目录
哈希的概念
哈希又称散列,是一种组织数据的方式
它的本质是通过哈希函数把关键字key跟存储位置建立一个映射关系,查找时再通过这个哈希函数计算出key存储的位置,进行快速查找
所以它的查找时间复杂度能达到恐怖的O(1)
直接定址法
直接定址法可以是一个26个大小的数组arr[26]来代表26个英文字母,这时候这26个空间就和这26个英文字母建立了映射关系
cpp
class Solution {
public:
int firstUniqChar(string s) {
int hash[26];
for (auto str : s)
hash[str - 'a']++;
for (int i = 0; i < s.size(); i++)
if (hash[s[i] - 'a'] == 1)
return i;
return -1;
}
};
哈希冲突
当我们使用直接定址法的时候,当数据映射到同一个位置的时候就把它叫做哈希冲突或者哈希碰撞
哈希冲突是不可避免的
为了减少哈希冲突,我们可以使用一个比较好的哈希函数来减少哈希冲突
负载因子
若哈希表的大小为M,已经映射存储的数据个数为N,那么 负载因子 = N / M
负载因子越大,哈希冲突的概率越高,空间利用率越高
负载因子越小,哈希冲突的概率越低,空间利用率越低
哈希函数
除法散列法/除留余数法
假设哈希表的大小为M
那么通过key除以M的余数作为映射位置的下标
哈希函数为:hashi = key % M
乘法散列法
乘法散列法对哈希表的大小M没有要求
第一步:用关键字key乘上常数A(0 < A < 1),并抽取key*A的小数部分
第二部:后再用M乘以key*A的小数部分,再向下取整
哈希函数为:hashi = floor(M * ((A * key) % 1.0))
这里最重要的是A的值如何设定
Knuth认为A = 0.6180339887......(黄金分割点)比较好
处理哈希冲突
主要有两种方法,开放定址法和链地址法
开放定址法
线性探测
从发生冲突的位置开始,依次线性向后探测,直到寻找到下一个没有存储数据的位置为止
如果走到哈希表尾,则回绕到哈希表头的位置
h(key) = hash0 = key % M,若hash0冲突,则线性探测公式为
hc(key, i) = hashi = (hash0 + i) % M, i = {1, 2, 3..., M - 1}(负载因子小于1,最多探测M-1次,一定能找到一个位置存储)
二次探测
从发生冲突的位置开始,依次左右按二次方跳跃式探测,直到寻找到下一个没有存储数据的位置为止,如果从右走到哈希表尾,则回绕到哈希表头的位置,如果往左走到哈希表头,则回绕到哈希表尾的位置
h(key) = hash0 = key % M,若hash0冲突,则二次线性探测公式为
hc(key, i) = hashi = (hash0 +/- i^2) % M, i = {1, 2, 3, ....., M / 2}
当hashi < 0时,需要hash += M
双重散列
当第一个哈希函数计算出的值发生冲突,使用第二个哈希函数计算出一个跟key相关的偏移量值,不断往后探测,直到寻找到下一个没有数据的位置为止
h1(key) = hash0 = key % M,hash0位置冲突了,则双重探测公式为
hc(key, i) = hashi = (hash0 + i * h2(key)) % M,i = {1, 2, 3, ....., M}
链地址法
开放定址法中所有的元素都放到哈希表里,链地址法中所有的数据不再直接存储在哈希表中
哈希表里只需要存储一个指针,当没有数据映射这个位置时,指针为空,当有多个数据映射这个位置时,我们把这些冲突的数据链接成一个链表,挂在哈希表当前位置的下面
链地址法也叫做拉链法或者哈希桶
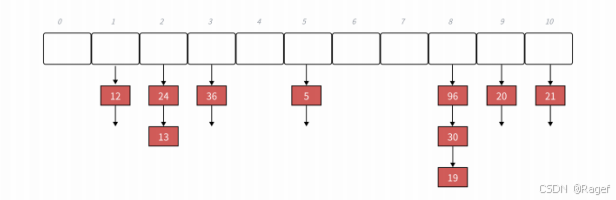
哈希表的实现
哈希表的结构
cpp
enum State
{
EXIST,
EMPTY,
DELETE
};
template<class K, class V>
struct HashData
{
pair<K, V> _kv;
State _state = EMPTY;
};
因为我们删除一个值后无法判断这个值是存在还是删除,所以我们可以用一个State状态来标记当前位置的是一个什么状态,所以我们需要枚举出三个状态区分
cpp
template<class K>
struct HashFunc
{
size_t operator()(const K& key)
{
return (size_t)key;
}
};
template<>
struct HashFunc<string>
{
size_t operator()(const string& s)
{
// BKDR
size_t hash = 0;
for (auto ch : s)
{
hash += ch;
hash *= 131;
}
return hash;
}
};
因为我们映射的key不能确定是什么类型,若是char、int这类整型,可以强转成size_t当作key
但是如果是string这类无法转换成数字的类型,我们就需要自己写一个仿函数来拿出一个整数key
这里用的是BKDR算法来进行转换的key
cpp
inline unsigned long __stl_next_prime(unsigned long n)
{
// Note: assumes long is at least 32 bits.
