在学术研究与数据分析中,数据可视化是呈现研究成果、挖掘数据规律的重要手段。本文将通过 Python 的matplotlib
和seaborn
库,结合实际案例,详细介绍时间序列趋势、分组对比、数据分布、相关矩阵及多变量关系等多种场景下的数据可视化方法,并提供完整可复用代码。无论是论文撰写、报告展示,还是数据探索,都能找到实用的解决方案!大家喜欢就关注一下,代码可以直接运行!
一、可视化基础设置
在进行数据可视化之前,我们需要导入必要的库,并进行一些初始化设置,确保图表风格统一、美观。
import numpy as np
import pandas as pd
import matplotlib.pyplot as plt
import seaborn as sns
from matplotlib.gridspec import GridSpec
# 初始化设置
np.random.seed(42)
plt.style.use('seaborn-v0_8') # 使用seaborn风格
sns.set_context("paper", font_scale=1.1) # 设置适合论文的字体比例
palette = sns.color_palette("Set2") # 定义颜色调色板
这里使用seaborn
的风格和上下文设置,能够让图表在保持专业感的同时,具备良好的可读性。np.random.seed(42)
确保随机数据的可重复性,方便调试和结果复现。
二、生成示例数据集
为了演示不同类型的可视化效果,我们生成五种常见的数据集:时间序列数据、分类数据、分布数据、相关矩阵数据和多维数据。
# 1. 时间序列数据
date_rng = pd.date_range(start='2020-01-01', end='2022-12-31', freq='M')
ts_data = np.cumsum(np.random.normal(0, 1.2, len(date_rng))) + 50
# 2. 分类数据
categories = ['A', 'B', 'C', 'D']
group1 = np.random.randint(20, 50, 4)
group2 = np.random.randint(15, 45, 4)
# 3. 分布数据
dist_data = [np.random.normal(loc=i, scale=1.2, size=100) for i in range(4)]
labels = ['Control', 'T1', 'T2', 'T3']
# 4. 相关矩阵
variables = ['X1', 'X2', 'X3', 'X4', 'X5']
corr_matrix = np.random.uniform(-0.8, 0.8, (5, 5))
np.fill_diagonal(corr_matrix, 1)
# 5. 多维数据
df = pd.DataFrame({
'Var1': np.random.normal(0, 1.5, 200),
'Var2': np.random.exponential(1, 200),
'Category': np.random.choice(['Low', 'Medium', 'High'], 200)
})
这些数据集涵盖了从时间维度变化到变量间关系等多种分析场景,接下来我们将针对每种数据类型进行可视化。
三、多子图布局与可视化实现
我们使用matplotlib
的GridSpec
