意,代码将向你选择的 Elastic 索引上传大约 200,000 个数据点,大约 400MB。
本文探讨的流程
问题陈述
RAG 和混合搜索是当今最流行的搜索方式。将非结构化文档上传到索引,然后查询它,并在流程的每一步都使用机器学习,这一前景被证明是一个用途广泛的想法,拥有大量用例。
现在,数量庞大并非无限。尽管 RAG 用途广泛,但有时 RAG 要么过度使用,要么根本不适合数据。一个这样的例子是包含如下数据点的结构化数据集:
{
'address': {'block': '827',
'postal_code': '705858',
'street': 'Commonwealth Avenue',
'street No.': '756',
'town': 'Sengkang',
'unit': '#15-042'},
'age': 65,
'blood_type': 'A+',
'citizenship': 'Singapore PR',
'country_of_birth': 'Slovakia',
'cpf_number': 'S8613060M',
'date_of_birth': '1958-10-05',
'deceased': False,
'drivers_license_number': 'S4707782F',
'education': {'highest_qualification': 'N-Levels',
'institution': 'Local Polytechnic'},
'email': '[email protected]',
'emergency_contact': {'name': 'Deng An Sheng',
'phone_number': '+65 8163 9924',
'relationship': 'Sibling'},
'gender': 'Male',
'height_cm': 166,
'immigration_status': None,
'languages': {'spoken': {'Afrikaans': 'Basic', 'Xhosa': 'Native'},
'written': {'Bengali': 'Native'}},
'marital_status': 'Married',
'name': 'Wong Jiu Zhan',
'national_service': {'rank': 'Captain', 'status': 'NSman'},
'nric': 'S77385949H',
'occupation': 'Sociologist',
'passport_number': 'K8013743A',