static const int __stl_num_primes = 28;
static const unsigned long __stl_prime_list[__stl_num_primes] = {
53, 97, 193, 389, 769,
1543, 3079, 6151, 12289, 24593,
49157, 98317, 196613, 393241, 786433,
1572869, 3145739, 6291469, 12582917, 25165843,
50331653, 100663319, 201326611, 402653189, 805306457,
1610612741, 3221225473, 4294967291
};
const unsigned long* first = __stl_prime_list;
const unsigned long* last = __stl_prime_list + __stl_num_primes;
const unsigned long* pos = lower_bound(first, last, n);
return pos == last ? *(last - 1) : *pos;
}
这是扩容的逻辑,因为哈希表为了减少哈希冲突,哈希表的容量需要尽可能的是素数,所以这里写了一个函数列了一个素数表来拿到下一个接近2倍并且是素数的值
lower_bound函数前两个参数是一个迭代器区间,第三个参数是一个值value
该函数会在给定区间内给出一个不小于value的值
这样在扩容的时候就能按接近2倍扩容并且容量还是素数,减少了哈希冲突的可能性
闭散列(开放定址法)
结构
cpp
template<class K, class V, class Hash = HashFunc<K>>
class HashTable
{
public:
HashTable()
:_tables(__stl_next_prime(0))
, _n(0)
{}
private:
vector<HashData<K, V>> _tables;
size_t _n;
};
第一个模板参数表示key,第二个模板参数表示value,第三个模板参数是将key转换成整数的类,里面会有仿函数
_tables表的容量默认是素数表中大于0的数也就是第一个53
_n表示当前表的大小为0
插入
cpp
bool Insert(const pair<K, V>& kv)
{
if (Find(kv.first))
return false;
// 负载因子大于0.7则扩容
if (_n * 10 / _tables.size() >= 7)
{
HashTable<K, V> newht;
newht._tables.resize(__stl_next_prime(_tables.size() + 1));
for (auto& data : _tables)
{
if (data._state == EXIST)
{
newht.Insert(data._kv);
}
}
_tables.swap(newht._tables);
}
Hash hash;
size_t hash0 = hash(kv.first) % _tables.size();
size_t hashi = hash0;
size_t i = 1;
while (_tables[hashi]._state == EXIST)
{
hashi = (hash0 + i) % _tables.size();
i++;
}
_tables[hashi]._kv = kv;
_tables[hashi]._state = EXIST;
_n++;
return true;
}
首先用Find函数判断该值的key是否存在哈希表内(该哈希表不存在值冗余)
Find函数下面实现
负载因子若是太小会浪费空间,若是太大容易造成哈希冲突,所以这里规定若是负载因子>=0.7则扩容
首先创建一个新的哈希表,并且提前将它的容量扩容到当前表大小的后一个素数表中的值
然后再遍历旧表将每个EXIST存在的值插入到新表中,最后两个表交换即可
首先用Hash类创建出hash对象,从而能够取出key中代表的那个的整数
用这个整数模上表大小即可得到映射关系的位置
若当前位置已经存在值,则表示哈希冲突,那么可以使用线性探测来一个个走
最后插入当前位置即可
查找
cpp
HashData<K, V>* Find(const K& key)
{
Hash hash;
size_t hash0 = hash(key) % _tables.size();
size_t hashi = hash0;
size_t i = 1;
while (_tables[hashi]._state != EMPTY)
{
if (_tables[hashi]._state == EXIST && _tables[hashi]._kv.first == key)
{
return &_tables[hashi];
}
hashi = (hash0 + i) % _tables.size();
i++;
}
return nullptr;
}
先用hash对象取出key对应的整数,求出映射关系,若当前位置有值则根据上面的线性探测规则一个个往后找即可
删除
cpp
bool Erase(const K& key)
{
HashData<K, V>* ret = Find(key);
if (ret)
{
ret->_state = DELETE;
return true;
}
else
{
return false;
}
}
删除只需要找到位置之后把状态state置为DELETE即可
开散列(链地址法)
结构
cpp
template<class K, class V>
struct HashNode
{
pair<K, V> _kv;
HashNode<K, V>* _next;
HashNode(const pair<K, V>& kv)
:_kv(kv)
, _next(nullptr)
{}
};
template<class K, class V, class Hash = HashFunc<K>>
class HashTable
{
typedef HashNode<K, V> Node;
public:
HashTable()
:_tables(__stl_next_prime(0))
, _n(0)
{}
private:
vector<Node*> _tables; // 指针数组
size_t _n = 0;
};
开散列需要Hash节点,该节点需要有值value和一个next指针,这样就能构成一个链表
下面的结构和上面的闭散列一致
开散列需要写拷贝构造、赋值重载、析构函数,这些节点的值需要我们手动释放
插入
cpp
bool Insert(const pair<K, V>& kv)
{
if (Find(kv.first))
return false;
Hash hash;
// 负载因子为1时扩容
if (_n == _tables.size())
{
vector<Node*> newTable(__stl_next_prime(_tables.size() + 1));
for (size_t i = 0; i < _tables.size(); i++)
{
Node* cur = _tables[i];
while (cur)
{
Node* next = cur->_next;
// 头插到新表
size_t hashi = hash(cur->_kv.first) % newTable.size();
cur->_next = newTable[hashi];
newTable[hashi] = cur;
cur = next;
}
_tables[i] = nullptr;
}
_tables.swap(newTable);
}
size_t hashi = hash(kv.first) % _tables.size();
Node* newnode = new Node(kv);
newnode->_next = _tables[hashi];
_tables[hashi] = newnode;
_n++;
return true;
}
这里的负载因子只需要到1再扩容即可,因为这里处理哈希冲突是用链表接在下面的,不会计入负载因子的分子中
这里的扩容逻辑几乎和上面闭散列的一致,区别就是插入数据的时候这里是需要用链表的方式头插
下面的插入逻辑也是将新值头插到映射关系的位置上即可
查找
cpp
Node* Find(const K& key)
{
Hash hash;
size_t hashi = hash(key) % _tables.size();
Node* cur = _tables[hashi];
while (cur)
{
if (cur->_kv.first == key)
{
return cur;
}
cur = cur->_next;
}
return nullptr;
}
先找到映射关系的位置,再沿着链表一个个查找即可
删除
cpp
bool Erase(const K& key)
{
size_t hashi = key % _tables.size();
Node* prev = nullptr;
Node* cur = _tables[hashi];
while (cur)
{
if (cur->_kv.first == key)
{
if (prev == nullptr)
{
// 头结点
_tables[hashi] = cur->_next;
}
else
{
// 中间节点
prev->_next = cur->_next;
}
delete cur;
--_n;
return true;
}
else
{
prev = cur;
cur = cur->_next;
}
}
return false;
}
先找到映射关系的位置,再沿着链表一个个查找
若找到删除的值,则可以根据prev是否为空来判断删除的节点是否是头节点
完整代码
cpp
#pragma once
#include <iostream>
#include <vector>
#include <string>
using namespace std;
enum State
{
EXIST,
EMPTY,
DELETE
};
template<class K, class V>
struct HashData
{
pair<K, V> _kv;
State _state = EMPTY;
};
template<class K>
struct HashFunc
{
size_t operator()(const K& key)
{
return (size_t)key;
}
};
template<>
struct HashFunc<string>
{
size_t operator()(const string& s)
{
// BKDR
size_t hash = 0;
for (auto ch : s)
{
hash += ch;
hash *= 131;
}
return hash;
}
};
inline unsigned long __stl_next_prime(unsigned long n)
{
// Note: assumes long is at least 32 bits.
