目录
[0 日志数据集介绍](#0 日志数据集介绍)
[1 构建数据仓库](#1 构建数据仓库)
[1.1 ods创建用户打车订单表](#1.1 ods创建用户打车订单表)
[1.2 创建分区](#1.2 创建分区)
[1.3 上传到对应分区](#1.3 上传到对应分区)
[1.4 数据预处理](#1.4 数据预处理)
[2 订单分析](#2 订单分析)
[2.1 app层建表](#2.1 app层建表)
[2.2 加载数据到app表](#2.2 加载数据到app表)
[2.3 不同地域订单占比分析(省份)](#2.3 不同地域订单占比分析(省份))
0 日志数据集介绍
以下就是一部门用户打车的日志文件。
b05b0034cba34ad4a707b4e67f681c71,15152042581,109.348825,36.068516, 陕 西 省 , 延 安 市,78.2,男,软件工程,70后,4,1,2020-4-12 20:54,0,2020-4-12 20:06
23b60a8ff11342fcadab3a397356ba33,15152049352,110.231895,36.426178, 陕 西 省 , 延 安 市,19.5,女,金融,80后,3,0,0,2020-4-12 4:04
1db33366c0e84f248ade1efba0bb9227,13905224124,115.23596,38.652724, 河 北 省 , 保 定 市,13.7,男,金融,90后,7,1,2020-4-12 10:10,0,2020-4-12 0:29
日志包含了以下字段:
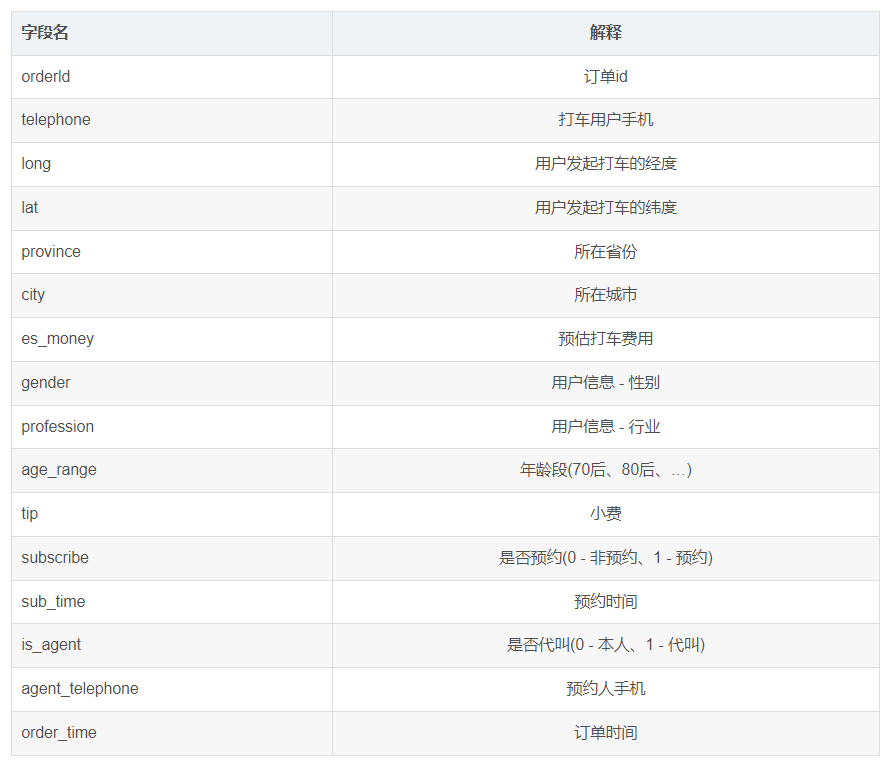
1 构建数据仓库
为了方便组织、管理上述的三类数据,我们将数仓分成不同的层,简单来说,就是分别将三类不同 的数据保存在Hive的不同数据库中。
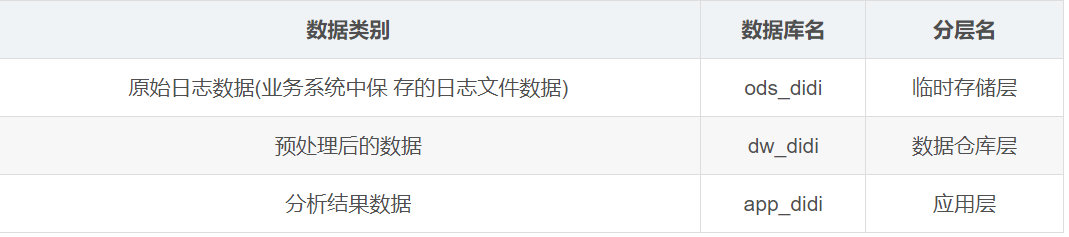
sql
-- 1.1 创建ods库
create database if not exists ods_didi;
-- 1.2 创建dw库
create database if not exists dw_didi;
-- 1.3 创建app库
create database if not exists app_didi;
1.1 ods创建用户打车订单表
sql
-- 2.1 创建订单表结构
create table if not exists ods_didi.t_user_order(
orderId string comment '订单id',
telephone string comment '打车用户手机',
lng string comment '用户发起打车的经度',
lat string comment '用户发起打车的纬度',
province string comment '所在省份',
city string comment '所在城市',
es_money double comment '预估打车费用',
gender string comment '用户信息 - 性别',
profession string comment '用户信息 - 行业',
age_range string comment '年龄段(70后、80后、...)',
tip double comment '小费',
subscribe integer comment '是否预约(0 - 非预约、1 - 预约)',
sub_time string comment '预约时间',
is_agent integer comment '是否代叫(0 - 本人、1 - 代叫)',
agent_telephone string comment '预约人手机',
order_time string comment '预约时间'
)
partitioned by (dt string comment '时间分区')
ROW FORMAT DELIMITED FIELDS TERMINATED BY ',' ;
1.2 创建分区
sql
alter table ods_didi.t_user_order add if not exists partition(dt='2020-04-12');
1.3 上传到对应分区
sql
-- 上传订单数据
hadoop fs -put /root/data/didi/order.csv /user/hive/warehouse/ods_didi.db/t_user _order/dt=2020-04-12
-- 查看文件
hadoop fs -ls /user/hive/warehouse/ods_didi.db/t_user_order/dt=2020-04-12
-- 查看是否映射成功
select * from ods_didi.t_user_order limit 10