来创建一个 3 行 2 列的子图布局,并在每个子图中展示不同类型的数据可视化效果。
3.1 子图 1:时间序列趋势
时间序列图常用于展示数据随时间的变化趋势,在学术研究中可用于分析实验指标、经济数据等的动态变化。
# 子图1:时间序列趋势
ax1 = fig.add_subplot(gs[0, 0])
ax1.plot(date_rng, ts_data, color='#2c7bb6', linewidth=2)
ax1.set_title('(a) Time Series Trend', pad=12, fontweight='semibold')
ax1.set_xlabel('Date', labelpad=8)
ax1.set_ylabel('Value', labelpad=8)
ax1.grid(alpha=0.3)
ax1.tick_params(axis='x', rotation=30)
通过plot
函数绘制折线图,并设置颜色、线宽等参数增强可读性。tick_params
调整横坐标标签的旋转角度,避免标签重叠。
3.2 子图 2:分组柱状图
柱状图适用于对比不同类别或组之间的数据差异,常用于实验结果对比、市场份额分析等场景。
# 子图2:分组柱状图
ax2 = fig.add_subplot(gs[0, 1])
x = np.arange(len(categories))
width = 0.35
rects1 = ax2.bar(x - width/2, group1, width, label='Group 1', color=palette[0])
rects2 = ax2.bar(x + width/2, group2, width, label='Group 2', color=palette[1])
ax2.set_title('(b) Group Comparison', pad=12, fontweight='semibold')
ax2.set_xticks(x)
ax2.set_xticklabels(categories)
ax2.legend(frameon=True, framealpha=0.9)
ax2.set_ylabel('Measurement', labelpad=8)
ax2.grid(axis='y', alpha=0.3)
使用bar
函数绘制两组柱状图,并通过设置width
和x
坐标实现分组排列。legend
函数添加图例,帮助区分不同组别。
3.3 子图 3:数据分布
箱线图和抖动图结合能够直观展示数据的分布特征、异常值及组间差异,常用于统计学分析和实验结果评估。
# 子图3:数据分布
ax3 = fig.add_subplot(gs[1, 0])
sns.boxplot(data=dist_data, palette='Set2', width=0.5, ax=ax3)
sns.stripplot(data=dist_data, color='black', alpha=0.5, jitter=0.2, size=4, ax=ax3)
ax3.set_title('(c) Data Distribution', pad=12, fontweight='semibold')
ax3.set_xticks(range(4))
ax3.set_xticklabels(labels)
ax3.set_ylabel('Response Value', labelpad=8)
ax3.grid(axis='y', alpha=0.3)