'phone_number': '+65 9395 8462',
'race': 'Others',
'religion': 'Taoism',
'weight_kg': 92
}
对我来说,使用这种结构化数据进行基于向量的 RAG 没什么意义。要做到这一点,你可能需要尝试嵌入字段值,但这有几个问题。
首先,并非所有单个字段都是相等的。根据用例,某些字段会更受关注,因此应该赋予更高的权重(血型、年龄、体重和已故,如果你从事医学领域,也许可以这样?)。如果嵌入每个字段,然后进行加权求和,则可以对字段进行加权。这将使嵌入的计算成本乘以你拥有的字段数 - 在本例中约为 37 倍。如果不这样做,相关字段将被埋没在无关字段之下,性能可能会很差。糟糕的选择。
其次,即使你确实在每个数据点中嵌入了每个字段,你到底在查询什么?一个简单的英语问题,例如 "Adult men over the age of 25" ?向量无法像这样根据特定标准进行过滤 - 它们纯粹基于统计相关性工作,这很难明确。我敢打赌这些查询的结果很差,甚至根本无法给出结果。
数据
我们使用的数据不包括真实人物的个人资料。我编写了一个数据生成器来生成假的新加坡人。它是一系列随机选择语句,每个选择都从大量职业、出生国家、地址等选项中进行选择......有几个函数可以生成令人信服的护照、电话和 NRIC 号码。现在这个假数据生成器本身并不是特别有趣,所以我不会在这里讨论它。生成器本身位于相关笔记本上 - 请随意查看!我为这次测试生成了 352,500 个个人资料。
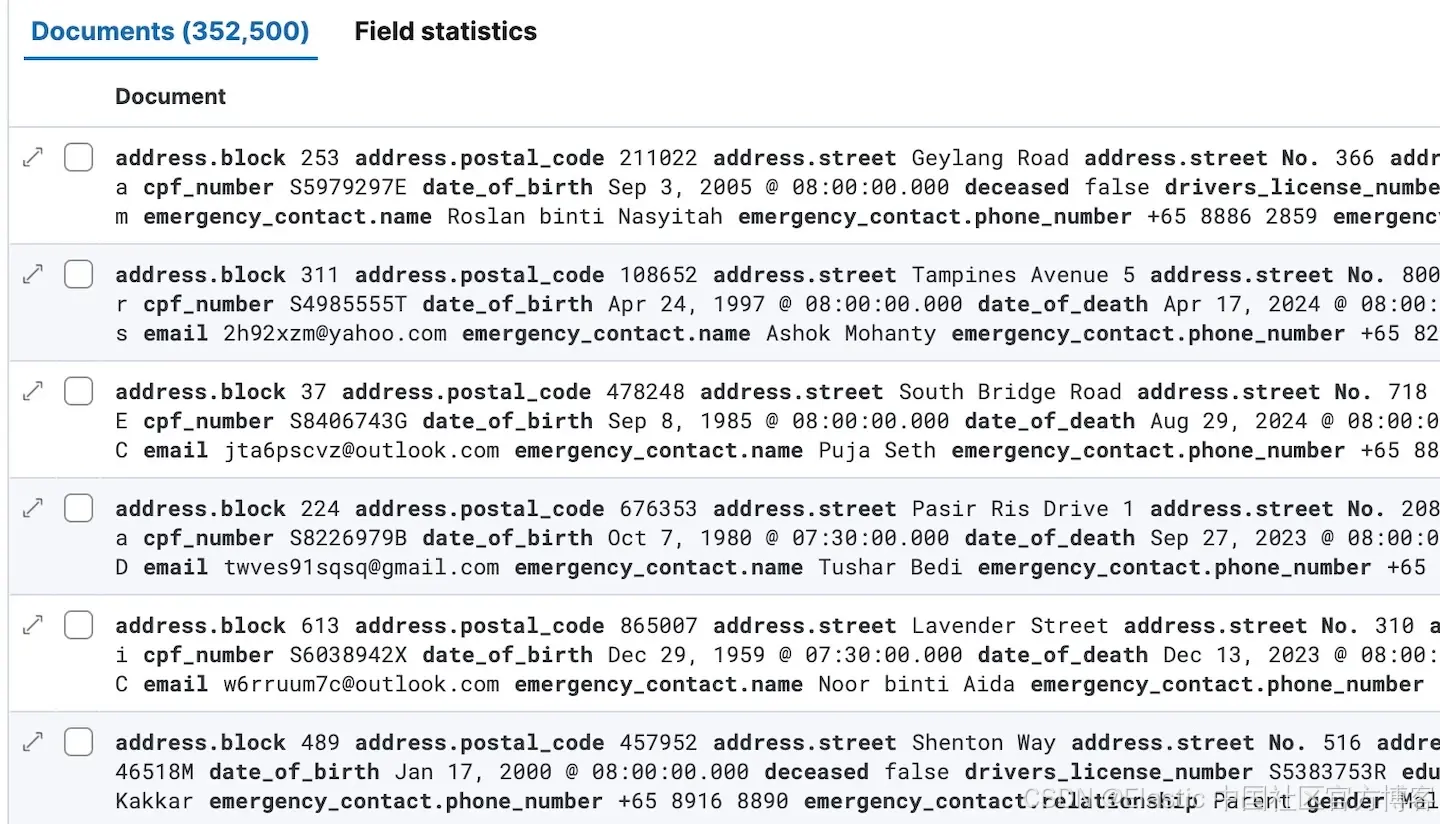
解决方案
传统搜索是一种更有效的选择,因为它具有明确的过滤器和范围。Elastic 是一个功能齐全的搜索引擎,具有远远超出向量的大量搜索功能。这是搜索的一个方面,最近被有关 RAG 和向量的讨论所掩盖。我认为这代表着错过了一个在相关性(搜索引擎性能)方面获得重大提升的机会,因为在昂贵的向量搜索发挥作用之前,就有可能过滤掉大量不相关的数据(这可能会产生统计噪音,使结果恶化)。
然而,交付和形式因素是重大障碍。最终用户可能不需要学习 SQL 或 Elastic Query DSL 等数据库查询语言,尤其是如果他们一开始就不懂技术的话。理想情况下,我们希望保留用户使用普通的自然语言查询数据的想法。让用户能够搜索你的数据库,而无需摆弄过滤器或疯狂地谷歌搜索如何编写 SQL 或 QDSL 查询。
在 LLM 时代之前,解决这个问题的办法可能是编写一些按钮并将它们标记为查询的特定组件。然后,你可以通过选择选项列表来构建复杂的查询。但这样做的缺点是,最终会变成一个非常丑陋的用户界面,里面充满了大量按钮。如果你只有一个聊天框可以搜索,那不是更好吗?