1.4 数据预处理
目的主要是让预处理后的数据更容易进行数据分析,并且能够将一些非法 的数据处理掉,避免影响实际的统计结果
dw层创建宽表:
sql
create table if not exists dw_didi.t_user_order_wide(
orderId string comment '订单id',
telephone string comment '打车用户手机',
lng string comment '用户发起打车的经度',
lat string comment '用户发起打车的纬度',
province string comment '所在省份',
city string comment '所在城市',
es_money double comment '预估打车费用',
gender string comment '用户信息',
profession string comment '用户信息',
age_range string comment '年龄段(70后、80后、',
tip double comment '小费',
subscribe integer comment '是否预约(0 - 非预约、1 - 预约)',
subscribe_name string comment '是否预约名称',
sub_time string comment '预约时间',
is_agent integer comment '是否代叫(0 - 本人、1 - 代叫)',
is_agent_name string comment '是否代叫名称',
agent_telephone string comment '预约人手机',
order_date string comment '预约日期,yyyy-MM-dd',
order_year integer comment '年',
order_month integer comment '月',
order_day integer comment '日',
order_hour integer comment '小时',
order_time_range string comment '时间段',
order_time string comment '预约时间'
)
partitioned by (dt string comment '按照年月日来分区')
row format delimited fields terminated by ',';
预处理需求
- 过滤掉order_time长度小于8的数据,如果小于8,表示这条数据不合法,不应该参加统计。
- 将一些0、1表示的字段,处理为更容易理解的字段。例如:subscribe字段,0表示非预约、1表 示预约。我们需要添加一个额外的字段,用来展示非预约和预约,这样将来我们分析的时候,跟容 易看懂数据。
- order_time字段为2020-4-12 1:15,为了将来更方便处理,我们统一使用类似 2020-04-12 01:15 来表示,这样所有的order_time字段长度是一样的。并且将日期获取出来
- 为了方便将来按照年、月、日、小时统计,我们需要新增这几个字段。
- 后续要分析一天内,不同时段的订单量,我们需要在预处理过程中将订单对应的时间段提前计 算出来
数据加载到dw层宽表
HQL编写好后,为了方便后续分析,我们需要将预处理好的数据写入到之前创建的宽表中。注意: 宽表也是一个分区表,所以,写入的时候一定要指定对应的分区。
sql
insert overwrite table dw_didi.t_user_order_wide partition(dt = '2020-04-12')
select
orderId,
telephone,
long,
lat,
province,
city,
es_money,
gender,
profession,
age_range,
tip,
subscribe,
case when subscribe = 0 then '非预约'
when subscribe = 1 then '预约'
end as subscribe_name,
sub_time,
is_agent,
case when is_agent = 0 then '本人'
when is_agent = 1 then '代叫'
end as is_agent_name,
agent_telephone,
date_format(order_time, 'yyyy-MM-dd') as order_date,
year(order_time) as year,
month(order_time) as month,
day(order_time) as day,
hour(order_time) as hour,
case when hour(order_time) >= 1 and hour(order_time) < 5 then '凌晨'
