系列文章目录
- 【扩散模型(一)】中介绍了 Stable Diffusion 可以被理解为重建分支(reconstruction branch)和条件分支(condition branch)
- 【扩散模型(二)】IP-Adapter 从条件分支的视角,快速理解相关的可控生成研究
- 【扩散模型(三)】IP-Adapter 源码详解1-训练输入 介绍了训练代码中的 image prompt 的输入部分,即 img projection 模块。
- 【扩散模型(四)】IP-Adapter 源码详解2-训练核心(cross-attention)详细介绍 IP-Adapter 训练代码的核心部分,即插入 Unet 中的、针对 Image prompt 的 cross-attention 模块。
- 【扩散模型(五)】IP-Adapter 源码详解3-推理代码 详细介绍 IP-Adapter 推理过程代码。
- 【可控图像生成系列论文(四)】IP-Adapter 具体是如何训练的?1公式篇
- 【扩散模型(六)】IP-Adapter 是如何训练的?2 源码篇(IP-Adapter Plus)
- 【扩散模型(九)】IP-Adapter 与 IP-Adapter Plus 的具体区别是什么?
文章目录
通过前面的系列文章,很清楚要训练的就是 image_proj_model(或者对于 plus 来说是 resampler) 和 adapter_modules 两块。
而 image_proj_model 这块比较简单,原码如下所示
python
# freeze parameters of models to save more memory
unet.requires_grad_(False)
vae.requires_grad_(False)
text_encoder.requires_grad_(False)
image_encoder.requires_grad_(False)
#ip-adapter
image_proj_model = ImageProjModel(
cross_attention_dim=unet.config.cross_attention_dim,
clip_embeddings_dim=image_encoder.config.projection_dim,
clip_extra_context_tokens=4,
)
adapter_modules 分为两类
- AttnProcessor 对应 self attention
- IPAttnProcessor 对应 cross attention
按理说 self attention 对应的 AttnProcessor 应该不会被训练,但是 training = True,便让人非常费解。
进一步查看 AttnProcessor2_0 和 IPAttnProcessor2_0 后,就清楚了,因为从 AttnProcessor2_0 的构造函数(init)中并没有参数,就算是 trianing = True 也并不影响训练,实际训练的模块还是 IPAttnProcessor2_0 构造函数中的 to_k_ip 和 to_v_ip 两层 linear!
python
class AttnProcessor2_0(torch.nn.Module):
r"""
Processor for implementing scaled dot-product attention (enabled by default if you're using PyTorch 2.0).
"""
def __init__(
self,
hidden_size=None,
cross_attention_dim=None,
):
super().__init__()
if not hasattr(F, "scaled_dot_product_attention"):
raise ImportError("AttnProcessor2_0 requires PyTorch 2.0, to use it, please upgrade PyTorch to 2.0.")
def __call__(
...
class IPAttnProcessor2_0(torch.nn.Module):
r"""
Attention processor for IP-Adapater for PyTorch 2.0.
Args:
hidden_size (`int`):
The hidden size of the attention layer.
cross_attention_dim (`int`):
The number of channels in the `encoder_hidden_states`.
scale (`float`, defaults to 1.0):
the weight scale of image prompt.
num_tokens (`int`, defaults to 4 when do ip_adapter_plus it should be 16):
The context length of the image features.
"""
def __init__(self, hidden_size, cross_attention_dim=None, scale=1.0, num_tokens=4):
super().__init__()
if not hasattr(F, "scaled_dot_product_attention"):
raise ImportError("AttnProcessor2_0 requires PyTorch 2.0, to use it, please upgrade PyTorch to 2.0.")