是的,我们将编写一个自然语言查询,将其传递给 LLM,生成一个 Elastic 查询,并使用它来搜索我们的数据。
提示设计 - prompt design
这是一个简单的概念,唯一需要解决的挑战是提示写作。我们应该写一个具有几个关键特征的提示:
1. 文档模式 - document schema
它包含数据模式,因此 LLM 知道哪些字段有效并且可以查询,哪些不能。像这样:
The document schema for the profiles is as follows:
{
"nric": "string",
"name": "string",
"race": "string",
"gender": "string",
"date_of_birth": "date",
"age": "integer",
"country_of_birth": "string",
"citizenship": "string",
"religion": "string" ["Buddhism", "Christianity", "Islam", "Hinduism", "Taoism", "No Religion"],
"marital_status": "string" ["Single", "Married", "Divorced", "Separated", "Widowed", "Civil Partnership", "Domestic Partnership", "Engaged", "Annulled"],
"address": {
"block": "string",
"street_no": "string",
"street": "string",
"unit": "string",
"town": "string",
"postal_code": "string"
},
"phone_number": "string",
"email": "string",
"occupation": "string",
"cpf_number": "string",
"education": {
"highest_qualification": "string",
"institution": "string"
},
"languages": {
"spoken": {"language":"fluency" ["Basic", "Conversational", "Fluent", "Native"]},
"written": {"language":"fluency" ["Basic", "Conversational", "Fluent", "Native"]},
},
"height_cm": "integer",
"weight_kg": "integer",
"blood_type": "string" ["A+", "A-", "B+", "B-", "O+", "O-", "ABß", "AB-"],
"passport_number": "string",
"drivers_license_number": "string",
"national_service": {
"status": "string",
"rank": "string"
},
"immigration_status": "string",
"emergency_contact": {
"name": "string",
"relationship": "string",
"phone_number": "string"
},
"deceased": "boolean",
"date_of_death": "date"
}
请注意,对于某些字段(例如 blood_type、religion 和 fluency),我明确定义了有效值以确保一致性。对于其他字段(例如 languages 或 streetnames),可用选项的数量过多,会导致提示膨胀。在这种情况下,最好的选择是信任 LLM。
2. 示例
它包含示例查询和指南,有助于减少无效的无意义查询的几率。
Example query:
User: Find all male Singapore citizens between 25 and 30 years old who work as software developers and speak fluent English.
Your response should be:
{
"query": {
"bool": {
"should": [
{ "match": { "gender": "Male" } },
{ "match": { "citizenship": "Singapore Citizen" } },
{ "range": { "age": { "gte": 25, "lte": 30 } } },
{ "match": { "occupation": "Software Developer" } },
{
"match": {
"languages.spoken.English": {
"query": "Fluent",
"fuzziness": "AUTO"
}
}
}
],
"minimum_should_match": 2
}
}
}
3. 特殊情况
它应该包含一些特殊情况,这些情况可能需要稍微更高级的查询:
Consider using multi_match for fields that might contain the value in different subfields:
{
"multi_match": {
"query": "Software Developer",
"fields": ["occupation", "job_title", "role"],
"type": "best_fields",
"fuzziness": "AUTO"
}
}
For names or other fields where word order matters, you might want to use match_phrase with slop:
{
"match_phrase": {
"full_name": {
"query": "John Doe",
"slop": 1
}
}
}
4. 宽大处理和覆盖范围
应包含避免过度依赖精确匹配的说明。我们希望鼓励模糊性和部分匹配,并避免使用布尔 AND 语句。这是为了抵消可能的幻觉,这种幻觉可能会导致不可行或不合理的条件,最终导致搜索结果列表为空。
Generate a JSON query for Elasticsearch. Provide only the raw JSON without any surrounding tags or markdown formatting, because we need to convert your response to an object.