static const int __stl_num_primes = 28;
static const unsigned long __stl_prime_list[__stl_num_primes] = {
53, 97, 193, 389, 769,
1543, 3079, 6151, 12289, 24593,
49157, 98317, 196613, 393241, 786433,
1572869, 3145739, 6291469, 12582917, 25165843,
50331653, 100663319, 201326611, 402653189, 805306457,
1610612741, 3221225473, 4294967291
};
const unsigned long* first = __stl_prime_list;
const unsigned long* last = __stl_prime_list + __stl_num_primes;
const unsigned long* pos = lower_bound(first, last, n);
return pos == last ? *(last - 1) : *pos;
}
namespace open_address
{
template<class K, class V, class Hash = HashFunc<K>>
class HashTable
{
public:
HashTable()
:_tables(__stl_next_prime(0))
, _n(0)
{}
bool Insert(const pair<K, V>& kv)
{
if (Find(kv.first))
return false;
// 负载因子大于0.7则扩容
if (_n * 10 / _tables.size() >= 7)
{
HashTable<K, V> newht;
newht._tables.resize(__stl_next_prime(_tables.size() + 1));
for (auto& data : _tables)
{
if (data._state == EXIST)
{
newht.Insert(data._kv);
}
}
_tables.swap(newht._tables);
}
Hash hash;
size_t hash0 = hash(kv.first) % _tables.size();
size_t hashi = hash0;
size_t i = 1;
while (_tables[hashi]._state == EXIST)
{
hashi = (hash0 + i) % _tables.size();
i++;
}
_tables[hashi]._kv = kv;
_tables[hashi]._state = EXIST;
_n++;
return true;
}
HashData<K, V>* Find(const K& key)
{
Hash hash;
size_t hash0 = hash(key) % _tables.size();
size_t hashi = hash0;
size_t i = 1;
while (_tables[hashi]._state != EMPTY)
{
if (_tables[hashi]._state == EXIST && _tables[hashi]._kv.first == key)
{
return &_tables[hashi];
}
hashi = (hash0 + i) % _tables.size();
i++;
}
return nullptr;
}
bool Erase(const K& key)
{
HashData<K, V>* ret = Find(key);
if (ret)
{
ret->_state = DELETE;
return true;
}
else
{
return false;
}
}
private:
vector<HashData<K, V>> _tables;
size_t _n;
};
}
namespace hash_bucket
{
template<class K, class V>
struct HashNode
{
pair<K, V> _kv;
HashNode<K, V>* _next;
HashNode(const pair<K, V>& kv)
:_kv(kv)
, _next(nullptr)
{}
};
template<class K, class V, class Hash = HashFunc<K>>
class HashTable
{
typedef HashNode<K, V> Node;
public:
HashTable()
:_tables(__stl_next_prime(0))
, _n(0)
{}
~HashTable()
{
for (size_t i = 0; i < _tables.size(); i++)
{
Node* cur = _tables[i];
while (cur)
{
Node* next = cur->_next;
delete cur;
cur = next;
}
_tables[i] = nullptr;
}
}
bool Insert(const pair<K, V>& kv)
{
if (Find(kv.first))
return false;
Hash hash;
// 负载因子为1时扩容
if (_n == _tables.size())
{
vector<Node*> newTable(__stl_next_prime(_tables.size() + 1));
for (size_t i = 0; i < _tables.size(); i++)
{
Node* cur = _tables[i];
while (cur)
{
Node* next = cur->_next;
// 头插到新表
size_t hashi = hash(cur->_kv.first) % newTable.size();
cur->_next = newTable[hashi];
newTable[hashi] = cur;
cur = next;
}
_tables[i] = nullptr;
}
_tables.swap(newTable);
}
size_t hashi = hash(kv.first) % _tables.size();
Node* newnode = new Node(kv);
newnode->_next = _tables[hashi];
_tables[hashi] = newnode;
_n++;
return true;
}
Node* Find(const K& key)
{
Hash hash;
size_t hashi = hash(key) % _tables.size();
Node* cur = _tables[hashi];
while (cur)
{
if (cur->_kv.first == key)
{
return cur;
}
cur = cur->_next;
}
return nullptr;
}
bool Erase(const K& key)
{
size_t hashi = key % _tables.size();
Node* prev = nullptr;
Node* cur = _tables[hashi];
while (cur)
{
if (cur->_kv.first == key)
{
if (prev == nullptr)
{
// 头结点
_tables[hashi] = cur->_next;
}
else
{
// 中间节点
prev->_next = cur->_next;
}
delete cur;
--_n;
return true;
}
else
{
prev = cur;
cur = cur->_next;
}
}
return false;
}
private:
vector<Node*> _tables; // 指针数组
size_t _n = 0;
};
}
完