线性回归是监督学习中的一种基础算法,用于解决回归问题。它通过拟合一条直线(或平面、高维超平面),来预测输出与输入变量之间的关系。
1. 线性回归的基本概念
目标
给定输入 和对应的输出 y,找到一个线性函数:
其中:
是权重(回归系数)。
- b 是偏置(截距)。
- y 是预测值。
损失函数
为了找到最佳的 w 和 b,需要最小化预测值 和真实值
的误差,常用的误差度量是 均方误差(MSE):
其中 m 是样本数。
通过优化该损失函数,可以得到最优的参数 w 和 b。
2. 线性回归的假设
- 线性关系:特征和目标变量之间具有线性关系。
- 独立性:数据样本是相互独立的。
- 同方差性:误差的方差相同,不随输入变化。
- 正态分布:误差服从正态分布。
如果这些假设不能成立,模型的性能可能会下降。
3. 线性回归的实现方式
线性回归可以通过以下两种方式实现:
3.1 正规方程法
通过解析法直接求解参数:
- 优点:无需选择学习率,计算直接得出结果。
- 缺点:当特征维度很高时,计算
的代价很高。
3.2 梯度下降法
通过迭代优化逐步减小损失函数值:
其中 α\alphaα 是学习率。
- 优点:适用于大规模数据。
- 缺点:需要选择合适的学习率,迭代次数较多。
4. Python 实现
4.1 数据生成
python
import numpy as np
import matplotlib.pyplot as plt
# 生成模拟数据
np.random.seed(0)
X = 2 * np.random.rand(100, 1)
y = 4 + 3 * X + np.random.randn(100, 1)
plt.scatter(X, y)
plt.xlabel("x")
plt.ylabel("y")
plt.title("Generated Data")
plt.show()
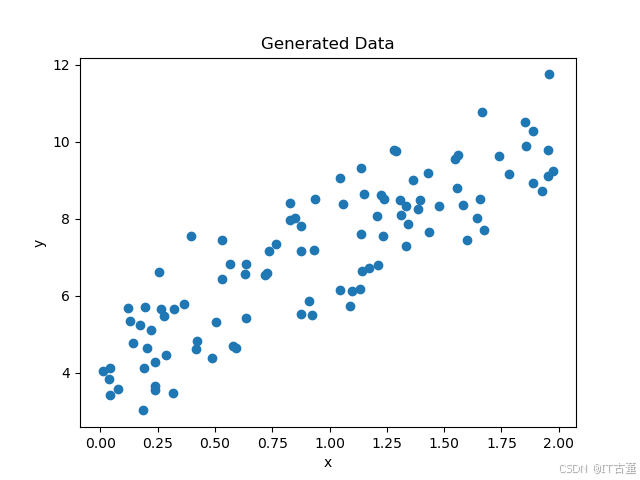
4.2 使用 Scikit-learn 实现
python
import numpy as np
from sklearn.linear_model import LinearRegression
from sklearn.model_selection import train_test_split
from sklearn.metrics import mean_squared_error
# 生成模拟数据
np.random.seed(0)
X = 2 * np.random.rand(100, 1)
y = 4 + 3 * X + np.random.randn(100, 1)
# 数据集划分
X_train, X_test, y_train, y_test = train_test_split(X, y, test_size=0.2, random_state=42)
# 线性回归模型
model = LinearRegression()
model.fit(X_train, y_train)
# 模型预测
y_pred = model.predict(X_test)
# 评估模型
mse = mean_squared_error(y_test, y_pred)
print(f"Mean Squared Error: {mse}")
print(f"Intercept: {model.intercept_}")
print(f"Coefficients: {model.coef_}")
输出结果
bash
Mean Squared Error: 0.9177532469714291
Intercept: [4.20634019]
Coefficients: [[2.9902591]]
4.3 自定义实现(梯度下降法)
bash
import numpy as np
# 生成模拟数据
np.random.seed(0)
X = 2 * np.random.rand(100, 1)
y = 4 + 3 * X + np.random.randn(100, 1)
# 初始化参数
m = len(X)
theta = np.random.randn(2, 1) # [w, b]
X_b = np.c_[np.ones((m, 1)), X] # 添加偏置列
# 超参数
learning_rate = 0.1
n_iterations = 1000
# 梯度下降
for iteration in range(n_iterations):
gradients = 2/m * X_b.T.dot(X_b.dot(theta) - y)
theta -= learning_rate * gradients
print(f"Optimized Theta: {theta.ravel()}")
输出结果
bash
Optimized Theta: [4.22215108 2.96846751]
5. 线性回归的优缺点
优点
- 简单易用,计算成本低。
- 结果具有较高的可解释性。
- 对小数据集表现良好。
缺点
- 假设特征和目标变量之间具有线性关系,适用性有限。
- 对异常值敏感。
- 无法很好地处理复杂的非线性关系。
6. 应用场景
- 预测分析:如房价预测、销售额预测。
- 统计建模:解释变量与目标之间的关系。
- 简单基线模型:为复杂模型提供参考基准。
7. 线性回归的扩展
- 岭回归(Ridge Regression):引入 L2 正则化,缓解过拟合问题。
- Lasso 回归:引入 L1 正则化,实现特征选择。
- 多项式回归:扩展到非线性关系,通过增加多项式特征拟合更复杂的模型。