Use a lenient approach with 'should' clauses instead of strict 'must' clauses. Include a 'minimum_should_match' parameter to ensure some relevance while allowing flexibility. Avoid using 'must' clauses entirely.
All queries must be lowercase.
Use 'match' queries instead of 'term' queries to allow for partial matches and spelling variations. Where appropriate, include fuzziness parameters to further increase tolerance for spelling differences.
For name fields or other phrases where word order matters, consider using 'match_phrase' with a slop parameter. Use 'multi_match' for fields that might contain the value in different subfields.
If some criteria are strict, please judiciously use term queries and must clauses appropriately.
一旦我们有了满足所有这些标准的提示,我们就可以进行测试了!
测试程序
我们将定义一个类来调用我们的 Azure OpenAI LLM:
class AzureOpenAIClient:
def __init__(self):
self.client = AzureOpenAI(
api_key=os.environ.get("AZURE_OPENAI_KEY_1"),
api_version="2024-06-01",
azure_endpoint=os.environ.get("AZURE_OPENAI_ENDPOINT")
)
def generate_non_streaming_response(self, prompt, model="gpt-4o", system_prompt=""):
response = self.client.chat.completions.create(
model=model,
messages=[
{"role": "system", "content": system_prompt},
{"role": "user", "content": prompt}
],
max_tokens=4096
)
return response.choices[0].message.content
LLM = AzureOpenAIClient()
并将查询与我们刚刚定义的提示一起传递给 LLm,如下所示:
query="All non-Singaporean men over the age of 25 who are software people living in woodlands"
response=LLM.generate_non_streaming_response(query, system_prompt=prompt)
es_query=json.loads(response)
pprint(es_query)
提示中的这一行应该允许我们直接将 LLM 的响应加载为 JSON 对象,而无需进行任何进一步的处理:
Generate a JSON query for Elasticsearch. Provide only the raw JSON without any surrounding tags or markdown formatting, because we need to convert your response to an object.
现在让我们进行一些测试并看看它的表现如何:
测试 1
首先,让我们测试一下 LLM 处理简单标准和处理一些模糊性的能力。我期望一个简单的年龄范围、男性和软件相关职业的模糊匹配以及新加坡公民的否定匹配。
查询:
所有 25 岁以上居住在 woodlands 的软件人员非新加坡男性
生成的 Elastic 查询:
{
"query": {
"bool": {
"minimum_should_match": 3,
"must_not": [
{
"match": {
"citizenship": "singapore"
}
}
],
"should": [
{
"match": {
"gender": "male"
}
},
{
"range": {
"age": {
"gt": 25
}
}
},
{
"multi_match": {
"fields": [
"occupation"
],
"fuzziness": "AUTO",
"query": "software",
"type": "best_fields"
}
},
{
"match_phrase": {
"address.town": {
"query": "woodlands",
"slop": 1
}
}
}
]
}
}
}
结果:
Total matches: 7742
Score: 8.3714
Name: Xiao E
Age: 78
Gender: Male
Citizenship: Foreigner
Occupation: Software Developer
Address: {'town': 'Woodlands', 'postal_code': '838414'}
---
Score: 8.3714
Name: Zafran binti Kamaruzaman
Age: 67
Gender: Male
Citizenship: Foreigner