when hour(order_time) >= 5 and hour(order_time) < 8 then '早上'
when hour(order_time) >= 8 and hour(order_time) < 11 then '上午'
when hour(order_time) >= 11 and hour(order_time) < 13 then '中午'
when hour(order_time) >= 13 and hour(order_time) < 17 then '下午'
when hour(order_time) >= 17 and hour(order_time) < 19 then '晚上'
when hour(order_time) >= 19 and hour(order_time) < 20 then '半夜'
when hour(order_time) >= 20 and hour(order_time) < 24 then '深夜'
when hour(order_time) >= 0 and hour(order_time) < 1 then '凌晨'
end as order_time_range,
date_format(order_time, 'yyyy-MM-dd HH:mm') as order_time
from
ods_didi.t_user_order
where
dt = '2020-04-12' and
length(order_time) >= 8;
2 订单分析
2.1 app层建表
数据分析好了,但要知道,我们处理大规模数据,每次处理都需要占用较长时间,所以,我们可以 将计算好的数据,直接保存下来。将来,我们就可以快速查询数据结果了。所以,我们可以提前在 app层创建好表。
sql
-- 创建保存日期对应订单笔数的app表
create table if not exists app_didi.t_order_total(
date string comment '日期(年月日)',
count integer comment '订单笔数'
)
partitioned by (month string comment '年月,yyyy-MM')
row format delimited fields terminated by ',';
2.2 加载数据到app表
sql
insert overwrite table app_didi.t_order_total partition(month='2020-04')
select
'2020-04-12',
count(orderid) as total_cnt
from
dw_didi.t_user_order_wide
where
dt = '2020-04-12';
2.3 不同地域订单占比分析(省份)
sql
create table if not exists app_didi.t_order_province_total(
date string comment '日期',
province string comment '省份',
count integer comment '订单数量'
)
partitioned by (month string comment '年月,yyyy-MM')
row format delimited fields terminated by ',';
insert overwrite table app_didi.t_order_province_total partition(month = '2020-04')
select
'2020-04-12',
province,
count(*) as order_cnt
from
dw_didi.t_user_order_wide
where
dt = '2020-04-12'
group by province;
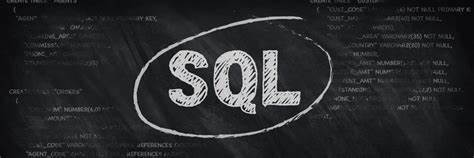
如果您觉得本文还不错,对你有帮助,那么不妨可以关注一下我的数字化建设实践之路专栏,这里的内容会更精彩。
专栏 原价99,现在活动价59.9,按照阶梯式增长,还差5个人上升到69.9,最终恢复到原价。
专栏优势:
(1)一次收费持续更新。
(2)实战中总结的SQL技巧,帮助SQLBOY 在SQL语言上有质的飞越,无论你应对业务难题及面试都会游刃有余【全网唯一讲SQL实战技巧,方法独特】
SQL很简单,可你却写不好?每天一点点,收获不止一点点-CSDN博客
(3)实战中数仓建模技巧总结,让你认识不一样的数仓。【数据建模+业务建模,不一样的认知体系】(如果只懂数据建模而不懂业务建模,数仓体系认知是不全面的)
(4)数字化建设当中遇到难题解决思路及问题思考。
我的专栏具体链接如下: