代码地址
https://github.com/LeapLabTHU/ACmix
https://gitee.com/mindspore/models
论文创新点,将注意力机制 和卷积 相结合
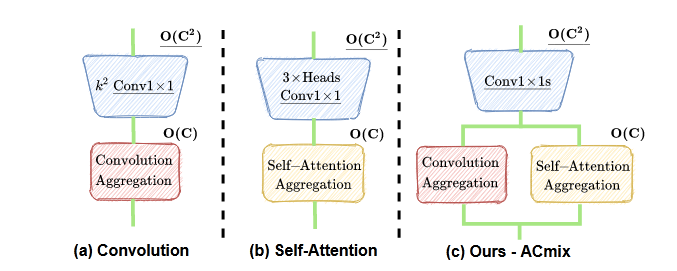
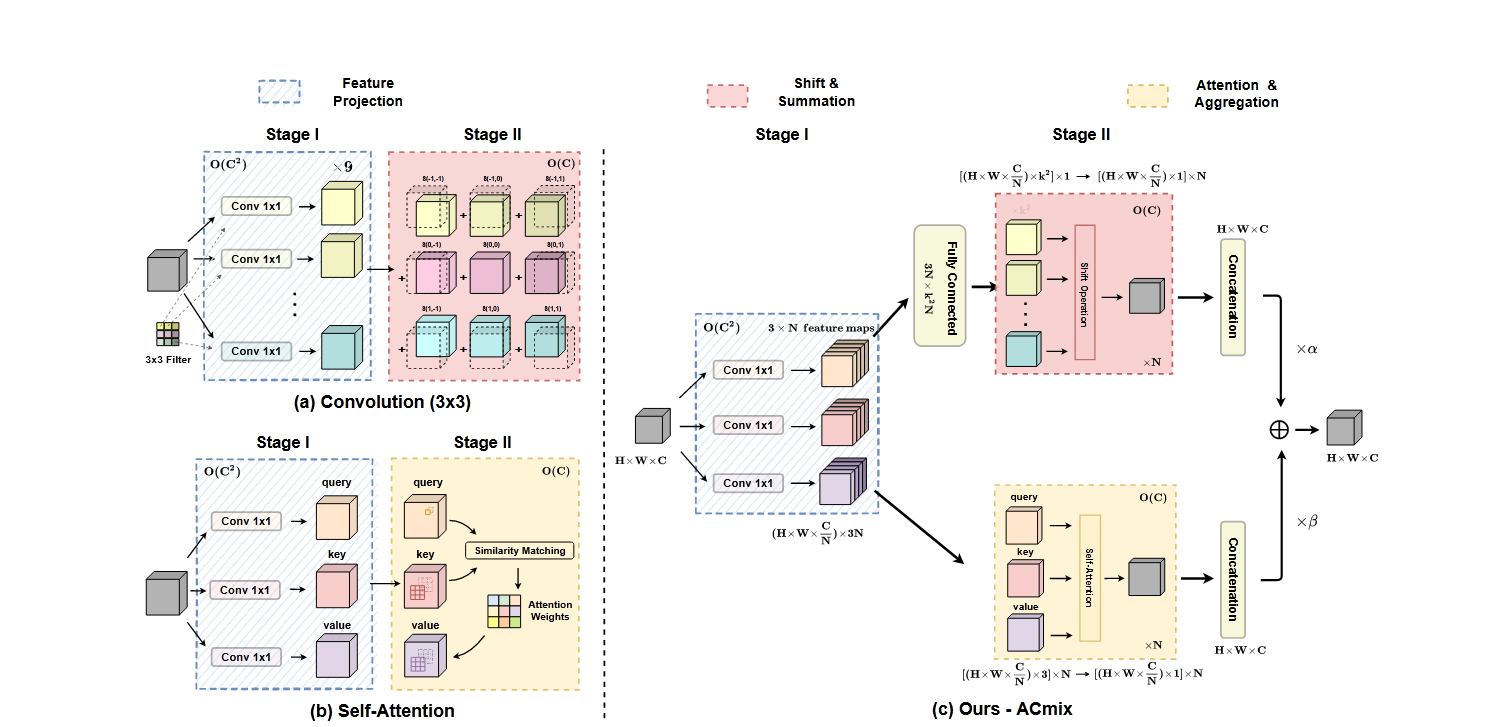
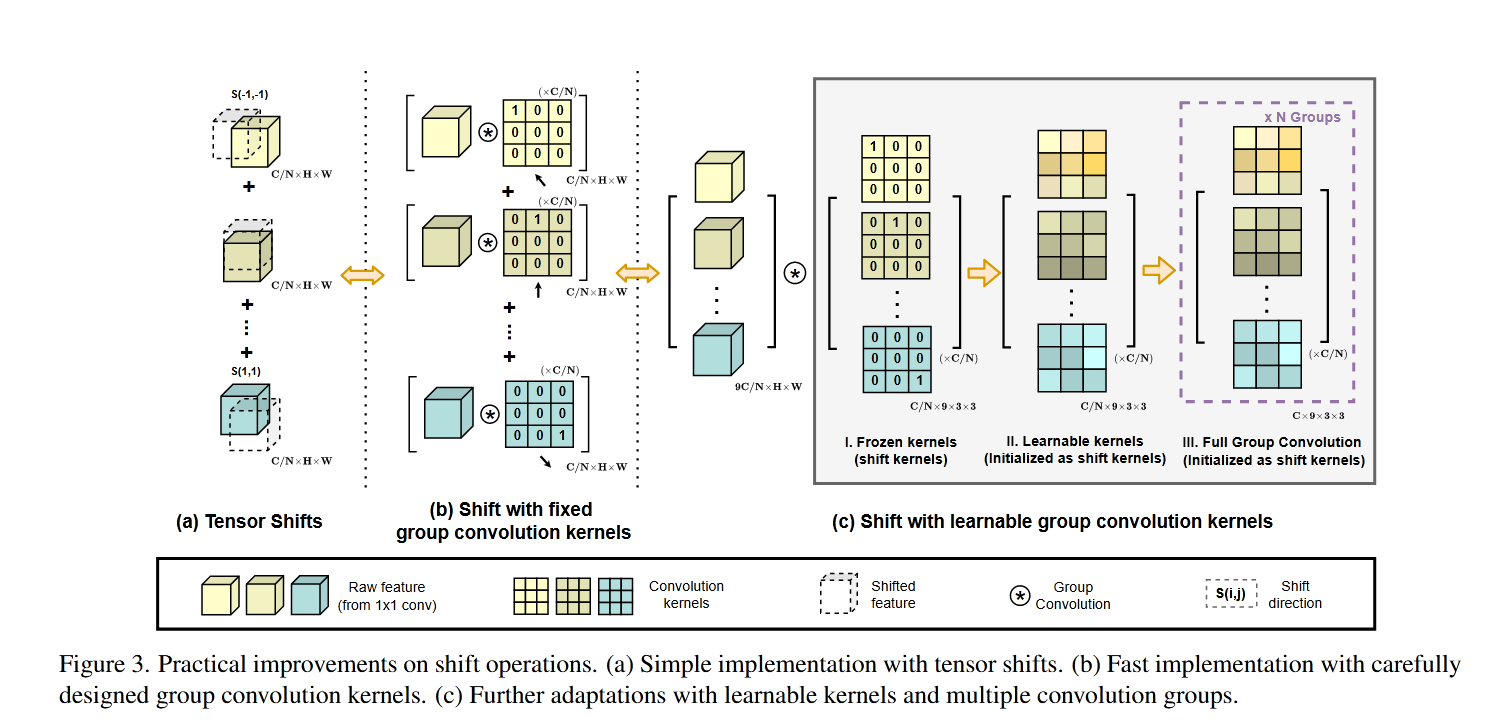
python# encoding: utf-8 ''' @author: duhanyue @start time: 2024/10/13 10:04 ''' import torch import torch.nn as nn def position(H, W, is_cuda=True): if is_cuda: loc_w = torch.linspace(-1.0, 1.0, W).cuda().unsqueeze(0).repeat(H, 1) loc_h = torch.linspace(-1.0, 1.0, H).cuda().unsqueeze(1).repeat(1, W) else: loc_w = torch.linspace(-1.0, 1.0, W).unsqueeze(0).repeat(H, 1) loc_h = torch.linspace(-1.0, 1.0, H).unsqueeze(1).repeat(1, W) loc = torch.cat([loc_w.unsqueeze(0), loc_h.unsqueeze(0)], 0).unsqueeze(0) return loc def stride(x, stride): b, c, h, w = x.shape return x[:, :, ::stride, ::stride] def init_rate_half(tensor): if tensor is not None: tensor.data.fill_(0.5) def init_rate_0(tensor): if tensor is not None: tensor.data.fill_(0.) class ACmix(nn.Module): def __init__(self, in_planes, out_planes, kernel_att=7, head=4, kernel_conv=3, stride=1, dilation=1): super(ACmix, self).__init__() self.in_planes = in_planes self.out_planes = out_planes self.head = head self.kernel_att = kernel_att self.kernel_conv = kernel_conv self.stride = stride self.dilation = dilation self.rate1 = torch.nn.Parameter(torch.Tensor(1)) self.rate2 = torch.nn.Parameter(torch.Tensor(1)) self.head_dim = self.out_planes // self.head self.conv1 = nn.Conv2d(in_planes, out_planes, kernel_size=1) self.conv2 = nn.Conv2d(in_planes, out_planes, kernel_size=1) self.conv3 = nn.Conv2d(in_planes, out_planes, kernel_size=1) self.conv_p = nn.Conv2d(2, self.head_dim, kernel_size=1) self.padding_att = (self.dilation * (self.kernel_att - 1) + 1) // 2 self.pad_att = torch.nn.ReflectionPad2d(self.padding_att) self.unfold = nn.Unfold(kernel_size=self.kernel_att, padding=0, stride=self.stride) self.softmax = torch.nn.Softmax(dim=1) self.fc = nn.Conv2d(3 * self.head, self.kernel_conv * self.kernel_conv, kernel_size=1, bias=False) self.dep_conv = nn.Conv2d(self.kernel_conv * self.kernel_conv * self.head_dim, out_planes, kernel_size=self.kernel_conv, bias=True, groups=self.head_dim, padding=1, stride=stride) self.reset_parameters() def reset_parameters(self): init_rate_half(self.rate1) init_rate_half(self.rate2) kernel = torch.zeros(self.kernel_conv * self.kernel_conv, self.kernel_conv, self.kernel_conv) for i in range(self.kernel_conv * self.kernel_conv): kernel[i, i // self.kernel_conv, i % self.kernel_conv] = 1. kernel = kernel.squeeze(0).repeat(self.out_planes, 1, 1, 1) self.dep_conv.weight = nn.Parameter(data=kernel, requires_grad=True) self.dep_conv.bias = init_rate_0(self.dep_conv.bias) def forward(self, x): q, k, v = self.conv1(x), self.conv2(x), self.conv3(x) scaling = float(self.head_dim) ** -0.5 b, c, h, w = q.shape h_out, w_out = h // self.stride, w // self.stride # ### att # ## positional encoding pe = self.conv_p(position(h, w, x.is_cuda)) q_att = q.view(b * self.head, self.head_dim, h, w) * scaling k_att = k.view(b * self.head, self.head_dim, h, w) v_att = v.view(b * self.head, self.head_dim, h, w) if self.stride > 1: q_att = stride(q_att, self.stride) q_pe = stride(pe, self.stride) else: q_pe = pe unfold_k = self.unfold(self.pad_att(k_att)).view(b * self.head, self.head_dim, self.kernel_att * self.kernel_att, h_out, w_out) # b*head, head_dim, k_att^2, h_out, w_out unfold_rpe = self.unfold(self.pad_att(pe)).view(1, self.head_dim, self.kernel_att * self.kernel_att, h_out, w_out) # 1, head_dim, k_att^2, h_out, w_out att = (q_att.unsqueeze(2) * (unfold_k + q_pe.unsqueeze(2) - unfold_rpe)).sum( 1) # (b*head, head_dim, 1, h_out, w_out) * (b*head, head_dim, k_att^2, h_out, w_out) -> (b*head, k_att^2, h_out, w_out) att = self.softmax(att) out_att = self.unfold(self.pad_att(v_att)).view(b * self.head, self.head_dim, self.kernel_att * self.kernel_att, h_out, w_out) out_att = (att.unsqueeze(1) * out_att).sum(2).view(b, self.out_planes, h_out, w_out) ## conv f_all = self.fc(torch.cat( [q.view(b, self.head, self.head_dim, h * w), k.view(b, self.head, self.head_dim, h * w), v.view(b, self.head, self.head_dim, h * w)], 1)) f_conv = f_all.permute(0, 2, 1, 3).reshape(x.shape[0], -1, x.shape[-2], x.shape[-1]) out_conv = self.dep_conv(f_conv) return self.rate1 * out_att + self.rate2 * out_conv acmix_model = ACmix(in_planes=64,out_planes=64, kernel_att=7, head=4, kernel_conv=3, stride=1, dilation=1) x=torch.randn(16,64,64,44) out=acmix_model(x) x=x+out print(out.shape)