Occupation: Software Developer
Address: {'town': 'Woodlands', 'postal_code': '790670'}
---
Score: 8.3714
Name: Saffuan binti Sofiah
Age: 48
Gender: Male
Citizenship: Foreigner
Occupation: Software Developer
Address: {'town': 'Woodlands', 'postal_code': '352474'}
---
好的。这基本上就是我们所期望的。只有完全相关的结果。让我们尝试更难的测试。
测试 2
让我们做一个更模糊的查询。我期望对目前已故且出生在新加坡的 O 型血的人有更明确的标准。
查询:
目前已去世且出生在新加坡的全民献血者女性
生成的 Elastic 查询:
{
"query": {
"bool": {
"minimum_should_match": 3,
"should": [
{
"match": {
"gender": "female"
}
},
{
"match": {
"deceased": true
}
},
{
"bool": {
"minimum_should_match": 1,
"should": [
{
"match": {
"blood_type": "o-"
}
}
]
}
},
{
"match": {
"country_of_birth": "singapore"
}
}
]
}
}
}
结果:
Total matches: 10000
Score: 7.8673687
Name: Bharat D'Souza
Blood Type: O-
Gender: Female
Country of Birth: Singapore
Deceased: True
---
Score: 7.8673687
Name: Neeti Kochhar
Blood Type: O-
Gender: Female
Country of Birth: Singapore
Deceased: True
---
Score: 7.8673687
Name: Shanta Somani
Blood Type: O-
Gender: Female
Country of Birth: Singapore
Deceased: True
---
这些结果完全符合预期,向量甚至没有出现在任何步骤中。当你有锤子时,自然的倾向是寻找钉子来敲打。在这种情况下,我认为嵌入数据不会产生任何积极的结果来证明添加嵌入模型所增加的成本和复杂性是合理的。
测试 3
最后一个测试 - 让我们看看它是否可以处理嵌套属性,例如语言字段。
查询:
说中文方言的人
生成的 Elastic 查询:
{
"query": {
"bool": {
"minimum_should_match": 1,
"should": [
{
"match": {
"languages.spoken.Chinese": "Fluent"
}
},
{
"match": {
"languages.spoken.Mandarin": "Fluent"
}
},
{
"match": {
"languages.spoken.Cantonese": "Fluent"
}
},
{
"match": {
"languages.spoken.Hokkien": "Fluent"
}
},
{
"match": {
"languages.spoken.Teochew": "Fluent"
}
},
{
"match": {
"languages.spoken.Hakka": "Fluent"
}
},
{
"match": {
"languages.spoken.Hainanese": "Fluent"
}
}
]
}
}
}
结果:
Total matches: 10000
Score: 2.8383636
Name: Hadi binti Saleh
languages: {'spoken': {'Hainanese': 'Fluent', 'Esperanto': 'Fluent', 'Hakka': 'Fluent'}, 'written': {'Slovenian': 'Native', 'Mongolian': 'Basic', 'Dutch': 'Fluent'}}
---
Score: 2.8383636
Name: Liu Zai Su
languages: {'spoken': {'Hainanese': 'Fluent', 'Amharic': 'Native', 'Hakka': 'Fluent'}, 'written': {'Sinhala': 'Fluent'}}
---
Score: 2.8383636
Name: Kamal binti Zarina
languages: {'spoken': {'Zulu': 'Basic', 'Hakka': 'Fluent', 'Hainanese': 'Fluent'}, 'written': {'Armenian': 'Fluent', 'Spanish': 'Fluent'}}
---
使用 LLM 定义查询的一个显著优势是:各种中文方言都被正确地添加到查询中,而无需我在提示中明确定义它们。方便!
讨论
在 GenAI 时代,并非所有搜索用例都应涉及 RAG 和嵌入。使用传统的结构化数据构建搜索引擎非常简单,而且具有现代的纯语言查询便利性。由于其核心实际上只是 prompt 工程,因此这种方法并不是搜索非结构化数据的最佳方法。我确实相信,有大量用例有待探索,其中可以将传统的结构化数据库表暴露给非技术用户进行一般查询。在 LLMs 可用于自动查询生成之前,这是一个困难的问题。
在我看来,这有点错失良机。
准备好自己尝试一下了吗?开始免费试用。
想要获得 Elastic 认证吗?了解下一次 Elasticsearch 工程师培训何时开始!
原文:Write Elastic Query DSL to search structured datasets --- Search Labs