self.hidden_size = hidden_size
self.cross_attention_dim = cross_attention_dim
self.scale = scale
self.num_tokens = num_tokens
self.to_k_ip = nn.Linear(cross_attention_dim or hidden_size, hidden_size, bias=False)
self.to_v_ip = nn.Linear(cross_attention_dim or hidden_size, hidden_size, bias=False)
def __call__(
总结
- IP-Adapter 训的就是 image_proj_model(或者对于 plus 来说是 resampler) 和 adapter_modules 两块。
- 在 adapter_modules 中,实际只训了 IPAttnProcessor2_0 的 to_k_ip 和 to_v_ip。
- adapter_modules 是在每个有含有 cross attention 的 unet block 里进行的替换,如下图所示。
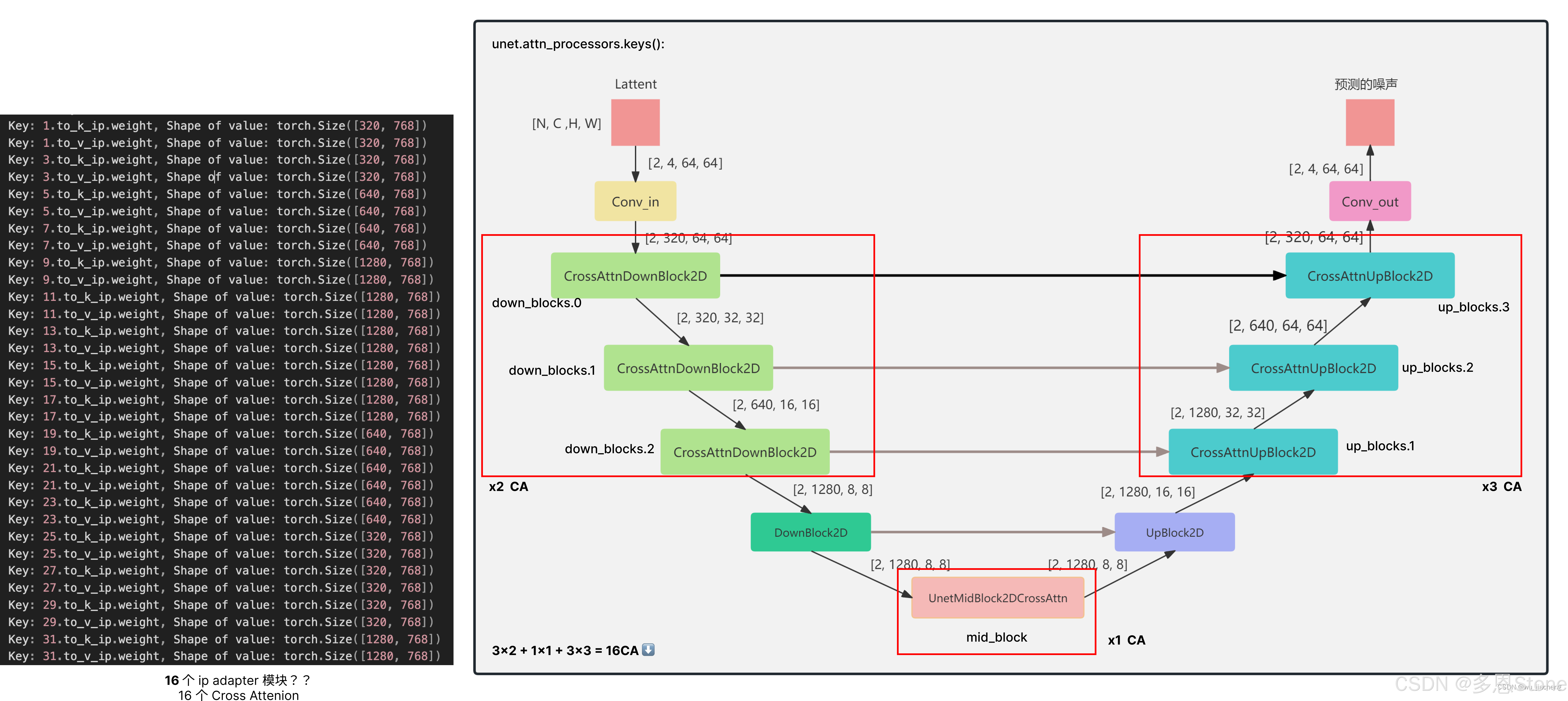