张量
在 PyTorch 中,张量(Tensor)是核心数据结构,类似于 NumPy 中的数组,但具有更强的计算能力和对 GPU 的支持。
创建
从列表或数组创建
py
import torch
# 从列表创建
tensor_from_list = torch.tensor([1, 2, 3, 4])
print(tensor_from_list)
# 从 NumPy 数组创建
import numpy as np
numpy_array = np.array([1, 2, 3, 4])
tensor_from_numpy = torch.from_numpy(numpy_array)
print(tensor_from_numpy)
随机初始化
py
# 创建一个形状为 (2, 3) 的随机张量
random_tensor = torch.rand(2, 3)
print(random_tensor)
# 创建一个形状为 (2, 3) 的标准正态分布随机张量
normal_tensor = torch.randn(2, 3)
print(normal_tensor)
# 创建一个形状为 (2, 3) 的全零张量
zero_tensor = torch.zeros(2, 3)
print(zero_tensor)
# 创建一个形状为 (2, 3) 的全一张量
one_tensor = torch.ones(2, 3)
print(one_tensor)
从其他张量创建
py
# 创建一个与现有张量相同形状和数据类型的张量
tensor_a = torch.tensor([[1, 2], [3, 4]])
tensor_b = torch.zeros_like(tensor_a)
print(tensor_b)
# 创建一个与现有张量相同形状但不同数据类型的张量
tensor_c = torch.ones_like(tensor_a, dtype=torch.float)
print(tensor_c)
基本运算
基本操作
py
# 加法
tensor1 = torch.tensor([1, 2, 3])
tensor2 = torch.tensor([4, 5, 6])
result = tensor1 + tensor2
print(result)
# 减法
result = tensor1 - tensor2
print(result)
# 乘法
result = tensor1 * tensor2
print(result)
# 除法
result = tensor1 / tensor2
print(result)
矩阵操作
py
# 矩阵乘法
matrix1 = torch.tensor([[1, 2], [3, 4]])
matrix2 = torch.tensor([[5, 6], [7, 8]])
result = torch.matmul(matrix1, matrix2)
print(result)
# 点积
vector1 = torch.tensor([1, 2, 3])
vector2 = torch.tensor([4, 5, 6])
result = torch.dot(vector1, vector2)
print(result)
形状操作
py
# 改变形状
tensor = torch.tensor([[1, 2, 3], [4, 5, 6]])
reshaped_tensor = tensor.view(3, 2)
print(reshaped_tensor)
# 展平
flattened_tensor = tensor.flatten()
print(flattened_tensor)
# 增加维度
expanded_tensor = tensor.unsqueeze(0)
print(expanded_tensor)
# 删除维度
squeezed_tensor = expanded_tensor.squeeze(0)
print(squeezed_tensor)
特殊之处
从上面给出的一些简单例子可以看出,张量的创建和一些基本操作方法都和ndarray数组类似,但其也有一些特殊的属性和方法。这些属性和方法都是针对于GPU支持和加速张量计算进行的优化
py
# 查看数据类型
print(tensor.dtype) # 输出 torch.int64
# 查看设备
print(tensor.device) # 输出 cpu