seaborn
的boxplot
和stripplot
函数组合使用,箱线图展示数据的四分位数和异常值,抖动图则显示数据点的具体分布。
3.4 子图 4:相关矩阵
热力图是展示变量间相关性的有效工具,在数据分析和特征工程中常用于筛选关键变量。
# 子图4:相关矩阵
ax4 = fig.add_subplot(gs[1, 1])
sns.heatmap(corr_matrix, annot=True, cmap='coolwarm', center=0,
fmt=".2f", annot_kws={"size":9}, ax=ax4)
ax4.set_title('(d) Feature Correlations', pad=12, fontweight='semibold')
ax4.set_xticks(np.arange(5)+0.5)
ax4.set_xticklabels(variables, rotation=45, ha='right')
ax4.set_yticks(np.arange(5)+0.5)
ax4.set_yticklabels(variables, rotation=0)
seaborn
的heatmap
函数自动生成热力图,并通过annot=True
添加数值标注,cmap='coolwarm'
使用冷暖色调区分正负相关性。
3.5 子图 5:多变量关系
散点图结合颜色编码能够展示多维数据中变量间的关系和类别分布,常用于探索性数据分析。
# 子图5:多变量关系
ax5 = fig.add_subplot(gs[2, :]) # 跨两列
sc = ax5.scatter(df['Var1'], df['Var2'], c=df['Category'].map({'Low':0, 'Medium':1, 'High':2}),
cmap='viridis', alpha=0.8, s=50, edgecolor='w', linewidth=0.5)
ax5.set_title('(e) Multivariate Relationship', pad=12, fontweight='semibold')
ax5.set_xlabel('Variable 1', labelpad=8)
ax5.set_ylabel('Variable 2', labelpad=8)
cbar = fig.colorbar(sc, ax=ax5, ticks=[0, 1, 2])
cbar.set_ticklabels(['Low', 'Medium', 'High'])
cbar.set_label('Category', rotation=270, labelpad=15)
ax5.grid(alpha=0.3)
scatter
函数绘制散点图,并通过c
参数根据类别设置颜色。colorbar
添加图例,清晰展示颜色与类别的对应关系。
四、全局调整与图表展示
最后,我们添加全局标题,并使用tight_layout
优化子图布局,确保图表美观且信息完整。
# 全局调整
plt.suptitle("Comprehensive Data Visualization for Academic Research",
y=0.99, fontsize=14, fontweight='bold')
plt.tight_layout()
plt.show(block=True)
五、可视化结果展示
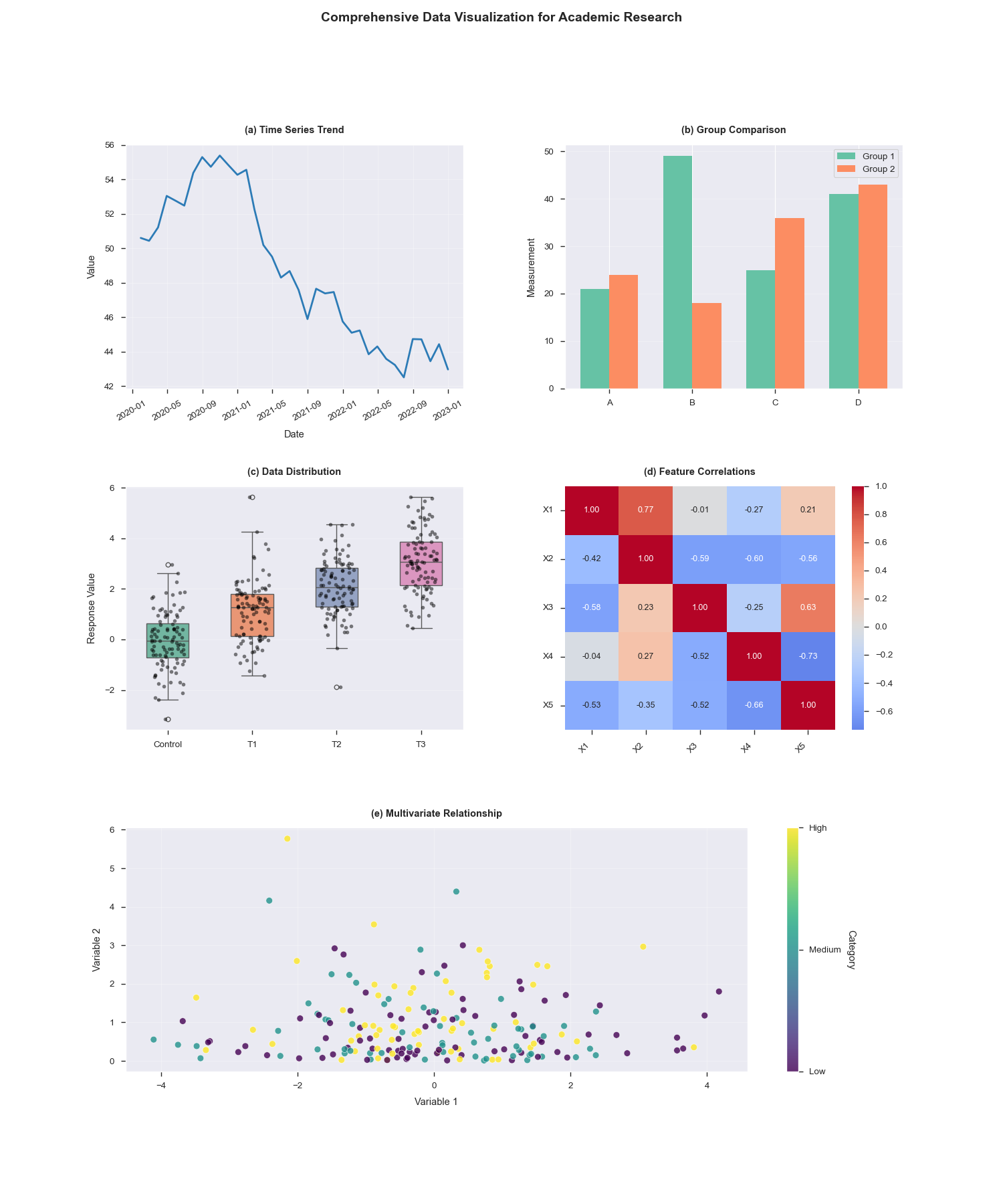