# 显示其梯度信息,方便于计算神经网络的前向传播
print(tensor.grad)
py
# 把张量迁移到GPU上,利用gpu的并行计算加速计算
if torch.cuda.is_available():
device = torch.device("cuda")
tensor_gpu = my_tensor.to(device)
print("-" * 30)
print(tensor_gpu)
print(tensor_gpu.device)
else:
print("CUDA is not available")
上面输出的设备为device='cuda:0'
表示使用第一个可用的 CUDA 设备(通常是显卡)。如果你有多个 GPU,可以通过改变设备编号来选择不同的 GPU。
我们还可以用三元运算符优化一下设备选择代码
py
torch.device("cuda:0" if torch.cuda.is_available() else "cpu")
图像格式转换
在进行计算机视觉任务的时候,有时候需要将pytorch和其余的机器视觉库同时使用,通常是利用opencv等工具先进行图像处理,然后将结果转化成tensor类型传递给pytorch,在pytorch执行之后,也可以将tensor类型的数据转换为numpy等类型用于matplotlib进行可视化展示,此处介绍一下tensor类型和Image类型以及Numpy数组类型的相互转化
我们先使用一张示例图
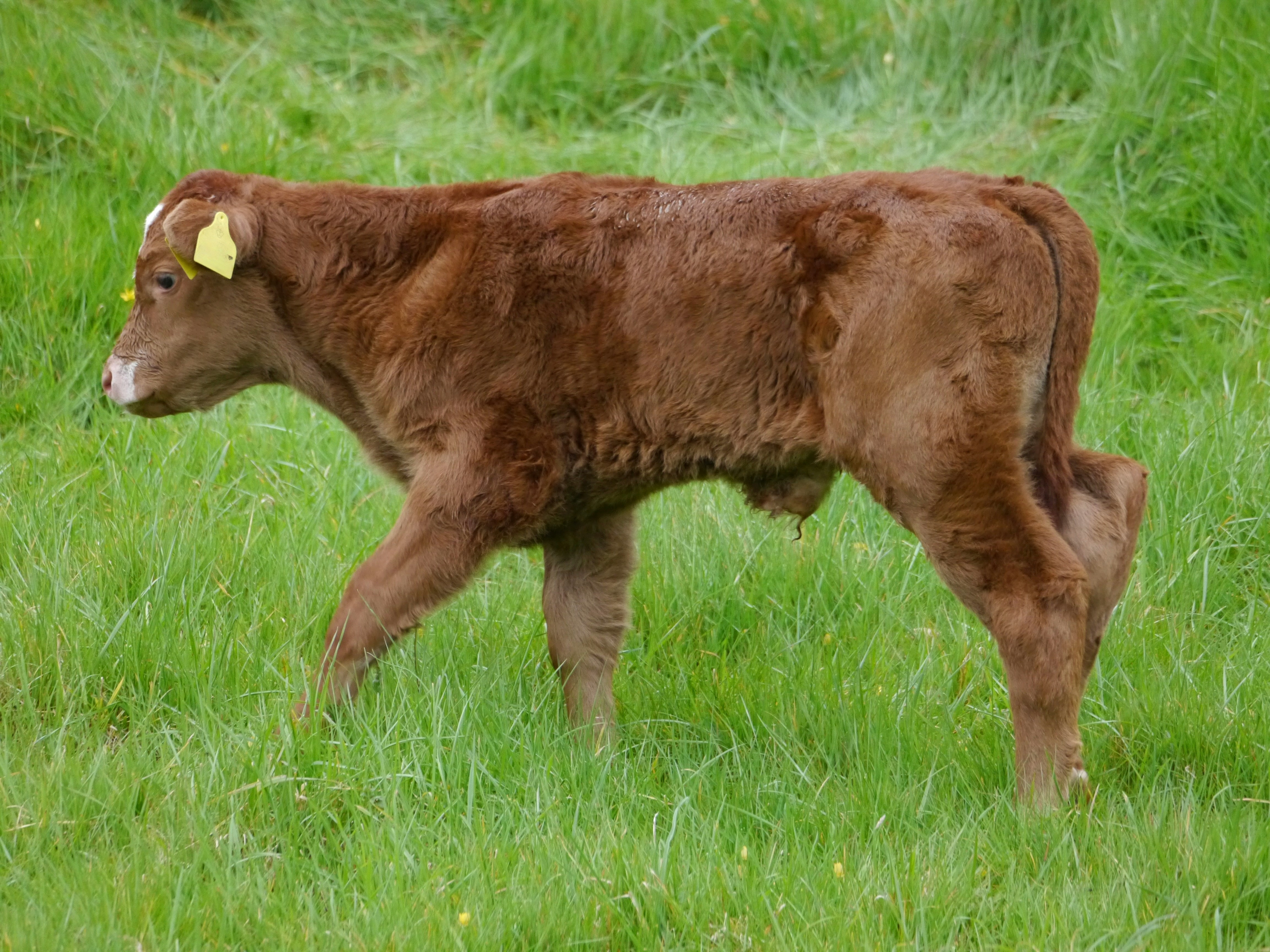
使用opencv读取的图片类型为numpy格式,在虚拟环境中安装opencv的命令为:
shell
pip install opencv-python
接下来读取示例图片并实现其类型的转换
py
# tensor 和 numpy数组的相互转换
import cv2
# opencv读取示例图片
im_cv = cv2.imread('test.jpg')
print("-" * 30)
print(type(im_cv)) # 输出<class 'numpy.ndarray'>
# 因为opencv读取进来的图像按照BGR顺序存储,我们先将其改成RGB模式
im_cv = cv2.cvtColor(im_cv, cv2.COLOR_BGR2RGB)
# 利用torchvision中的transformer模块中的ToTensor类将numpy数组转换成tensor类型
import torchvision
my_totensor = torchvision.transforms.ToTensor()
im_tensor = my_totensor(im_cv)
print(type(im_tensor)) # 输出<class 'torch.Tensor'>
# 为了使用matplotlib,将tensor转换成为numpy数组
import matplotlib.pyplot as plt
im_numpy = im_tensor.numpy()
# 调整维度顺序
im_numpy = np.transpose(im_numpy, (1, 2, 0))
plt.imshow(im_numpy)
plt.axis('off') # 关闭坐标轴
plt.show()
在计算机视觉中,除了opencv读取的numpy格式之外,使用PIL读取的Image类型也十分常用,其也可以和tensor类型进行转换
py
# tensor 和 Image数组的相互转换
from PIL import Image
im_pil = Image.open('test.jpg')
print("-" * 30)
print("PIL读取的图片类型为:",type(im_pil)) # 输出<class 'PIL.JpegImagePlugin.JpegImageFile'>
import torchvision
my_totensor = torchvision.transforms.ToTensor()
im_tensor = my_totensor(im_pil)
print(type(im_tensor)) # 输出<class 'torch.Tensor'>
# 将tensor转换成Image并可视化
my_toPIL = torchvision.transforms.ToPILImage()
im_pil = my_toPIL(im_tensor)
Image._show(im_pil)